5 challenges for government adoption of AI
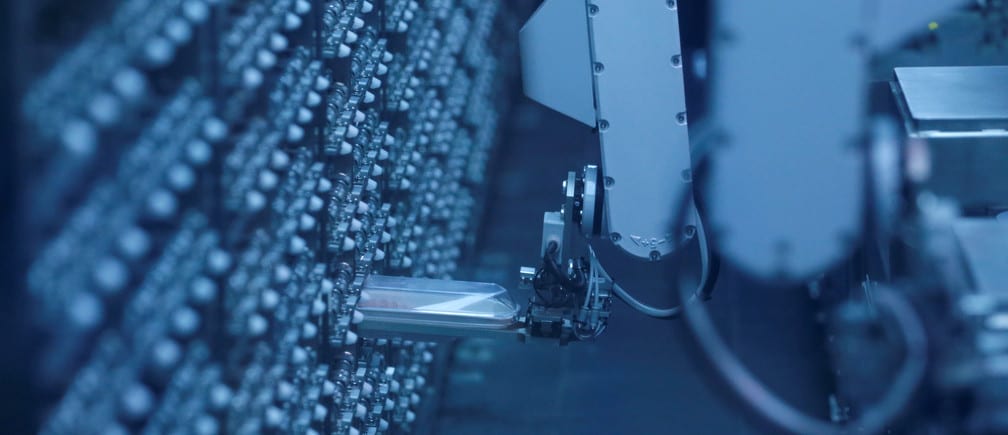
What's slowing the progress of widespread adoption of AI by government? Image: REUTERS/Jason Lee
Sabine Gerdon
Artificial Intelligence and Machine Learning Fellow, World Economic Forum, Senior Policy Adviser at the UK's Office for Artificial Intelligence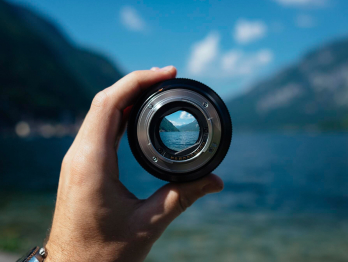
Get involved with our crowdsourced digital platform to deliver impact at scale
Stay up to date:
Emerging Technologies
From transportation solutions to video-streaming applications, artificial intelligence (AI) permeates almost every aspect of our lives. This includes government, where AI is increasingly making an impact. Consider the two examples below:
- Emma chatbot: US Citizenship and Immigration Services receives a considerable amount of service requests daily. In response, a chatbot named Emma was deployed to address immigration questions. Emma, which can operate in both English and Spanish, handles more than a million immigration queries a month.
- Firebird framework: Co-developed by Georgia Tech and the Atlanta Fire Rescue Department, Firebird helps the City of Atlanta prioritize buildings for inspection according to the building’s risk of fire.
Widespread adoption of AI has been slower in government than in the private sector. Given the magnitude of the impact that AI could have on public entities, it is important to understand the roadblocks that stand in the way of systemic government adoption of AI.

Through extensive stakeholder consultation we have identified five key barriers to AI adoption in government:
1. Effective use of data
We live in a digital world, and every day we leave behind a trail of digital data, from step counts to internet browsing patterns. IBM estimated in 2017 that 90% of the world’s data had been created in the past two years. The problem is, our organizations, both public and private, were not created to handle and take advantage of this volume and variety of data. Most organizations have a very rudimentary understanding of their data assets (i.e. the data they hold and the infrastructure that holds that data) and trying to answer even basic questions such as how many databases exist within the organization, which database contains what information, or how data is collected in the first place, can be challenging. This is a significant problem given data is the fuel that powers modern AI solutions.
