What India’s cities show us about improving urban mobility
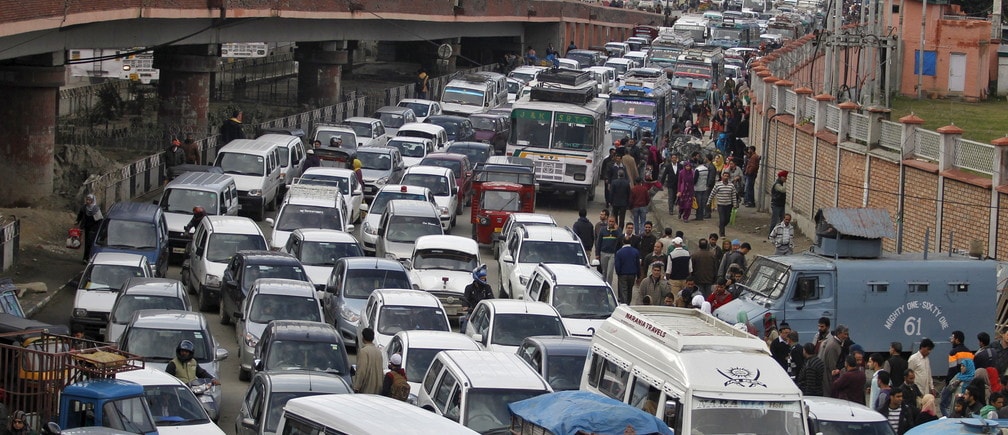
There are large differences in mobility between various Indian cities. Image: REUTERS/Danish Ismail
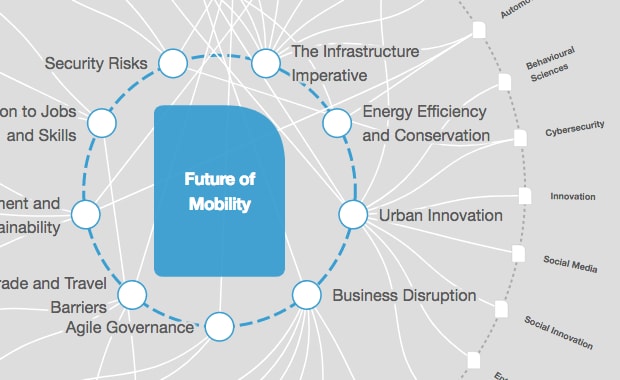
Explore and monitor how Mobility is affecting economies, industries and global issues
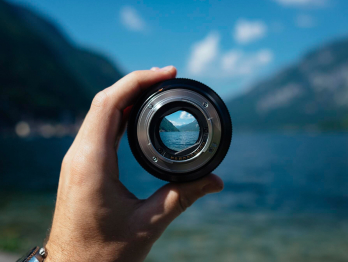
Get involved with our crowdsourced digital platform to deliver impact at scale
Stay up to date:
Mobility
There is an extreme paucity of useful knowledge about urban transportation, especially in developing countries. At the same time, urban transportation in developing countries is prioritised for massive investments. For instance, transportation is the largest sector of lending by the World Bank and represented more than 20% of its net commitments as of 2016.
Existing academic work on the performance of urban transportation systems in the developing world generally focuses on a particular feature of the system in one large city. For instance, Tsivanidis (2018) studies rapid bus transit in Bogota, Hanna et al. (2017) study the high-occupancy-vehicle (HOV) lane in Jakarta, Ang et al. (2018) study speed limits in Sao Paulo, and Kreindler (2018) studies congestion pricing in Bangalore. These innovative studies provide useful policy perspectives, but the lack of city-wide data to compare outcomes across cities prevents a more comprehensive analysis of the determinants of urban mobility. The popular press also regularly features discussions of urban mobility, with a strong focus on congestion as a cause of poor outcomes and often little consideration for other determinants of mobility.
We contribute to this discussion by developing a systematic methodology for measuring the performance of overall motor-vehicle transportation in a city and applying it to study the 154 largest cities in India (Akbar et al. 2018). Using a popular web mapping and transportation service, we generate information for more than 22 million counterfactual trip instances in these cities. We then use our methodology to measure vehicular mobility within cities and decompose it into uncongested mobility and a congestion factor.
Four main lessons arise. First, there are substantial differences in mobility across large Indian cities. The fastest city is nearly twice as fast as the slowest. To illustrate this, Figure 1 plots the speed of travel by motorised vehicles throughout the day in a particularly fast Indian city, Chandigarh, and in a particularly slow city, Kolkata. This is not because Chandigarh is too small to have serious traffic. It has over a million people and typical rush hour speeds there are nearly 10 km/h slower than the fastest overnight speeds.
