6 ways to help the manufacturing sector embrace AI
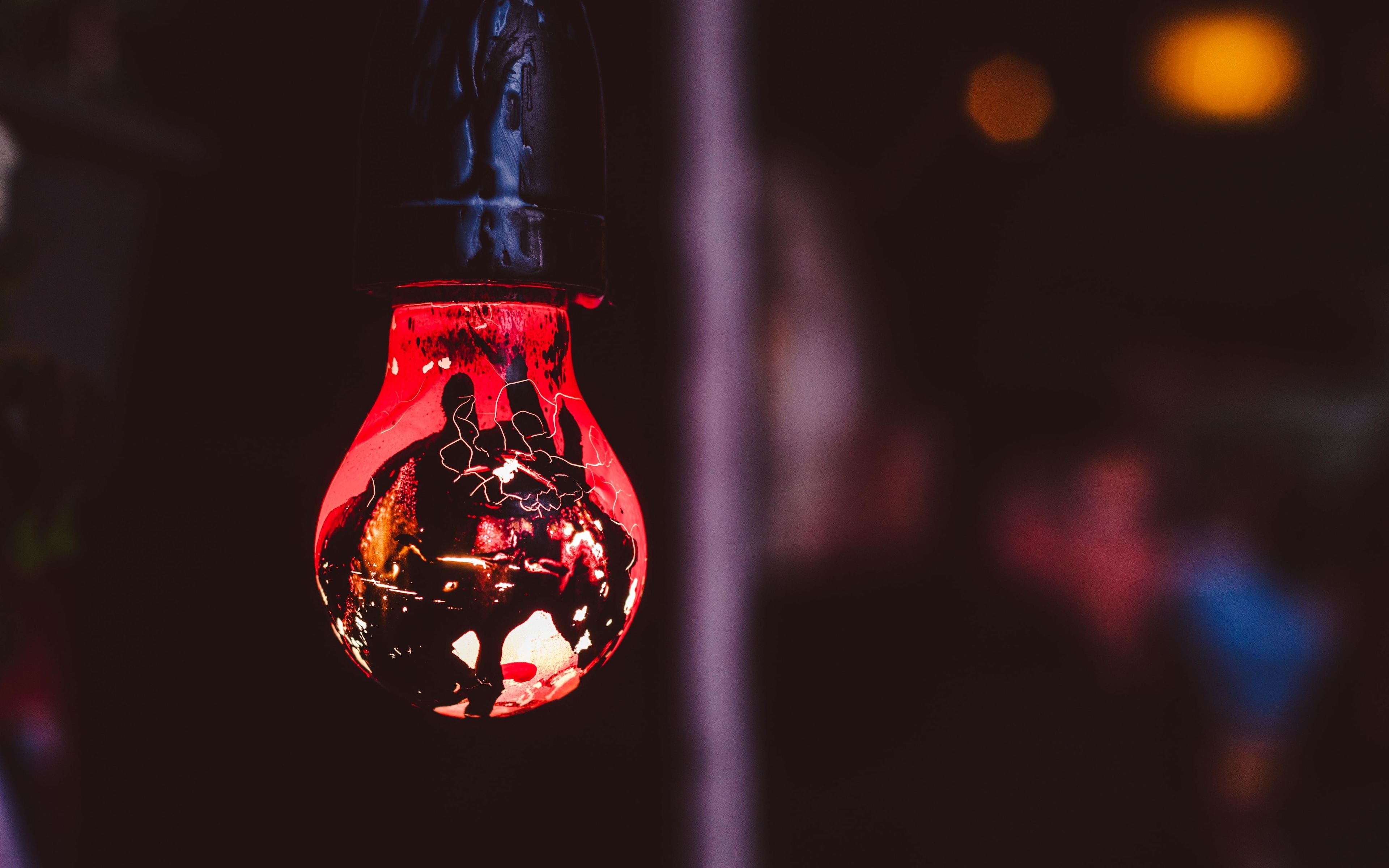
A step-by-step approach to AI adoption sets out a roadmap for AI in manufacturing. Image: Unsplash/Shane Rounce
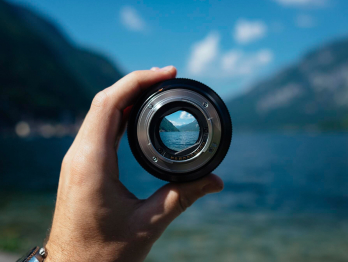
Get involved with our crowdsourced digital platform to deliver impact at scale
Stay up to date:
Artificial Intelligence
Listen to the article
- Artificial Intelligence (AI) can help manufacturers overcome supply chain shocks and economic headwinds.
