AI’s trolley problem can lead us to surprising conclusions
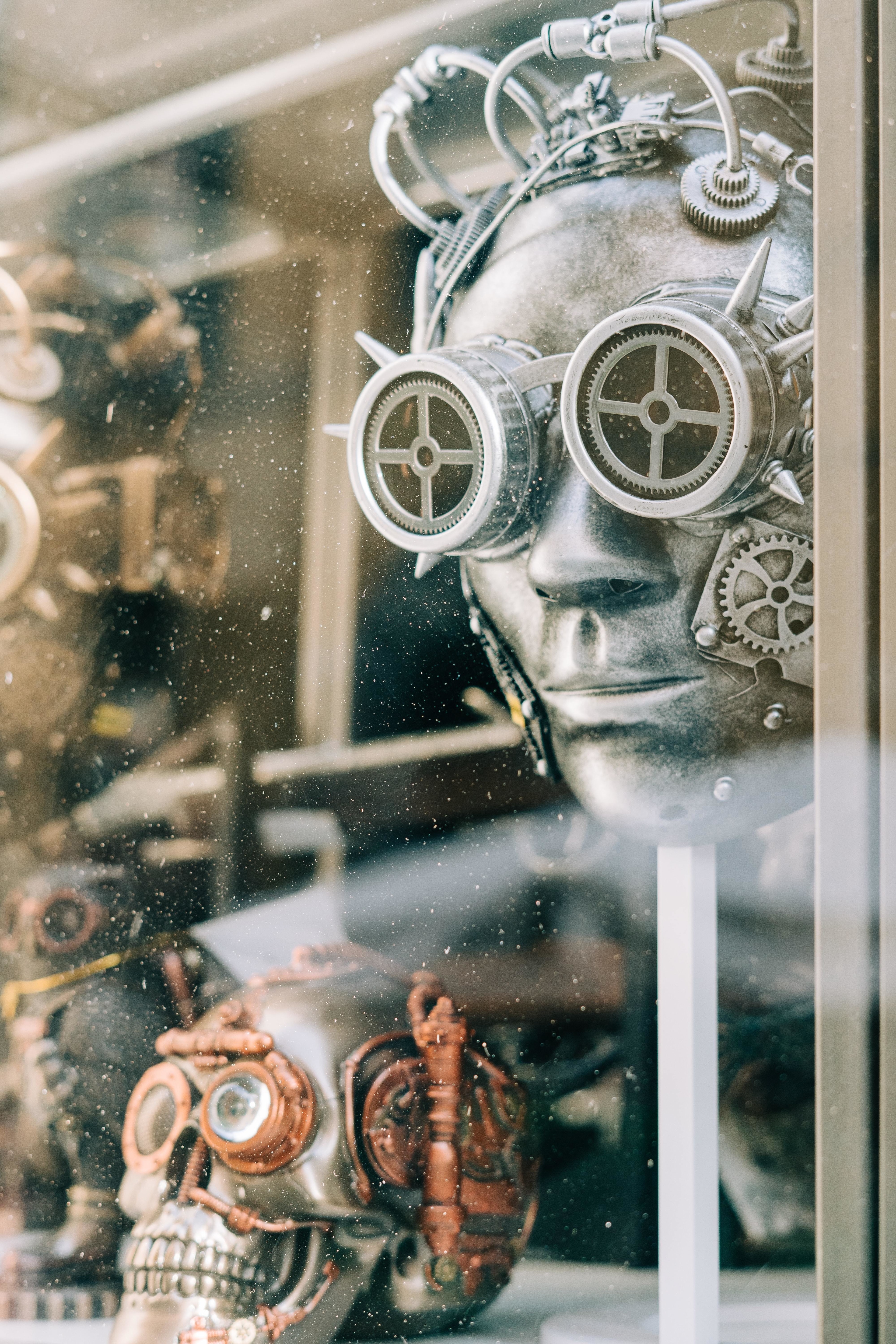
Responsibly deployed robotics and machine learning can lead to more lives saved than lost. Image: Unsplash/Gabriella Clare Marino
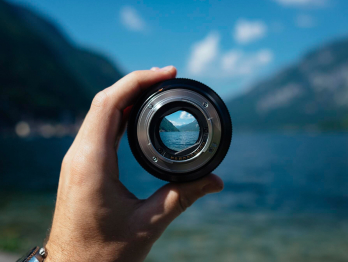
Get involved with our crowdsourced digital platform to deliver impact at scale
Stay up to date:
Automotive and New Mobility
Listen to the article
- Autonomous machines and decision-making can lead to potentially fatal errors.
