How deep learning can improve productivity and boost business
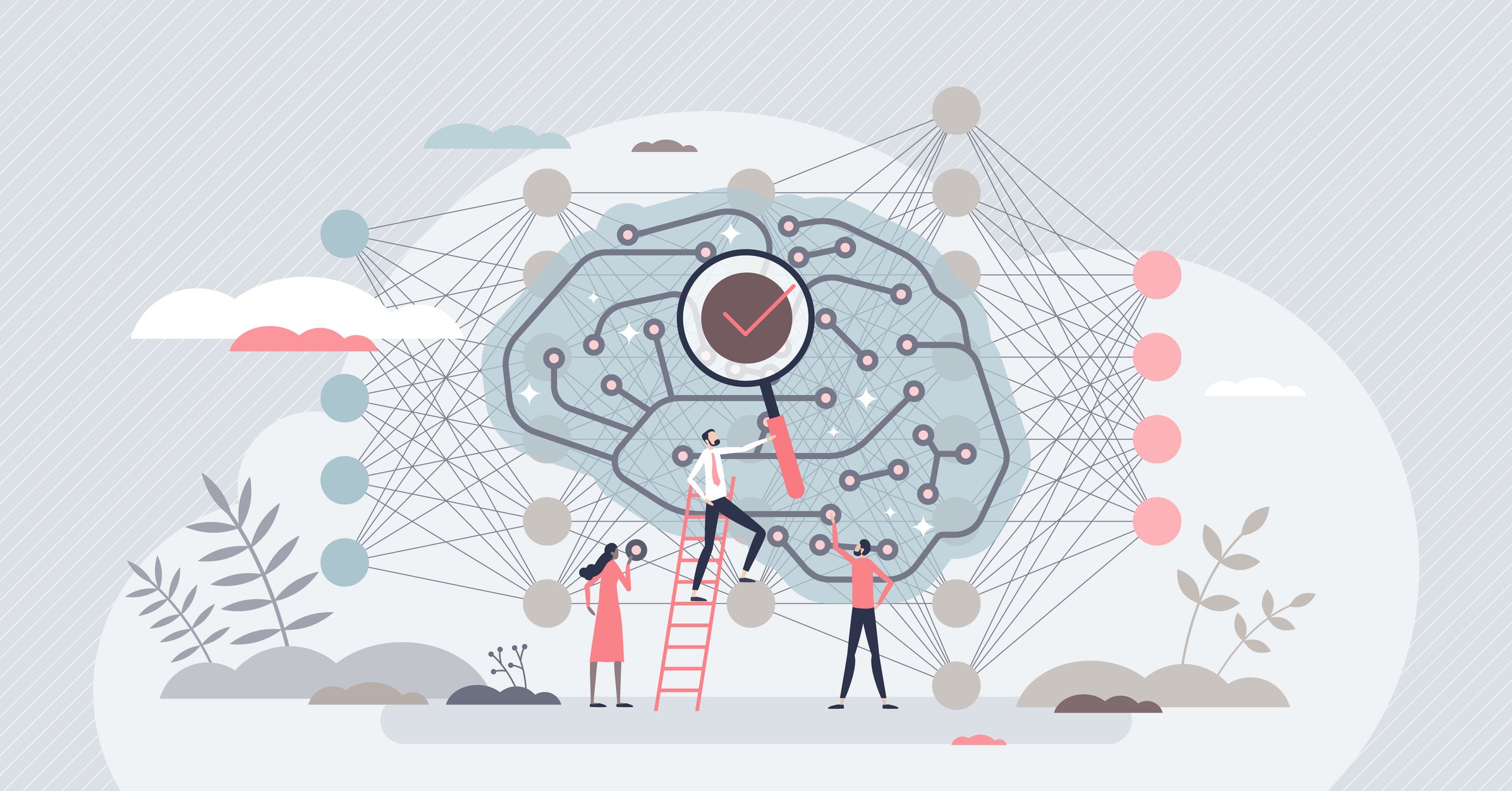
Deep learning neural networks learn from examples, just like human brains do. Image: Getty Images/iStockphoto
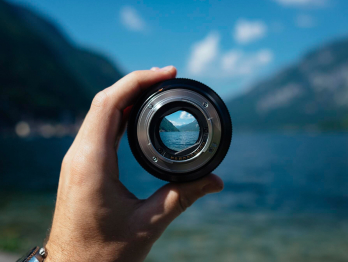
Get involved with our crowdsourced digital platform to deliver impact at scale
Stay up to date:
Innovation
Listen to the article
- Deep learning neural networks mimic the decision-making processes of the human brain by making a series of calculations to reach a conclusion.
- Machines can process massive amounts of data that humans can't, but sound governance structures are needed to ensure positive results.
- Deep learning can improve productivity, increase retention and drive revenue if companies use data to their advantage.
When you “work through” a problem or issue that requires a decision, you likely feel as if you’re going through a linear checklist. But that’s not how the human brain operates; it processes in a non-linear pattern. And this is essentially how deep learning, a subset of artificial intelligence (AI), works too.