- The manufacturing sector is struggling to harness the value AI can bring.
- A new report highlights barriers to AI in factories - and solutions for implementation.
- A step-by-step approach to AI adoption sets out a roadmap for AI in manufacturing.
The global manufacturing industry continues to be buffeted by the shockwaves from the pandemic and other disruptive global events. Supply chains for components such as semiconductors have still not recovered and the war in Ukraine is impacting a range of other value chains, particularly energy prices, which is having a knock-on effect on manufacturers.
These additional pressures are creating a need for manufacturers to “go beyond the traditional means of driving productivity to uncover the next wave of value for businesses, the workforce and the environment.” That’s a key finding from a December 2022 report by the Türkiye Centre for the Fourth Industrial Revolution in partnership with the World Economic Forum’s Network for Global Technology Governance.
The report, Unlocking Value from Artificial Intelligence in Manufacturing, finds that: “In principle, AI could unlock more than $13 trillion in the global economy and boost GDP by 2% per year. However, companies struggle to tap into the value that AI applications can create.”
And that’s not because manufacturers can’t see the value-creation potential of AI. In a global survey of 3,000 companies, 70% understood how AI can generate business value, 59% have an AI strategy in place and 57% of respondents said their organization was already piloting AI. Despite these findings, only 1 in 10 said AI was generating significant financial benefits.
How is the World Economic Forum ensuring the responsible use of technology?
Six barriers to AI adoption in manufacturing
The report’s authors drew on insights from industry leaders as well as technology and academic experts to identify the main barriers to AI adoption and scaling in manufacturing. They also developed step-by-step processes to overcome these roadblocks.
The report identities six major challenges facing manufacturers that are hindering AI deployment and the ability to scale.
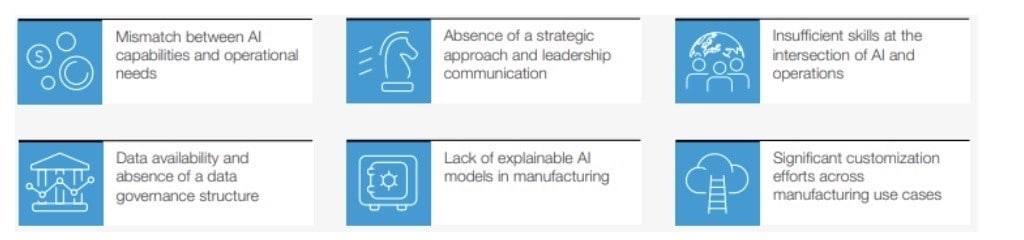
Below we detail the problems identified and the solutions offered in the report.
1. A mismatch between AI capabilities and operational needs
Organizations often neglect proper planning in the deployment of AI. They frequently fail to match the capabilities of AI platforms and the business pain points they are hoping to solve. This mismatch leads to failure and hinders the further adoption or scaling of AI in the business.
Solution: Businesses should build a robust business case with a problem-oriented approach. The aim is to define business needs and calculate the value that AI can bring in contributing to the solution.
2. The absence of a strategic approach and leadership communication
Businesses fail to draw up and implement a company-wide AI strategy and communication plan. This leaves the project lacking the right leaders to back the investment in AI and build support from end-users.
Solution: Adopt a strategic approach and communicate proactively to demonstrate the benefits of AI and the new processes it brings. This will increase employees’ willingness to embrace it in their routines.
3. Insufficient skills at the intersection of AI and operations
The leadership of AI projects is often handed to external consultants or traditional IT experts who have a limited understanding of AI.
Solution: AI applications must be developed and implemented by cross-functional teams with diverse knowledge of the convergence of IT, operations, data and AI technology.
4. Data availability and the absence of a data governance structure
Manufacturing companies often rely on limited data sets and siloed or fragmented data. This can severely limit the accuracy and value of insights from AI.
Solution: Create a single source of information that can be applied across the organization. Adopt a willingness to share data across traditional company boundaries to support AI adoption in the wider manufacturing sector.
5. A lack of explainable AI models in manufacturing
There’s a lot of misunderstanding of AI models and a perception that they are overly complex. Manufacturers require new AI platforms that build trust through transparency and deliver meaningful, explainable and accurate predictions.
