5 ways pharma can safely leverage AI for drug discovery and development
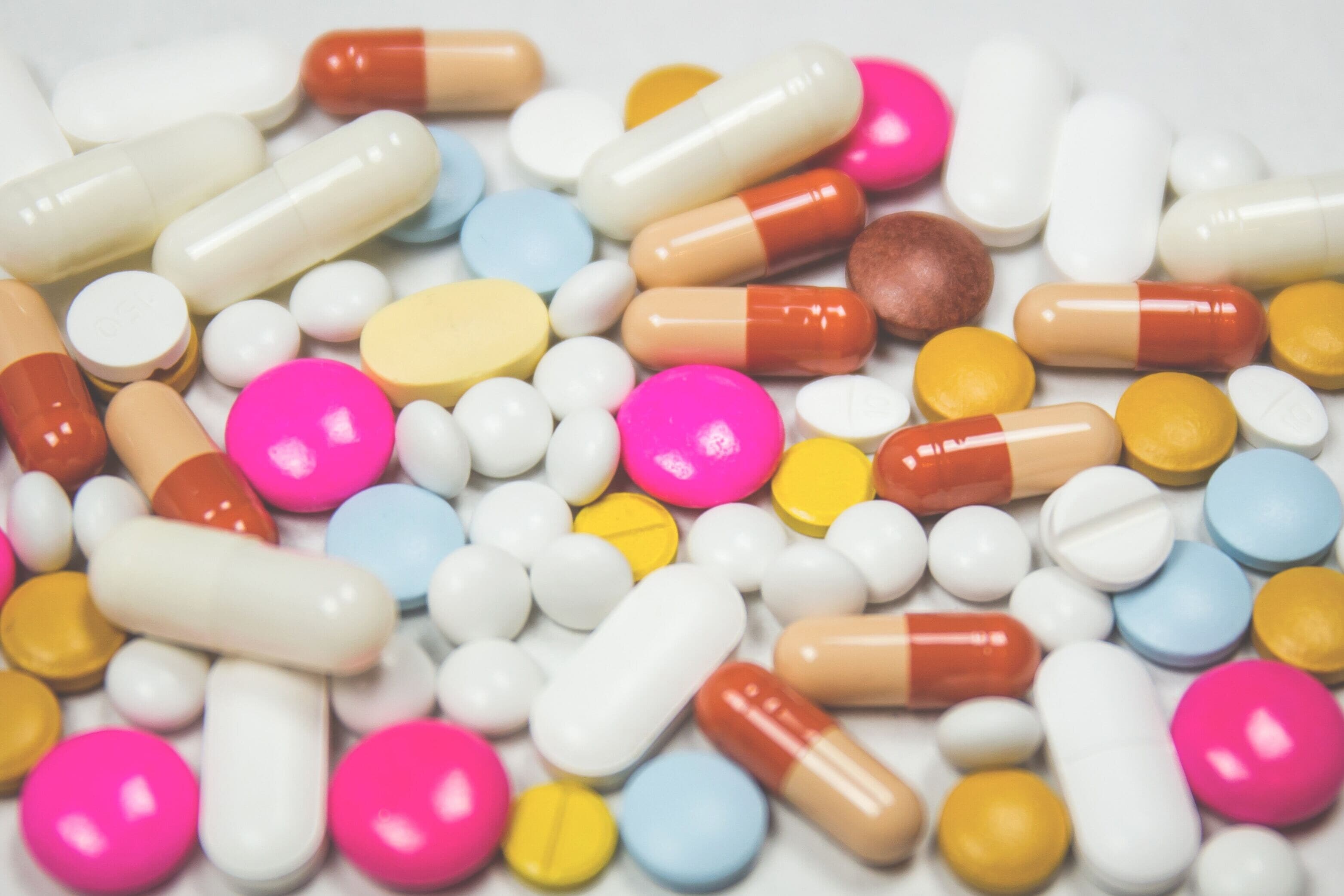
Here's how we can leverage AI for drug discovery and development. Image: Photo by freestocks on Unsplash
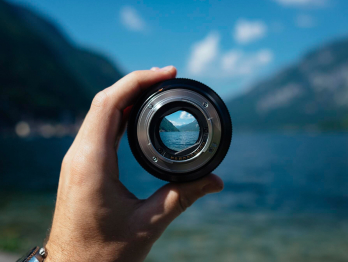
Get involved with our crowdsourced digital platform to deliver impact at scale
Stay up to date:
Health and Healthcare
- The promise of recent drug programmes was simple: get your hands on large datasets and machine learning will solve long-standing problems in the discovery, development and testing of new drugs. But many of these have stumbled recently.
