This AI tool helps identifies breast cancer with 90% accuracy rate
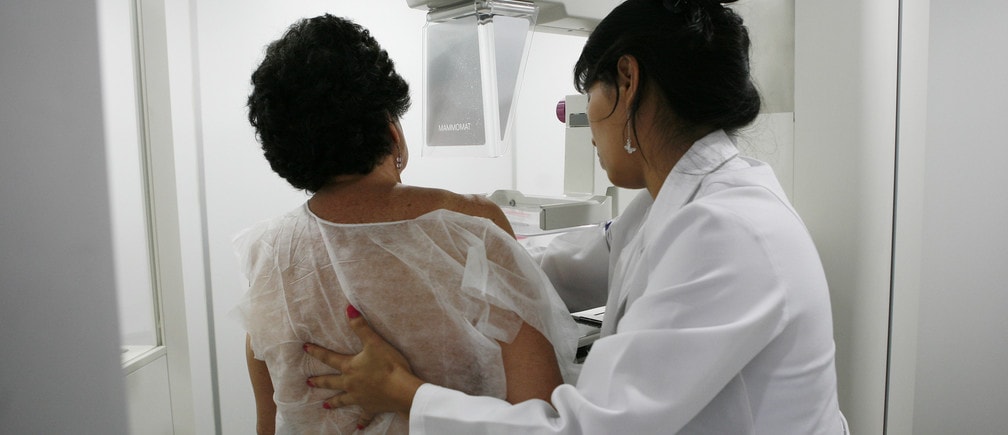
A woman undergoes breast cancer screening. Image: REUTERS/Enrique Castro-Mendivil
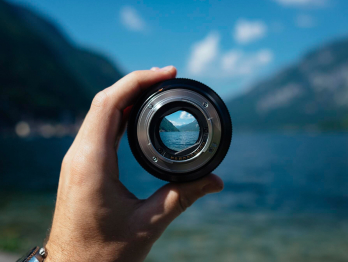
Get involved with our crowdsourced digital platform to deliver impact at scale
Stay up to date:
Global Health
An artificial intelligence tool—trained on roughly a million screening mammography images—can identify breast cancer with approximately 90% accuracy when combined with radiologist analysis, a new study finds.
The study examined the ability of a type of artificial intelligence (AI), a machine learning computer program, to add value to the diagnoses a group of 14 radiologists reached as they reviewed 720 mammogram images.
“Our study found that AI identified cancer-related patterns in the data that radiologists could not, and vice versa,” says senior study author Krzysztof Geras, assistant professor in the radiology department at New York University’s Grossman School of Medicine.
“AI detected pixel-level changes in tissue invisible to the human eye, while humans used forms of reasoning not available to AI,” adds Geras, also an affiliated faculty member at the Center for Data Science. “The ultimate goal of our work is to augment, not replace, human radiologists.”
In 2014, women (without symptoms) in the United States got more than 39 million mammography exams to screen for breast cancer and determine the need for closer follow-up. Women whose test results yield abnormal mammography findings are referred for biopsy, a procedure that removes a small sample of breast tissue for laboratory testing.