Solution: Ensure the insights from AI are delivered in a format that manufacturers will understand and find relatable. This will speed the adoption of AI in the sector.
6. Significant customization efforts across manufacturing use cases
There is no one-size-fits-all application for AI in the manufacturing sector. Factories are complex ecosystems with specific engineering and technology related to industry sector and product output. It is not possible to deploy off-the-shelf AI models.
Solution: Further develop design, training and testing of AI models to allow for easy manual customization.
Five steps to successfully implementing AI in manufacturing
Having identified the major barriers to AI adoption, the report suggests a strategic, step-by-step approach for its successful implementation.
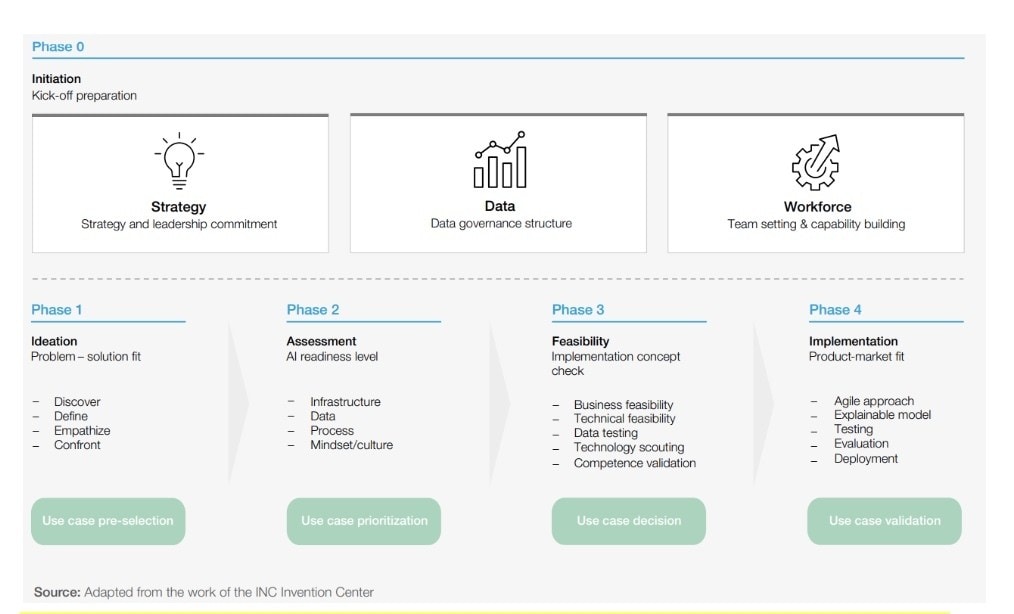
This approach begins with an initiation phase in which the project is endorsed by senior leadership. A data governance framework is put in place and cross-functional teams are established to drive the AI implementation.
An identification phase follows to identify and develop a set of use cases that will add significant value by solving specific business problems. The assessment phase determines whether the previously identified AI use cases can be incorporated into existing operations, IT and data systems and the organizational culture.
A feasibility phase follows which includes data testing, technology scouting to identify the best AI solutions and competence analysis to identify relevant skill sets in the workforce and areas for upskilling.
Finally, the implementation phase defines a roadmap for deployment with testing a validation of AI models and collaboration to ensure the needs of the end users are being met to eliminate fears around the impact of AI technology.
The research shows the huge potential of AI to transform the manufacturing sector and add exponential value. The report concludes: “With a holistic approach, AI can solve some of the most persistent problems in manufacturing and tap into new opportunities that allow companies to increase their operational performance, drive the sustainability agenda and empower the workforce.”
Don't miss any update on this topic
Create a free account and access your personalized content collection with our latest publications and analyses.
License and Republishing
World Economic Forum articles may be republished in accordance with the Creative Commons Attribution-NonCommercial-NoDerivatives 4.0 International Public License, and in accordance with our Terms of Use.
The views expressed in this article are those of the author alone and not the World Economic Forum.
The Agenda Weekly
A weekly update of the most important issues driving the global agenda
You can unsubscribe at any time using the link in our emails. For more details, review our privacy policy.
More on Emerging TechnologiesSee all
James Fell
April 26, 2024
Alok Medikepura Anil and Uwaidh Al Harethi
April 26, 2024
Thomas Beckley and Ross Genovese
April 25, 2024
Robin Pomeroy
April 25, 2024
Beena Ammanath
April 25, 2024
Vincenzo Ventricelli
April 25, 2024