Deep learning works like the human brain
Deep learning, at its essence, learns from examples — the way the human brain does. It’s imitating the way humans acquire certain types of knowledge. Because deep learning processes information in a similar manner, it can be used to do things people can do – for example, learning how to drive a car or identifying a dog in a picture.
Deep learning is also used to automate predictive analytics – for example, identifying trends and customer buying patterns so a company can gain more customers and keep more of them. You know those sections on retail sites that show items “frequently bought together” when you’re purchasing a new screwdriver? Those are based on predictive deep learning algorithms that have considered both your current search and past buying patterns to suggest additional products you might also need.
Other applications include numerous everyday encounters and activities, such as virtual assistants, fraud detection, language translation, chatbots and service bots, colourization of black-and-white images, facial recognition and disease diagnoses.
A simple example of a neural network’s application is in parsing speech. The network takes sounds from raw audio, which combine to make syllables, which combine to make words, which combine to make phrases that prompt actions. The machine learns that this particular sound means that it should pull up a credit card balance and the more times it’s asked the same thing, the more accurate it gets.
Deep learning has applications across industries
Neural networks are not new; they’ve been around since the 1940s. In 1943, two computer scientists introduced models of neurological networks, recreated threshold switches based on neurons and showed that even simple networks of this kind are able to calculate nearly any logic or arithmetic function.
The first computer precursors were developed by a computer scientist who was tired of calculating ballistic trajectories by hand. Today, more than 70 years later, deep learning has exploded in sophistication and use, primarily because of expanded computing power (along with greatly reduced costs per unit of power), better modelling and the availability of data. Deep learning requires massive amounts of data. Currently, it’s estimated that the data we generate every day is 2.6 quintillion bytes. And it can analyse massive datasets far faster than a human. Machines don’t suffer from monotony or fatigue.
Are there risks with deep learning?
Let’s answer that question using the example of autonomous vehicles. Deep learning has given us these self-driving cars, but they seem unlikely to eliminate all road accidents, something that would be akin to a self-driving utopia. In fact, a recent study from the Insurance Institute for Highway Safety (IIHS) says that autonomous vehicles might prevent only about a third of all crashes. Still, that's more successful than people.
Yet concerns about widespread adoption may also include an increase in accident rates in the early days of rollout as the technology learns, moral decisions being left to manufacturers, and difficulty in attributing responsibility for accidents. And then there’s hacking, because, after all, deep learning is simply technology encased in a vehicle. In March 2019, two “white hat” hackers (the good guys) needed only a few minutes to go through the browser of the infotainment system to get inside a Tesla’s computer, run their own code, and have the car respond to their commands.
We must also consider the use of deep learning from the consumer’s viewpoint. If it doesn’t “work”—for example, a phone fails to unlock—it can create an unhappy or frustrated customer, which defeats the purpose. Compounding the issue, because of the complexity of the neural networks in deep learning, it can be difficult to know where or why the system went awry. Often described as the black box of deep learning, data scientists are working to improve the visibility and transparency around how deep learning models work.
Models can also have bias unintentionally built-in — and these deep learning models are being used for significant decisions, including who gets loans, jobs or parole. Deep learning needs to have clear guardrails with appropriate governance structures.
Deep learning is the future of business
Deep learning has given us image-based product searches – Pinterest, for example – and efficient ways to sort fruits and vegetables to reduce labour costs. The former is more of a consumer convenience, while the latter is a true business case for productivity.
Significant resources are being put into deep learning in financial services, in which it is used to detect fraud, reduce risk, automate trading and provide “robo-advice” to investors. According to a report from the Economist Intelligence Unit (EIU), 86% of financial services firms plan to increase their AI-related investments by 2025.
How is the World Economic Forum ensuring the responsible use of technology?
Embedding AI across your business has the power to enhance differentiation and competitiveness, increase productivity, influence retention, and even change the course of disease – and it is happening across industries and within all aspects of businesses.
It is influencing everything from the re-creation of business and operating models, to hiring and retention strategies, to creating new corporate cultures that not only embrace but enable the use of deep learning. However, by some estimates, less than 1% of most organizations’ data is being used, even though there are massive amounts of data available to use for transformative decision making. When will you start tapping — and using — yours?
Don't miss any update on this topic
Create a free account and access your personalized content collection with our latest publications and analyses.
License and Republishing
World Economic Forum articles may be republished in accordance with the Creative Commons Attribution-NonCommercial-NoDerivatives 4.0 International Public License, and in accordance with our Terms of Use.
The views expressed in this article are those of the author alone and not the World Economic Forum.
Related topics:
The Agenda Weekly
A weekly update of the most important issues driving the global agenda
You can unsubscribe at any time using the link in our emails. For more details, review our privacy policy.
More on Forum InstitutionalSee all
Vivek Salgaocar
September 19, 2024
Andrea Willige
September 19, 2024
Pam Cheng
September 19, 2024
Sarah Barker and Michael Burger
September 19, 2024
Andres Ginatta and Philippe Isler
September 18, 2024
Beatrice Di Caro
September 18, 2024