- It’s important for the field and patients to understand why and redress these growing pains.
- Here's how we can clear 5 key hurdles and leverage AI for drug discovery and development.
In recent years, those applying computer science to biology have had no trouble raising the money to take their innovations straight into the commercial world. The promise was simple: get your hands on large datasets and machine learning will solve long-standing problems in the discovery, development and testing of new drugs.
Many of these early drug programmes have stumbled, however. It’s important for the field and patients to understand why and redress these growing pains. We’ve identified five key hurdles to success to date and show how to overcome them.
1. Patient data is fundamental
In the tech and biotech worlds, taking innovative know-how from an academic lab to solve a technical problem with well-defined datasets is a proven path to success. But the application of Artificial Intelligence (AI) to medicine - often called 'techbio' - is comparatively less about 'tech' and more about 'bio' in the fullest sense of the word.
The data required for success is often more varied, from more places and, critically, coming from actual patients within the complexity of multiple healthcare settings.
Too many people first turned to what was there and most straightforward: cell lines, chemical libraries and mouse models. Yet, this betrays the novel potential of AI. For the first time, we can now analyze truly massive and relatively new types of patient data at scale - from digital pathology slides to genomes or proteomic profiles. In other words, we no longer have to work with model simplifications of human biology.
And, since it’s all about patients, the data must be too. This means going beyond data-scraping and model systems to engage the participation of the patients the data comes from and the doctors and experts who work with them.
What is the World Economic Forum doing to improve healthcare systems?
2. Staying close to academia
In making medicine, human intelligence needs to come before - and after - the artificial kind. Universities and research hospitals are where it can be found. So, a critical factor in increasing the success of AI in making better medicine lies in forging deep and long-term partnerships with universities and research hospitals.
This is not a one-way street but a network of boulevards of constant interaction between academia, pharma and techbios. New ways of interacting, such as privacy-preserving federated learning, make it possible to train machine learning models remotely on vast multimodal data sets without moving them. This can power basic research, diagnostics and drug discovery, delivering a stream of benefits to patients while preserving privacy and maximizing the use of more diverse data.
3. Multi-dimensional problems require multimodal data
At this stage of the AI journey in medicine, we can confidently say that AI successfully gives us a new way of seeing human biology.
One digital pathology slide, for example, contains as much data as a feature-length film and only machine learning can take full advantage of it all. Yet, to understand disease and be able to change its course, it's essential to embrace the complexity of biology at every scale — from molecules to cells, tissues, the disease microenvironment and the organism as a whole.