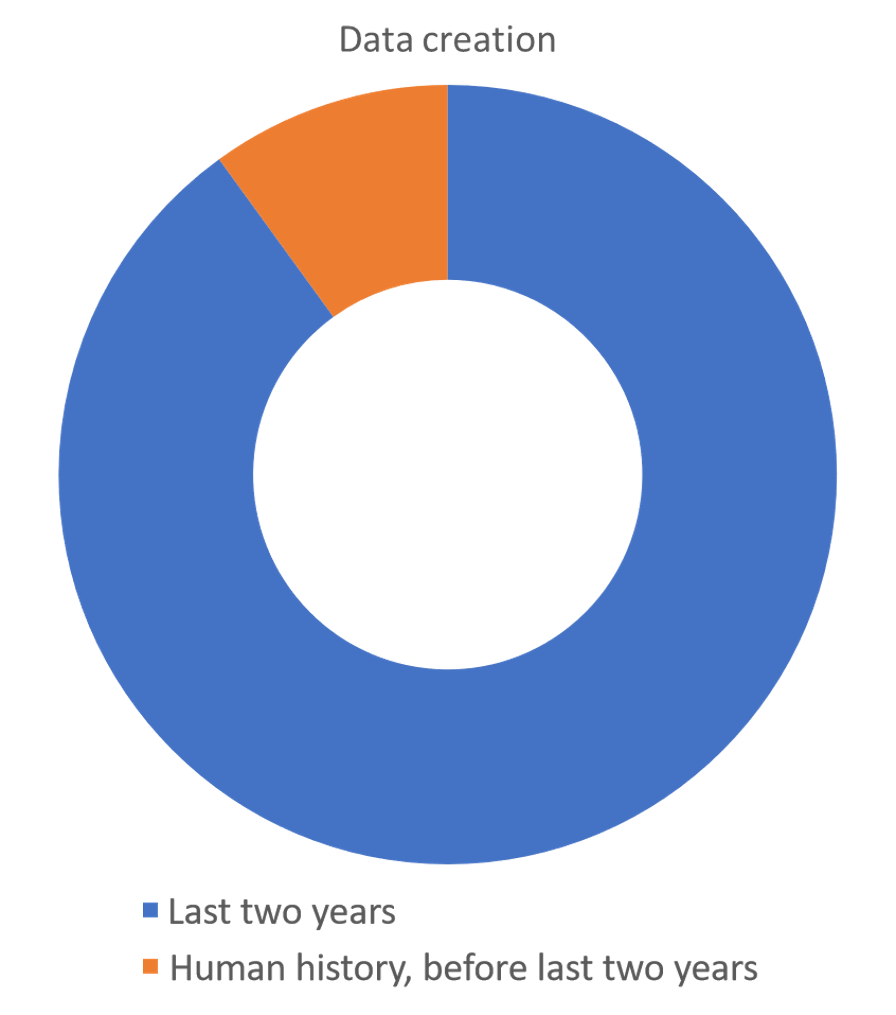
A parallel roadblock is that most organizations do not have data governance processes in place, such as established data owners, an enterprise data champion, such as a Chief Data Officer; tools for their employees to safely and efficiently access and take advantage of enterprise data, or practices to manage and ensure data privacy and integrity. Organizations that do not possess the capabilities to understand and manage their data cannot take advantage of AI.
2. Data and AI skills
AI and data management skills are in short supply. While the learning curve for data management is relatively surmountable, obtaining the required skills to develop AI solutions is much harder. Organizations place a premium on attracting high-calibre AI talent, raising compensation and making it difficult for organizations with smaller hiring budgets, like government, to attract top candidates. Public agencies find themselves without core AI skills, which hinders their ability to deploy and operate AI solutions.
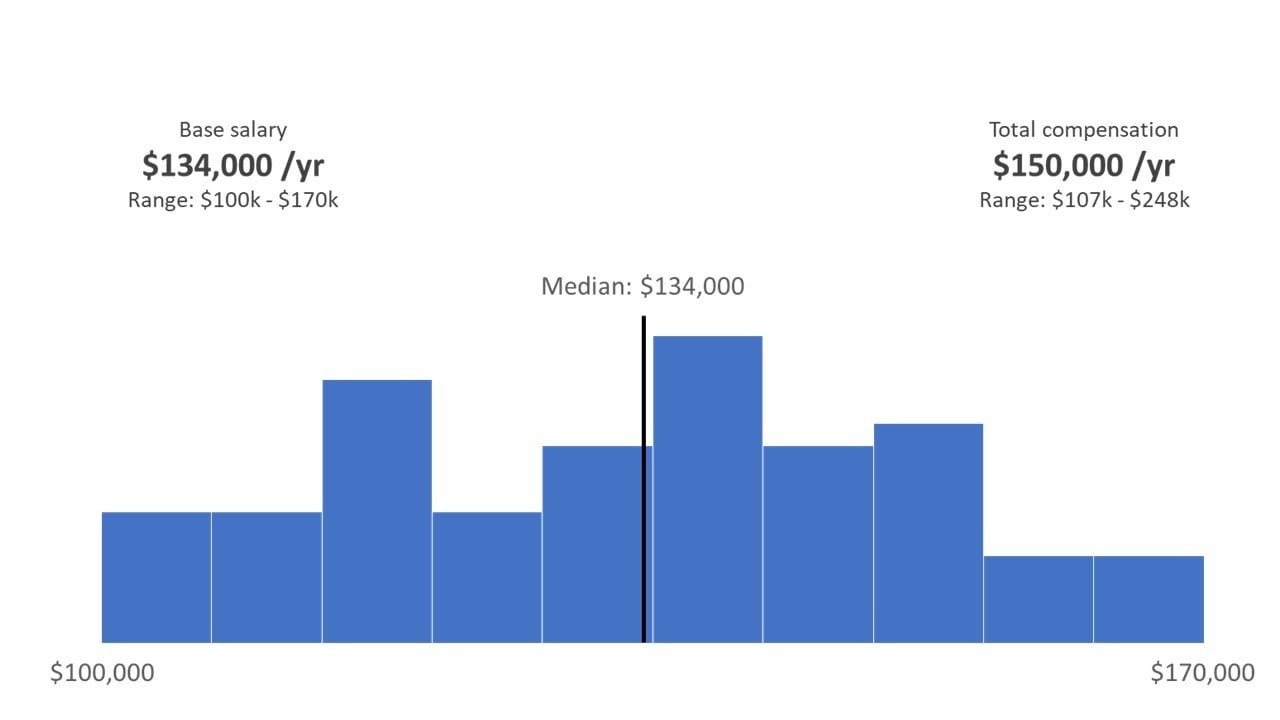
In addition, government personnel in non-technical roles, such as department directors, policy-makers, and procurement officials do not always have enough understanding about data and AI. This includes technical knowledge and most importantly knowledge of the legal and ethical implications of using vast amounts of data, where the main concern is privacy. This makes it difficult for them to feel comfortable investing in the technology, or be aware of existing laws that have a direct effect on AI projects, such as data and privacy legislation.