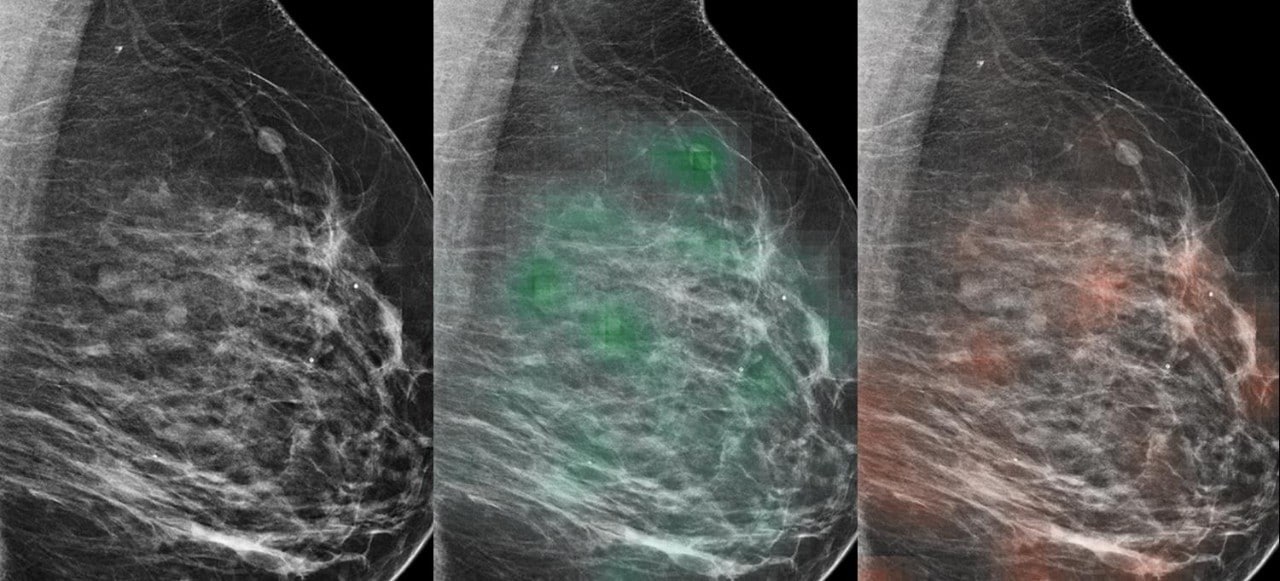
In the new study, the research team designed statistical techniques that let their program “learn” how to get better at a task without being told exactly how. Such programs build mathematical models that enable decision-making based on data examples fed into them, with the program getting “smarter” as it reviews more and more data.
Modern AI approaches, which take inspiration from the human brain, use complex circuits to process information in layers, with each step feeding information into the next, and assigning more or less importance to each piece of information along the way.
The authors of the current study trained their AI tool on many images matched with the results of biopsies performed in the past. Their goal was to enable the tool to help radiologists reduce the number of biopsies needed moving forward. This can only be achieved, says Geras, by increasing the confidence that physicians have in the accuracy of assessments made for screening exams (for example, reducing false-positive and false-negative results).
For the current study, the research team analyzed images collected as part of routine clinical care over seven years, sifting through the collected data and connecting the images with biopsy results. This effort created an extraordinarily large dataset for their AI tool to train on, the authors say, consisting of 229,426 digital screening mammography exams and 1,001,093 images. Most databases the researchers used in studies to date have been limited to 10,000 images or fewer.
Thus, the researchers trained their neural network by programming it to analyze images from the database for which cancer diagnoses had already been determined. This meant that researchers knew the “truth” for each mammography image (cancer or not) as they tested the tool’s accuracy, while the tool had to guess. The researchers measured accuracy in the frequency of correct predictions.
In addition, the researchers designed the study AI model to first consider very small patches of the full resolution image separately to create a heat map, a statistical picture of disease likelihood. Then the program considers the entire breast for structural features linked to cancer, paying closer attention to the areas flagged in the pixel-level heat map.
Rather than have the researchers identify image features for their AI to search for, the tool is discovering on its own which image features increase prediction accuracy. Moving forward, the team plans to further increase this accuracy by training the AI program on more data, perhaps even identifying changes in breast tissue that are not yet cancerous but have the potential to be.
“The transition to AI support in diagnostic radiology should proceed like the adoption of self-driving cars—slowly and carefully, building trust, and improving systems along the way with a focus on safety,” says first author Nan Wu, a doctoral candidate at the Center for Data Science.
The study appears in IEEE Transactions on Medical Imaging.
Additional coauthors are from NYU, SUNY Downstate College of Medicine, the University of Cambridge, and Jagiellonian University.
Support for the work came, in part, from the National Institutes of Health. The model used in this study has been made available to the field to drive innovation.
Don't miss any update on this topic
Create a free account and access your personalized content collection with our latest publications and analyses.
License and Republishing
World Economic Forum articles may be republished in accordance with the Creative Commons Attribution-NonCommercial-NoDerivatives 4.0 International Public License, and in accordance with our Terms of Use.
The views expressed in this article are those of the author alone and not the World Economic Forum.
The Agenda Weekly
A weekly update of the most important issues driving the global agenda
You can unsubscribe at any time using the link in our emails. For more details, review our privacy policy.
More on Fourth Industrial RevolutionSee all
Thomas Beckley and Ross Genovese
April 25, 2024
Agustina Callegari and Daniel Dobrygowski
April 24, 2024
Christian Klein
April 24, 2024
Sebastian Buckup
April 19, 2024
Claude Dyer and Vidhi Bhatia
April 18, 2024