To do this, we must develop AI that goes beyond unimodal tasks. We need to work with medical images and the latest molecular profiling technologies that can record the activity and location of biomolecules (called spatial omics), bridging the gap between the microscopic and macroscopic. With AI married to such datasets, we can understand disease from predisposition to progression and treatment.
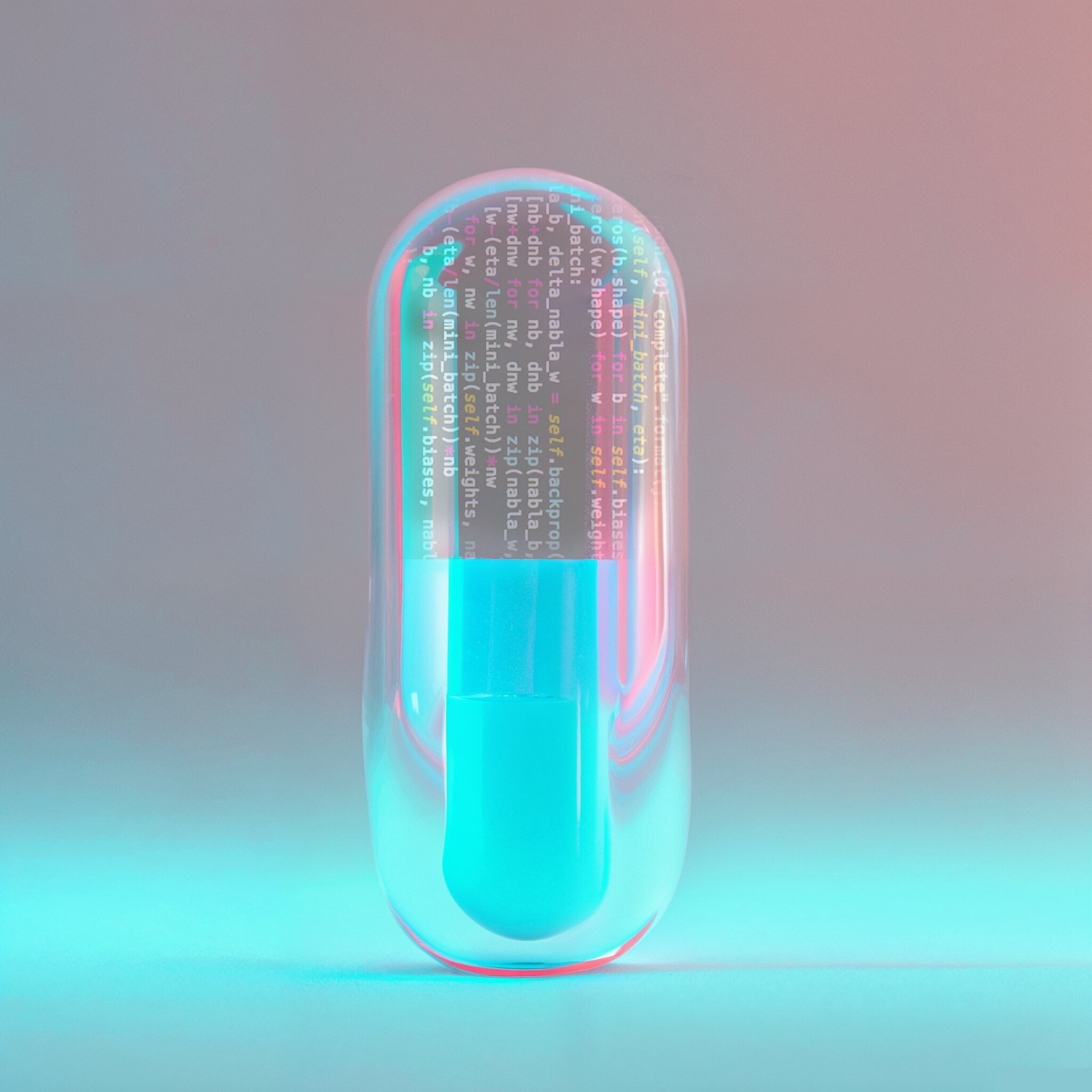
4. Improve the odds in clinical trials
The vast majority of experimental therapies fail in clinical trials. This is hugely expensive for society and industry alike. Fewer potentially promising treatments reach patients, and the cost of these failures drag on the resources that would otherwise be available to fund new programmes in the many areas of unmet need.
A principal reason for this is the structure of the trials themselves. In an ideal world, drugs would target the underlying biology of well-defined diseases and be tested only on those with that disease. Clinical trials could then be very small and nearly always successful. In practice, we don’t know nearly as much as we should about diseases, how drugs work or the exact disease type of trial participants.
AI can help us deliver on these steps better than we imagined only a few years ago. For the first time, machine learning enables us to map entire disease processes. Eventually, that will give us exciting new therapies.
But, even today, and even with existing medications, we can use AI-driven diagnostics to better diagnose patients, enrol those likeliest to respond in clinical trials, and even optimize trial covariates - which too often muddy the statistical power of trials - to stack the odds of success in our favour.
5. Regulators as partners in innovation
Alongside its immense potential, AI raises regulatory challenges around algorithms’ potential for bias, the appropriate use of personal data and, most importantly, ensuring patient benefit.
Regulators like the European Medicines Agency (EMA) and the US Food and Drug Administration (FDA) are highly engaged with these new technologies, applying their prudent, risk-based approaches to precision medicine innovation. Understandably, the increased focus on AI/ML-powered medicine should allow for smaller clinical trial cohorts, better approval rates, and lower overall costs.
The industry can do its bit to help regulators by clearly showing the potential and any risks in their research. Explainable AI and Good Practice (GxP) standards provide useful guidelines and transparent models and peer-reviewed, published results bolster best practices, accountability and public confidence.
Above all, a patient-centric research and ethical approach will shape the innovation landscape in a way that consistently prioritizes better health and care. That will accelerate the successful use of AI to transform how we discover, test and bring new therapies to market.
Don't miss any update on this topic
Create a free account and access your personalized content collection with our latest publications and analyses.
License and Republishing
World Economic Forum articles may be republished in accordance with the Creative Commons Attribution-NonCommercial-NoDerivatives 4.0 International Public License, and in accordance with our Terms of Use.
The views expressed in this article are those of the author alone and not the World Economic Forum.
Related topics:
The Agenda Weekly
A weekly update of the most important issues driving the global agenda
You can unsubscribe at any time using the link in our emails. For more details, review our privacy policy.
More on Health and Healthcare SystemsSee all
Shyam Bishen
May 13, 2024
Anna Cecilia Frellsen
May 9, 2024
Angeli Mehta
May 8, 2024
Emma Charlton
May 8, 2024
Kate Whiting
May 3, 2024