Does the world need more data scientists?
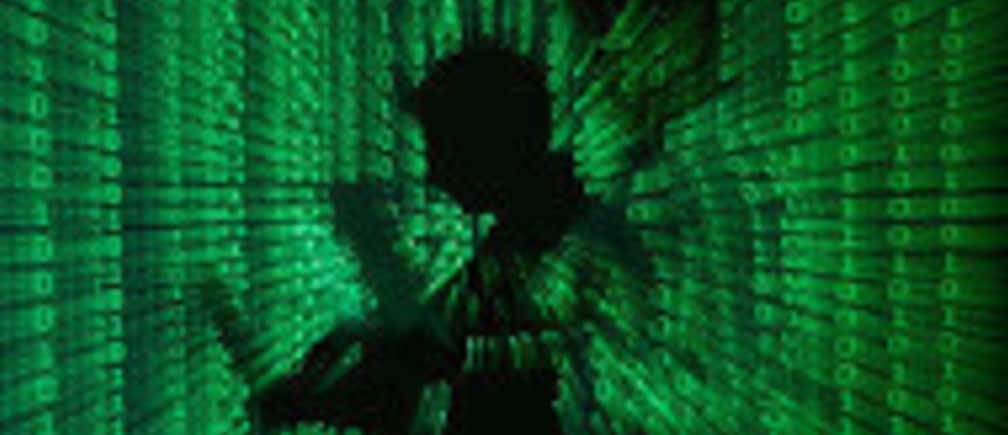
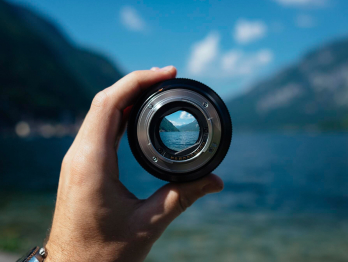
Get involved with our crowdsourced digital platform to deliver impact at scale
Stay up to date:
Digital Communications
The Internet of Things is trumping charges of hype. First mover GE already analyses data streams from 10m sensors in $1trn of managed equipment, while Gartner estimates that 26bn devices will have embedded Internet connectivity within five years. That will be a lot of data.
Going through such vast amounts of information requires a much more skilled workforce — although how large the shortfall and resulting gap are remains uncertain. In 2011, McKinsey Global Institute warned of an upcoming shortage of “140,000 to 190,000 people with deep analytic skills” and “1.5 million managers and analysts”. Gartner estimated in 2012 that only one-third of the 4.4m IT jobs needed globally to support big data would be filled by 2015. This year, the EU said it expected upwards of a 825,000 labour shortfall by 2020.
Part of the difficulty in estimating the size of the gap stems from the fact that data scientists require a quirky combination of statistical, computer, data management and interpretive skills. No one mints them overnight. In 2013, 2,342 doctorates were granted by US universities in biometrics/biostatistics, computer science, statistics and particle physics. Those fields are common sources for recruiting individuals to work in data science—but typically tens of thousands of job are available.
Public and private organisations are already taking steps to create more data scientists. The EU is launching a grand coalition for digital jobs, working with industries and schools to interest young people in technical employment and upgrade the skills of those already working. Singapore is working to build government and industry expertise in data analytics. Last February, the Obama administration appointed D J Patil, a well-known figure in Silicon Valley, as deputy chief technology officer for data policy and chief data scientist.
Governments and companies also need options for today, not just the future. One, advanced by Accenture, is a team approach that divides the many responsibilities of data scientists among a group of employees. A data scientist is “a data engineer, scientist, manager, and teacher, when you add it all together,” says Accenture Institute for High Performance Senior Research Fellow Allan Alter. “You can take that work, break it into components and create a team that can begin to get that work done.”
Crowdsourcing could help, according to Michael Schrage, a research fellow at MIT’s Sloan School of Management. Cloud solutions and broad-bandwidth Internet now enable companies to cost-effectively transmit the large amounts of data necessary for remote analysis, for example.
Automation will also be critical. Mr Schrage expects that within five years half of the complex data analysis tasks could be performed by less-specialised workers with the support of software. “What used to be a top 2% activity is going to be a top quartile activity within five years,” he said. But tools, outsourcing and training won’t guarantee success for all companies. The availability of medical help “doesn’t mean I can’t go to a bad doctor who misdiagnoses me” — people can badly misuse automated tools if they don’t understand the principles.
This article first appeared on GE LookAhead. Publication does not imply endorsement of views by the World Economic Forum.
To keep up with the Agenda subscribe to our weekly newsletter.
Author: Erik Sherman is a contributor at GE LookAhead.
Image: The image shows internet technology. REUTERS
Don't miss any update on this topic
Create a free account and access your personalized content collection with our latest publications and analyses.
License and Republishing
World Economic Forum articles may be republished in accordance with the Creative Commons Attribution-NonCommercial-NoDerivatives 4.0 International Public License, and in accordance with our Terms of Use.
The views expressed in this article are those of the author alone and not the World Economic Forum.
The Agenda Weekly
A weekly update of the most important issues driving the global agenda
You can unsubscribe at any time using the link in our emails. For more details, review our privacy policy.