- Deaths occurring due to robotic errors produce moral dilemmas, much like "the trolley problem."
- There is a case that many lives could be saved if society embraces machine learning and commits itself to deploying robotics technologies responsibly.
Advances in robotics mean autonomous vehicles, industrial robots and medical robots will be more capable, independent and pervasive over the next 20 years. Eventually, these autonomous machines could make decision-making errors that lead to hundreds of thousands of deaths, which could be avoided if humans were in the loop.
Such a future is reasonably frightening but more lives would be saved than lost if society adopts robotic technologies responsibly.
The machine learning process
Robots aren’t “programmed” by humans to mimic human decision-making; they learn from large datasets to perform tasks like “recognize a red traffic light” using complex mathematical formulas induced from data. This machine learning process requires much more data than humans need. However, once trained, robots would outperform humans in any given task and AI and robotics have dramatically improved their performance over the past five years through machine learning.
We can take autonomous driving as an example, although the following theories apply to healthcare, manufacturing and other rapidly automating sectors. A seasoned human driver may have a few hundred thousand miles of driving experience over their lifetime but Waymo – the self-driving car company from Google – completed over 2.3 million driven miles in 2021 alone. Its AI technologies learn from every car it deploys at once; these cars never tire and never forget lessons.
When Tesla first rolled out its “smart summon” feature allowing its cars to leave a parking space and navigate around obstacles without their owner, many users complained about the poor performance. But within weeks, Tesla collected data from the early users and retrained their machine learning models. As a result, the smart summon feature’s performance improved dramatically and has become a key differentiator for Tesla.
Autonomous robotic lifesavers
With more and more data to learn from, AI is improving quickly, becoming more accurate, adaptive and safe. As the number of robots grows into mainstream daily use, so will their applications, signalling a stepwise rollout strategy for functional robotics. Autonomous driving will go from “hands-on” to “hands-off” to “eyes off” to “mind off” and eventually to “no steering wheel.”
A good example is China’s WeRide. The autonomous vehicle company has deployed robo-buses and street cleaners in several cities in China. They currently operate in more constrained environments than robo-taxis, providing substantially improved safety compared to human operators. Yet these constrained vehicles gather a tremendous amount of data, eventually freeing them of such limitations.
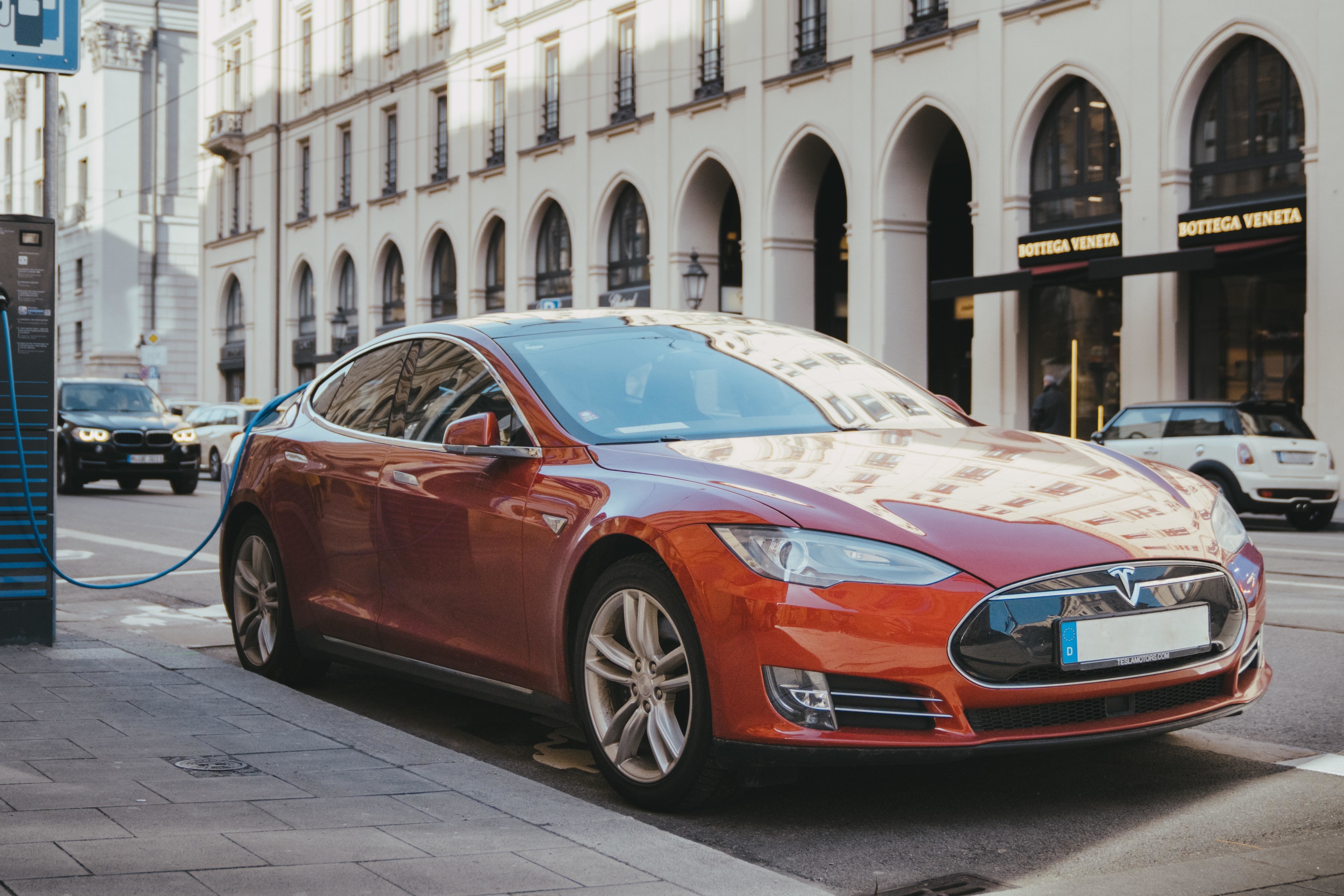
As robots move from simple to complex, more data will be collected to improve performance and safety. For example, by reducing human error (the most common cause of road accidents), autonomous vehicles could prevent 47,000 serious accidents and save 3,900 lives in the UK alone over the next decade. RAND Corporation found that AVs will save lives even when only 10% safer than humans.
A moral dilemma