Figure 1 Speed of travel by motorised vehicles throughout the day in two US cities and two Indian cities
Second, travel is generally slow in Indian cities, even outside peak hours, relative to the US. In addition to Chandigarh and Kolkata, Figure 1 also plots comparable speed data for a fast US city, Grand Rapids, and a slow one, Chicago. Even the central part of Chicago, one of the most congested locations in the US, is generally faster than one of the fastest Indian cities, Chandigarh.
Third, variation in mobility across cities is driven primarily by uncongested mobility, not by congestion delays. Figure 2 shows there is on average little variation across hours of the day for our sample of 154 cities. Due to slow uncongested mobility, a poor city like Varanasi (Benares) is slower than the average Indian city at all times, even at night in the absence of traffic. We see much greater intra-day differences in mobility in large cities, particularly the largest ones close to their centre, as illustrated by Delhi in the figure.
Figure 2 Speed of travel by motorised vehicles throughout the day in Indian cities
More generally, an index of uncongested mobility explains more than 50% of the variance in overall mobility across cities. A simple welfare analysis also suggests much larger gains from a 10% improvement in uncongested mobility than from implementing optimal congestion pricing in all of urban India, even after accounting for the potential for congestion pricing to reduce the significant level of travel-time unreliability that we document.
These findings challenge the conventional wisdom that traffic congestion is the main reason why some cities are slow and some are fast. To take one prominent example, a recent report by the Boston Consulting Group (2018) claims that Kolkata has the most traffic congestion among the four largest Indian cities, using a methodology that simply assumes that all speed reductions below the posted limit are due to congestion. We find that Kolkata is, in fact, the least congested of the four, but the slowest because of low uncongested mobility. This distinction has important policy implications because uncongested speed cannot be improved by congestion pricing or ridesharing promotion or restriction, or other policies often proposed to combat congestion.
Finally, we find that denser, more populated cities are slower; that there is a hill-shaped relationship between city per-capita income and mobility, with the fastest cities at upper middle-income levels; and that a city’s mobility is related to characteristics of its road network.
This set of results challenges the popular view that urbanisation and economic development lead to ever larger cities and increased rates of motorisation, and eventually to complete gridlock. We do find evidence of congestion in the largest Indian cities and a strong association between congestion and household access to motorised vehicles. However, economic development also brings about better travel infrastructure which facilitates uncongested mobility.
In fact, indicators of urban economic development such as faster recent population growth, higher income levels (except at the very top), and higher motorisation rates are generally associated with better overall mobility despite worse congestion. For instance, we find suggestive evidence that better mobility is associated with a more regular grid network and more primary roads.
The kind of data we use can also be used to learn about the fundamentals of urban travel beyond mobility and congestion. For example, they can improve our understanding of land use and property price patterns in cities in relation to transportation. While these kind of data are less rich in detail than traditional travel surveys, they can be gathered at a fraction of the cost – hundreds of dollars instead of tens of millions for a full travel survey. Given the low cost of collecting such data, large samples can easily be targeted to small areas and narrow issues.
This data can also be collected at a much higher frequency than the typical five- to eight-year gap between consecutive traditional travel surveys, thus allowing policy changes to be evaluated over the short run (Kreindler 2016, Hanna et al. 2017, Ang et al. 2018). We believe future studies of this type will shed useful light on many aspects of transportation policy in cities. Many other possible applications are possible, including, for instance, the monitoring of city recovery after major natural disasters.
We therefore hope that more data underlying the production of real-time travel information will be made available for research. The data that we use allow us to learn about mobility and the price (time cost) of travel for all possible trips at all times. The analogous quantities (i.e. number of travellers) are potentially knowable from the same underlying data. With both prices and quantities, the detailed study of congestion – both on particular road segments and in larger areas – will be possible.
Repeated observations of the same travellers would also enable a much better analysis of individual travel behaviour. For instance, Kreindler (2018) uses a panel of trip-level data for 2,000 commuters from a smartphone app to learn about individual response to peak-travel congestion and to measure the welfare impact of various pricing policies to alleviate congestion in Bangalore. With appropriate regard for privacy, the availability of larger trip-level samples across cities would allow for a comprehensive analysis of the welfare consequences of better urban mobility and accessibility.
Don't miss any update on this topic
Create a free account and access your personalized content collection with our latest publications and analyses.
License and Republishing
World Economic Forum articles may be republished in accordance with the Creative Commons Attribution-NonCommercial-NoDerivatives 4.0 International Public License, and in accordance with our Terms of Use.
The views expressed in this article are those of the author alone and not the World Economic Forum.
Related topics:
The Agenda Weekly
A weekly update of the most important issues driving the global agenda
You can unsubscribe at any time using the link in our emails. For more details, review our privacy policy.
More on Geographies in DepthSee all
Sha Song
May 8, 2024
Phil Baty
May 8, 2024
Pooja Chhabria
April 29, 2024
Naoko Tochibayashi and Naoko Kutty
April 25, 2024
Andrea Willige
April 23, 2024
Libby George
April 19, 2024