Machine learning can help protect urban water. Here's how
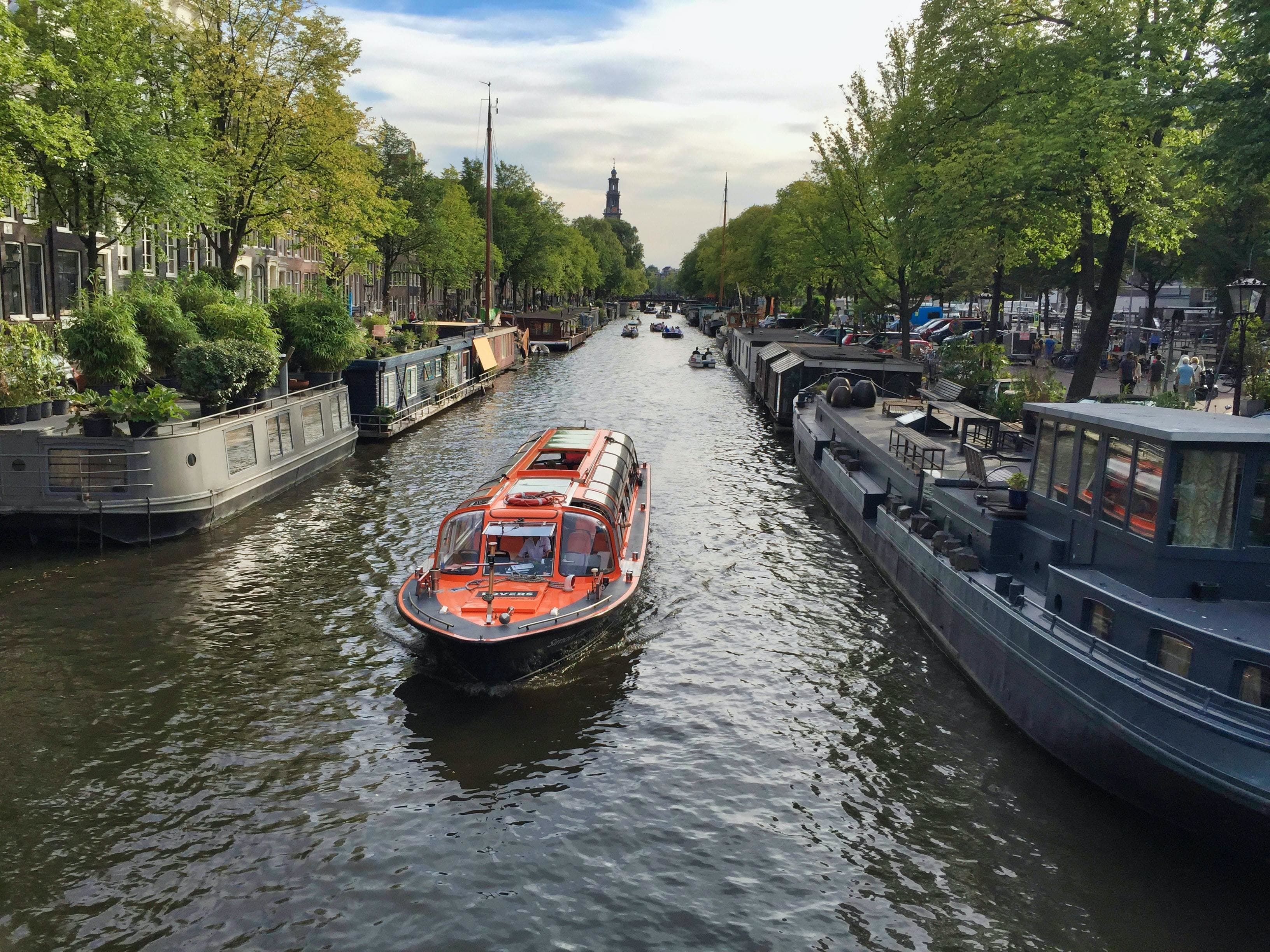
Only 1.2% of freshwater is potable: it is as scarce and valuable as certain rare metals. Image: Unsplash/Secret Travel Guide
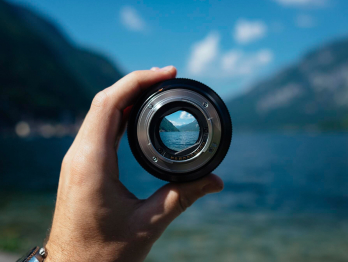
Get involved with our crowdsourced digital platform to deliver impact at scale
Stay up to date:
Water
Listen to the article
- Climate change has highlighted the importance of preserving freshwater as a resource.
- Effective infrastructure management in cities is vital to preserve water.
- Real-Time Sensor Networks with Machine Learning can predict and troubleshoot water leakages more effectively.
Water is perhaps Earth’s most unique natural resource. As the largest natural resource, water covers about 71% of Earth’s surface, but only 1.2% of freshwater is potable. This makes potable water as scarce and valuable as certain rare metals.
Freshwater sourced from rivers and streams can be used for various purposes: commercial, domestic, emergency, and industrial. Given this versatility, and a scarcity is driven by climate change, freshwater has become a point of contention in countries and cities with an expanding urban population. To ensure a steady water supply, good urban governance, and urban resilience, it is imperative for cities to have a robust water infrastructure.
