AI technology is revolutionizing Australia's cotton farming industry
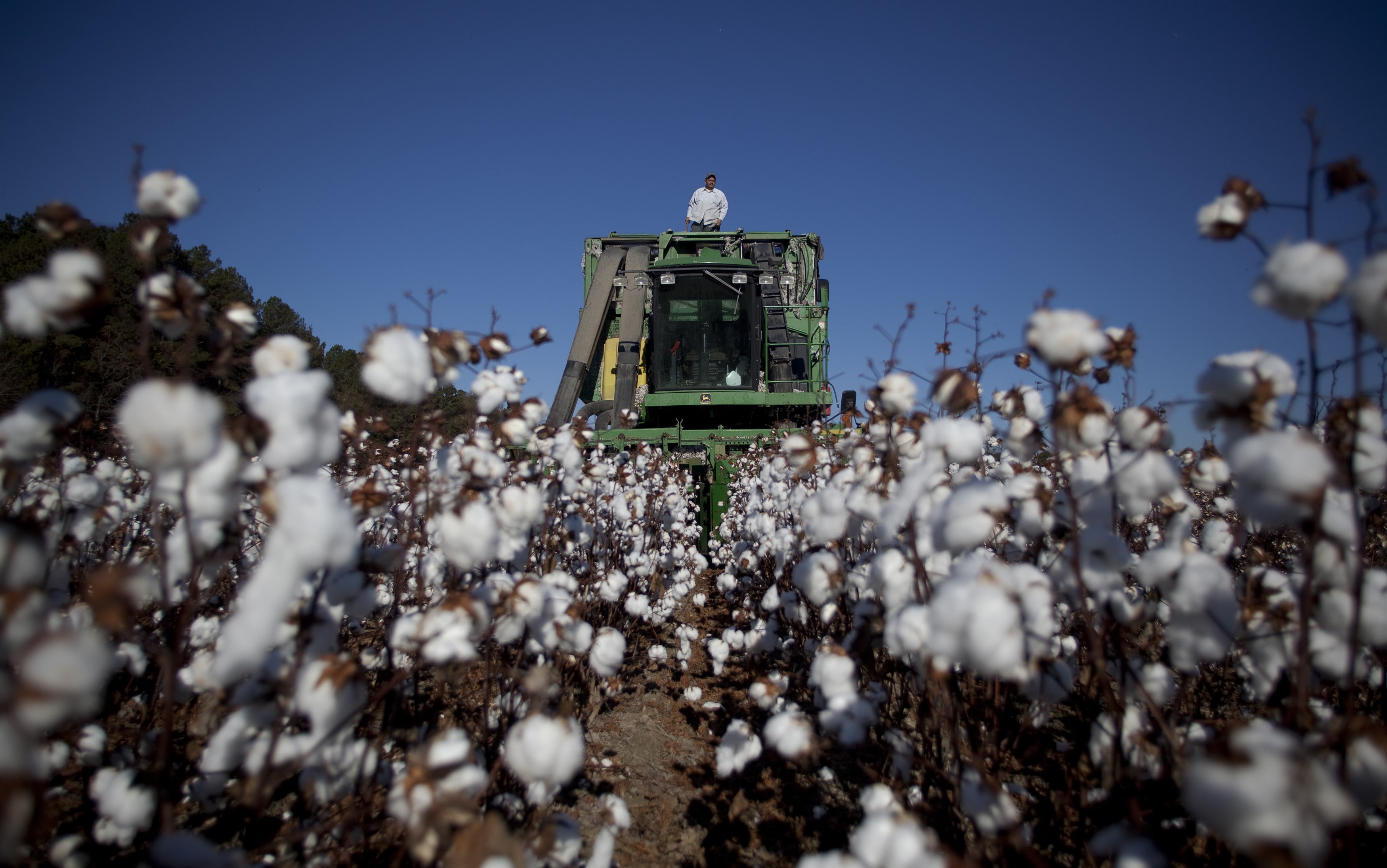
Cotton is one of the world's leading value-added crops. Image: REUTERS/Randall Hill.
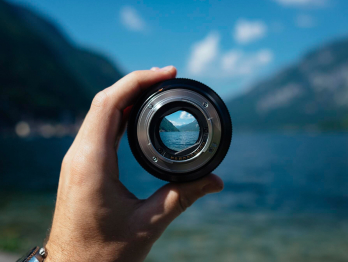
Get involved with our crowdsourced digital platform to deliver impact at scale
Stay up to date:
Emerging Technologies
- Cotton production in Australia is part of a billion dollar agricultural industry.
- Spray drift – pesticides moving beyond targeted areas – presents a risk to the industry.
- A new project using AI technology is enabling farmers to control spraying operations through data-driven decision making.
In 2018-2019, the Australian agricultural industry was worth $62 billion AUD. As the population continues to grow and food security is challenged, technological innovations are vital to keep this sector thriving.