Embarking on AI projects without having a full understanding of applicable local laws threatens constituents’ rights, such as privacy, and the government’s long-term ability to deploy AI with full public support. This can have as large an effect on AI procurement as a lack of technical AI skills.
Government agencies that do have AI in-house knowledge face an added complexity: lack of communication. Silos between functions make it hard for AI resources and their colleagues, such as policy-makers, to have frequent touchpoints and take full advantage of each other’s knowledge.
3. The AI environment
The AI landscape is very complex and continuously evolving. In more established technology sectors there are a few well-known players so buyers know where to go. For example, the cloud landscape is dominated by Alibaba, Amazon, Google and Microsoft, which together account for approximately 84% of the global public cloud market. In contrast, the AI market, which also has significant presence from tech heavy weights, is more fragmented and has many small players continuously appearing. Consider the Canadian AI environment: a 2019 study conducted by Deloitte Canada identified more than 800 AI companies. Of these, a majority had fewer than 50 employees and more than 50% had been established in the last five years. The number of players and the speed of change in the AI market is large enough to potentially hinder a buyer who is new to the AI market. Many buyers might not even be aware of the complete landscape in the first place.
This diversity of players in this system stems from the numerous AI start-ups that have appeared across multiple geographies. This represents a second challenge for government: there is a considerable amount of AI know-how inside small, newer organizations that have limited experience working with government and difficulty scaling for large projects. Governments need to find ways to involve these newer players, both to utilize their considerable expertise, and to foster the growth of AI industry hubs that can contribute significantly to the local economy.
4. Legacy culture
All organizations face challenges in adopting new technologies. However, public entities tend to be less agile than their private sector counterparts, owing in part to their established practices and processes. In parts of the private sector a strong culture for experimentation encourages employees to innovate, and positive performance is rewarded. In government there can be less encouragement for employees to take risks.
As an article from Apolitical states: “Government incentives for risk, meanwhile, don’t really exist. If you pull off a major improvement in service delivery, you don’t get a bump in compensation or promoted faster.”
While many government employees derive much of their work satisfaction from having the opportunity to positively impact society rather than from financial compensation, it can be difficult to adopt a transformative technology such as AI if agility is not inherent to the organization’s culture.
5. Procurement mechanisms
AI presents challenges that current procurement mechanisms do not address, for example, the private sector treats algorithms as IP. Governments who purchase off-the-shelf algorithms may want to be able to understand and edit them as required throughout the lifecycle of the tool – customization is very common in software procurement – yet AI providers are likely to object to this.
A parallel discussion is the inability of government to avoid vendor lock-in where algorithms are treated like IP, given the original provider would most likely not consent to other AI developers accessing their algorithm. For most technologies this affects maintenance, but for AI it also impacts the ability of government to continuously update the algorithm with new data in order to keep it relevant, significantly affecting its lifespan.
What is the World Economic Forum doing about the Fourth Industrial Revolution?
In addition, public procurement mechanisms are well known for being slow and complicated. Common impediments include extensive terms and conditions, long wait times from tender response submission to final decision and proposals that ask for specific solutions as opposed to focusing on the challenge or opportunity at hand. These factors make it problematic for providers to respond, especially small enterprises. For example, long wait times make it very difficult for small enterprises to commit to future staffing requirements, since they usually need to staff resources on projects as soon as one becomes available, in order to manage a positive cash flow.
Government faces significant challenges for widespread AI adoption. Contrary to the popular belief that technology is the main roadblock, technical challenges form just part of the task at hand and this is the most straightforward part to address. Culture and processes, both ingrained in organizations, also need adjustment before AI can be fully exploited.
Don't miss any update on this topic
Create a free account and access your personalized content collection with our latest publications and analyses.
License and Republishing
World Economic Forum articles may be republished in accordance with the Creative Commons Attribution-NonCommercial-NoDerivatives 4.0 International Public License, and in accordance with our Terms of Use.
The views expressed in this article are those of the author alone and not the World Economic Forum.
Related topics:
The Agenda Weekly
A weekly update of the most important issues driving the global agenda
You can unsubscribe at any time using the link in our emails. For more details, review our privacy policy.
More on Emerging TechnologiesSee all
James Fell
April 26, 2024
Alok Medikepura Anil and Uwaidh Al Harethi
April 26, 2024
Thomas Beckley and Ross Genovese
April 25, 2024
Robin Pomeroy
April 25, 2024
Beena Ammanath
April 25, 2024
Vincenzo Ventricelli
April 25, 2024