A good water distribution network is key
A critical component of the urban water infrastructure is the water distribution network. An efficient water distribution network bears the capacity to meet the demand for potable water with minimum or no losses. A leading cause of losses in the distribution network, and subsequent demand-supply gap especially in cities, is water leakage.
Water leakage can occur at any stage in the network - transmission, treatment, distribution, or storage. To an extent, the issue of water leakage has been addressed in various ways in cities, especially since decreasing the amount of non-revenue water (water lost in transit) can lead to better socioeconomic, environmental, health and safety outcomes for cities.
Cities generally focus on water leakage source identification and magnitude of leakage through water management systems. Broadly, these management systems focus on quantifying the amount of water lost, detection of leakage hotspots, and effective control of current and future leakage levels.
Despite these measures, according to the World Bank, developing countries lose about 45 million cubic meters of water daily. This water, pumped but lost or unaccounted for, can cost as much as $3 billion USD per year. Being able to save even half these losses can lead to sufficient water supply for at least 90 million people. This is a significant amount, especially for a country like India, which, according to a NITI Aayog report, is expected to witness a two-fold surge in demand for water supply by 2030.
Better water leakage management is urgently needed
There is a dire need to forego the traditional and existing methods of water leakage management for three main reasons.
Firstly, the existing methods are mostly corrective and not predictive. The predictive component in the current water distribution network is predictive maintenance, which is pre-scheduled, conducted manually, and is time-consuming. Even to implement corrective measures for larger water leakages, major sections of the water supply systems are often shut down, adversely affecting the daily lives of citizens especially those who already lack an uninterrupted water supply.
Secondly, while the scale of water leakages in cities vary in magnitude, most corrective measures are taken for larger water leakages while the smaller leakages are often unchecked for a prolonged period. As a result, smaller and unchecked leakages cause water losses of significant magnitude, in volume and revenue. Civic bodies can lose between a hundred thousand to a million dollars in revenue over a five-year period.
Thirdly, in worse instances, a leakage in major water pipelines has also led to short circuits of high-intensity cables thereby posing a lethal threat to the mass population. Such limitations raise a need for more efficient and effective water management systems which are simultaneously corrective and predictive.
A possible solution – real time sensor networks with Machine Learning
A widely recognized solution to check and control water leakage in cities lies in operating Real-Time Sensor Networks with Machine Learning (ML). Real-Time Sensor Networks mainly comprise components such as sensors, network monitoring, cloud storage, and supporting applications.
This Real-Time Sensor Network that monitors waterflow in real time when interfaced with Machine Learning models will be able to predict an anomaly (water leakage) and can accurately detect the same in the water distribution network. This system can have multiple benefits for the city.
Firstly, cities can expect a more accurate prediction of the scale and complexity of water leakages. This is beneficial for cities that have an ageing water distribution system which is more prone to water leakages. The accurate prediction of scale and complexity can lead to correct and timely identification of the location of water leakage. This, in turn, will lower water losses and increase revenue for the city.
Secondly, accurate and timely location of leakage accelerates the pace and effectiveness of troubleshooting. A shorter turnaround time in resolving water leakages is critical when entire sections of the water distribution network are often shut down in search of the point of leakage. Further, this ensures reduction in collateral costs that stem from activities such as digging/reconstruction of roads to locate underground pipe leakages.
Thirdly, the subsequent efficiency in addressing water leakages will reduce downtime of water supply systems thereby improving the reliability of water supply and quality of water. This is of high priority for cities that experience an acute water crisis during summers, peak hours, and that have a high dependence on water for economic activities.
Lastly, this system of Real Time Sensor Networks and ML is relatively inexpensive and straightforward to implement. This system can also assist local, city government, and citizens in anticipating water supply curtailment conditions and help in adopting water conservation measures. Adoption of such systems can contribute to the desired urban transformation.
The socioeconomic, environmental, health, and safety benefits that arise from using a real time sensor network with ML are obvious. Besides this, with rapid urbanization, rising water prices, and drying water sources, the deployment of advanced technological solutions such as ML is time-critical if cities are to avert exacerbated urban water stress.
Don't miss any update on this topic
Create a free account and access your personalized content collection with our latest publications and analyses.
License and Republishing
World Economic Forum articles may be republished in accordance with the Creative Commons Attribution-NonCommercial-NoDerivatives 4.0 International Public License, and in accordance with our Terms of Use.
The views expressed in this article are those of the author alone and not the World Economic Forum.
Related topics:
The Agenda Weekly
A weekly update of the most important issues driving the global agenda
You can unsubscribe at any time using the link in our emails. For more details, review our privacy policy.
More on Climate ActionSee all
Santiago Gowland
April 24, 2024
Amanda Young and Ginelle Greene-Dewasmes
April 23, 2024
Andrea Willige
April 23, 2024
Agustin Rosello, Anali Bustos, Fernando Morales de Rueda, Jennifer Hong and Paula Sarigumba
April 23, 2024
Carlos Correa
April 22, 2024
Shyam Bishen and Annika Green
April 22, 2024