Farms across the world are relying on automated machinery and analytics to monitor soil and crop levels in a bid to improve farming efficiency and ensure the world’s population can access what it needs to survive and thrive. This includes one of the most important and frequently used natural fibres grown across the world – cotton.
Cotton is an essential commodity in the world economy and is one of the world's leading value-added crops. The production of cotton on the land and the processing of cotton in factories, leads to billions of dollars worth of global business activities and revenue.
Finding ways to apply data-driven AI technology to ensure the viability of this industry is of worldwide importance.
What is spray drift and why is it an issue?
One of the greatest risks to the cotton industry – and many other agriculture industries – is spray drift.
Pesticides are used in many farming practices to protect crops against pests, weeds and diseases, and help ensure the Australian agricultural industry remains productive, profitable and sustainable.
However, the movement of pesticides beyond the target crop – spray drift – is extremely undesirable as it results in wastage and unwanted exposure to non-target areas.
Spray drift and the unwanted movement of pesticides, has a detrimental financial impact on growers and is a global environmental issue that needs to be addressed.
”Cotton is particularly sensitive to spray drift from pesticides, and in 2018 the financial impact on the Australian cotton industry was significant – costing an estimated $18 million AUD in production losses.
Spray drift and the unwanted movement of pesticides, has a detrimental financial impact on growers and is a global environmental issue that needs to be addressed.
While the problem of spray drift is not new, it has been a significant problem in the agriculture industry for some time. Robots and machine learning technologies, which form part of the broader field of AI, have the capability to facilitate new, more sustainable agricultural methods that will take farming practices to new heights by conserving resources, minimising the use of pesticides and shortening the time to market.
AI is improving productivity and reducing environmental impact
A recent partnership between the Faculty of Information Technology at Monash University, BARD AI, PentaQuest, and AgriSci, is applying AI technology to support informed spraying operations and better decision making, thereby reducing the impacts of spray drift and improving overall agricultural productivity, profitability and sustainability, while minimising the overall environmental impact.
The Revolutionising Agricultural Spray Applications for the Cotton Research and Development Corporation (CRDC) project is applying AI and game-design practices to tackle the challenge of spray drift.
Information alone does not change behaviour and the use of advanced technology doesn’t ensure the adoption of new platforms by farmers. By incorporating game-like design applications which drive better training and engagement outcomes, together with AI-driven decision support modelling, the project is able to deliver continuous adoption and accurate decision support that informs farmers appropriately.
The gamification mechanics of the platform encourage behavioural change by incentivising farmers through community participation, recognition of compliance as well as real-time risk assessments regarding their current spraying practices.
The AI component of the project will rely on Bayesian Networks, which provide a rigorous probabilistic methodology for modelling real-world problems that involve uncertainty. This technology will deliver decision support by informing farmers in real-time about the potential risks and consequences of their spraying decisions.
Investment is needed to scale-up innovations
In order to further the application of AI in the agriculture sector, it’s essential for governments at all levels to invest in infrastructure and programmes to support the adoption, trial and development of farm management solutions.
The project received a Business Research and Innovation Initiative grant of close to $100,000 AUD earlier this year and is also in consideration to receive a further $1 million AUD to develop a prototype or proof of concept, which will be determined later this year.
Funding opportunities such as this have ensured the project team was able to work with local groups of like-minded growers, agronomists, resellers, consultants and spray contractors to help eradicate spray drift.
It’s hoped that through further funding, the project will be able to engage the agricultural community and encourage practice change that reduces the occurrence of off-target spraying and safer spraying techniques.
With the scaling and diversification of agricultural activity across the globe, the issue of spray drift is becoming more crucial. With pesticides being used in greater numbers, the remaining un-farmed land and natural surrounding wildlife need to be protected more than ever before.
As global population growth leads to urban sprawl, less land will become available for agricultural practices, forcing farmers to be more efficient with how they use their land. Robotics, machine learning and new digital agricultural practices can play a part in future-proofing this sector.
Finding ways to reduce the volume of pesticides used in farming practices, while maintaining efficacy and being conscious of protecting the surrounding environment is of paramount importance.
Don't miss any update on this topic
Create a free account and access your personalized content collection with our latest publications and analyses.
License and Republishing
World Economic Forum articles may be republished in accordance with the Creative Commons Attribution-NonCommercial-NoDerivatives 4.0 International Public License, and in accordance with our Terms of Use.
The views expressed in this article are those of the author alone and not the World Economic Forum.
Related topics:
The Agenda Weekly
A weekly update of the most important issues driving the global agenda
You can unsubscribe at any time using the link in our emails. For more details, review our privacy policy.
More on Industries in DepthSee all
Robin Pomeroy
April 25, 2024
Daniel Boero Vargas and Mandy Chan
April 25, 2024
Abhay Pareek and Drishti Kumar
April 23, 2024
Charlotte Edmond
April 11, 2024
Victoria Masterson
April 5, 2024
Douglas Broom
April 3, 2024