There are still major concerns around mass robotic rollout, including the moral objection to any human life being lost at all due to machine error. The trolley problem – the ethical dilemma where an onlooker can save five lives from a rogue trolley by diverting it to kill just one person – illustrates why making decisions about who lives and dies are inherently moral judgments so they can’t be relegated to unfeeling machines.
This “moral dilemma” is exacerbated because robots’ and humans’ perceptions differ, resulting in different types of mistakes. For example, robots have lightning reflexes with unflinching attention but can misidentify hazards, such as when an Uber self-driving car took a pedestrian dragging a bicycle across the road to be a car, expecting it to travel faster than it was.
The disparity between human and machine errors makes public acceptance of deaths from robots harder, especially if each one is met by the same media reaction as the 2018 fatality in Phoenix received. If the media disproportionately condemns every robot-induced death with damning headlines it has the potential to destroy confidence in autonomous systems, despite the technology’s potential to save millions of lives.
When human drivers cause fatalities, they face judgment and consequences under the law. But the “black box of AI” can’t explain its decision-making in humanly comprehensible or legally and morally justifiable terms to a judge and the public.
Another issue is accountability. In the Phoenix case, the human backup driver was charged with negligible homicide. But is there a case for the car manufacturer, AI algorithm provider or engineer who wrote the algorithm to be responsible and liable? Only when accountability is clear can an ecosystem be built around it.
The trolly problem in the age of AI and machine learning
Because many lives could be saved, there’s an argument for launching robotic technologies once proven to be slightly better than people. Thus, every opportunity should be taken to launch robotic tools that assist humans before robots are given more autonomy. Then, their rollout should be within constrained environments before being available more widely. Doing so, in this way, will allow more data to be gathered, improving the robotic performance and minimizing the number of lives lost.
Given the likely objections, we need to work on communicating the short-term pain collectively and the long-term gain involved. Doing so will enable us to work towards a responsible and thoughtful rollout of robotics so that this adoption process brings greater good to humankind.
Don't miss any update on this topic
Create a free account and access your personalized content collection with our latest publications and analyses.
License and Republishing
World Economic Forum articles may be republished in accordance with the Creative Commons Attribution-NonCommercial-NoDerivatives 4.0 International Public License, and in accordance with our Terms of Use.
The views expressed in this article are those of the author alone and not the World Economic Forum.
Related topics:
The Agenda Weekly
A weekly update of the most important issues driving the global agenda
You can unsubscribe at any time using the link in our emails. For more details, review our privacy policy.
More on Forum InstitutionalSee all
Gayle Markovitz
April 28, 2024
Gayle Markovitz
April 27, 2024
Mirek Dušek and Maroun Kairouz
April 27, 2024
Kate Whiting
April 26, 2024
Spencer Feingold and Gayle Markovitz
April 19, 2024
Kate Whiting
April 17, 2024