How generative AI can benefit scientific experiments
Generative AI can enable scientists to overcome, at scale, longstanding scientific issues surrounding experiments. Image: REUTERS/Lori Shepler
Brian Jabarian
Howard and Nancy Marks Principal Researcher, University of Chicago Booth Business School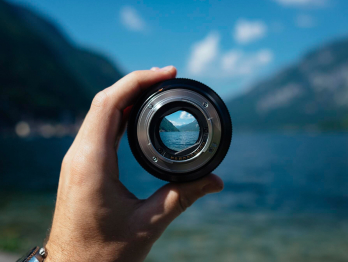
Get involved with our crowdsourced digital platform to deliver impact at scale
Stay up to date:
Tech and Innovation
Listen to the article
- Generative AI will have a seismic impact on scientific knowledge production across social sciences, in particular, on how we conduct experiments.
- Experiment design, implementation and data analysis can all be augmented by the use of generative AI.
- While integrating AI into scientific research necessitates a cautious approach to mitigate risks such as bias and privacy concerns, the potential benefits are monumental.
The recent emergence of generative artificial intelligence (AI) – applications of large language models (LLMs) capable of generating novel content – has become a focal point in economic policy discourse, capturing the attention of the EU, the US Senate and the UN.
This radical innovation, led by new specialized AI labs, including OpenAI and Anthropic, and financially supported by traditional "big tech" such as Microsoft and Amazon, is not merely a theoretical marvel; it is already reshaping markets from creative industries to health and many others. However, we are simply at the cusp of its full potential for the economy and humanity's future.
One domain poised for seismic change, albeit in its nascent stages, is scientific knowledge production across social sciences. Experimental methods are seminal for knowledge progress in social sciences, and they are the bedrock upon which technological revolutions are built and policies crafted.
As we suggest in our recent study, Generation Next: Experimentation with AI, integrating generative AI into scientific experimentation can revolutionize the practice of online experimentation to test theories involving multiple actors, from researchers to entrepreneurs and policy-makers, in different, scalable ways. It can do so by easing its deployment in different organizations, democratizing scientific education, and fostering evidence-based and critical thinking across society.
How AI can benefit online experiments
Our paper identifies three pivotal areas where AI can significantly augment online experiments — design, implementation and data analysis — enabling us to overcome, at scale, longstanding scientific issues surrounding online experiments.
1. In experimental design, LLMs can generate novel hypotheses by evaluating existing literature, current events, and seminal problems in a field. Their extensive training enables them to recommend appropriate methodologies to isolate causal relationships, such as economic games or market simulations. Furthermore, they can assist in determining sample size and ensuring statistical robustness while crafting clear and concise instructions, which is vital for ensuring the highest scientific value of experiments. Furthermore, they can transform plain English into different coding languages, allowing us to deploy experiments across different settings.
2. Recent evidence suggests that, in different settings, granting humans access to AI-powered chat assistants can significantly increase their productivity. AI assistance allows human support to provide faster and higher quality responses to more people.
This technique can be imported to experimental research, where participants might need clarification of the instructions or have other questions. AI’s scalability allows for the simultaneous monitoring of multiple participants, thereby maintaining data quality by detecting live engagement levels, cheating or erroneous responses. In addition, automating the data collection process through chat assistants reduces the risk of experimenter bias or the “demand effect” (where participants try to give expected rather than authentic answers) that influences participant behaviour, resulting in a more reliable evaluation of research questions.
3. In the data analysis phase, LLMs can employ state-of-the-art natural language processing techniques to explore new variables, such as participant sentiments or engagement levels. Using natural language processing (NLP) techniques on live chat logs from experiments can yield insights into participant behaviour, uncertainty and cognitive processes. They can automate data pre-processing, conduct statistical tests, and generate visualizations, allowing researchers to focus on substantive tasks.
During data pre-processing, language models can distil pertinent details from chat logs, organize the data into an analysis-friendly format and manage any incomplete or missing entries. Beyond these tasks, such models can perform content analysis – identifying and categorizing frequently expressed concerns of participants, analysing sentiments and emotions conveyed, and gauging the efficacy of instructions, responses and interactions.
The integration of LLMs into scientific research, however, does have its challenges. There is an inherent risk of biases in their training data and algorithms. Researchers must be vigilant in auditing these models for discrimination or skew. Privacy concerns are also paramount, given the vast amounts of data these models process, including sensitive participant information. Moreover, as LLMs become increasingly adept at generating persuasive text, the risk of deception and the spread of misinformation loom large.
Over-reliance on standardized prompts to generative AI could potentially stifle human creativity, necessitating a balanced approach that takes advantage of AI’s capabilities and human ingenuity.
How is the World Economic Forum ensuring the responsible use of technology?
While integrating AI into scientific research necessitates a cautious approach to mitigate risks such as bias and privacy concerns, the potential benefits are monumental. LLMs offer a unique opportunity to distil a culture of experimentation in firms and policy at scale, allowing for systematic, data-driven decision-making instead of reliance on intuition, which can increase workers’ productivity.
In policy-making, LLMs can facilitate the piloting of policy options through low-cost, randomized trials, thereby enabling an iterative, evidence-based approach. If these risks are judiciously managed, generative AI offers an invaluable toolkit for conducting more prolific, transparent and data-driven experimentation without diminishing the essential role of human creativity and discretion.
For more on this topic, read this Twitter thread from co-author Brian Jabarian.
Don't miss any update on this topic
Create a free account and access your personalized content collection with our latest publications and analyses.
License and Republishing
World Economic Forum articles may be republished in accordance with the Creative Commons Attribution-NonCommercial-NoDerivatives 4.0 International Public License, and in accordance with our Terms of Use.
The views expressed in this article are those of the author alone and not the World Economic Forum.
The Agenda Weekly
A weekly update of the most important issues driving the global agenda
You can unsubscribe at any time using the link in our emails. For more details, review our privacy policy.
More on Emerging TechnologiesSee all
Robin Pomeroy and Linda Lacina
April 29, 2024
Maroun Kairouz
April 29, 2024
Tanya Milberg
April 28, 2024
Michelle Meineke
April 28, 2024
James Fell
April 26, 2024