How to use mobile phones to prevent the spread of disease
Human movement can play an important role in spreading disease in areas where incidence has been reduced.
Image: REUTERS/Yuya Shino
Stay up to date:
Health and Healthcare
The Global Fund has disbursed nearly $28.4 billion in the last decade to reduce the disease burden from malaria, TB and HIV (Global Fund 2016). However, travelers can reverse the progress from campaigns that have decreased infectious disease prevalence (Cohen 2012 et al, Lu et al 2014), or can rapidly spread emerging diseases such as Ebola and Zika (Tam et al 2016, Bogoch et al 2016). While policymakers have largely targeted environmental drivers of malaria, this research provides evidence that human movement can play an important role in spreading disease in areas where incidence has been reduced. Given that migration has numerous economic and social benefits, policymakers face important trade-offs in designing policies to reduce travel-linked malaria cases. This paper provides a useful framework for identifying high-risk populations in order to reduce malaria incidence with minimal interference to movement patterns.
In my paper, I study malaria transmission in Senegal by quantifying the relationship between travel and spread of disease and demonstrating towards whom policies should be targeted. The main challenge in studying the impact of travel on disease and how to mitigate it is the difficulty of tracking population movement and infections. This paper overcomes this challenge by utilizing a new source of data---15 billion mobile phone records representing almost 90% of the adult population in Senegal---to track population movement at a high spatial and temporal resolution. I combine the mobility data with monthly data on confirmed malaria cases at the health facility level. This paper causally identifies the size of the negative externality from travelers, building on previous work that uses mobile phone data to categorize the transmission risk for a set of origins and destinations (Wesolowski 2012 et al). This is also one of the first papers to focus on strategies for who should a policy be targeted towards, instead of what policy should be implemented (Adda 2016, Cohen and Dupas 2010, Hammer 1993).
Do Travelers Matter for Spread of Malaria?
I first establish if a negative externality from travel exists. If travel simply relocates existing cases, there is limited rationale for additional government investments. If instead, travel generates new cases of malaria in an area where the disease has been substantially reduced, policies would be necessary to address this negative impact of travelers in order to prevent a resurgence of the disease.
To quantify the impact of travel on malaria cases, the paper builds on an existing epidemiological model of malaria propagation, which incorporates population movement and separates locally generated and imported cases. I use mobile phone data provided by Sonatel and Orange within the D4D Challenge. The data consists of 9 million SIM cards in Senegal in 2013 that I use to extract patterns of movement between different areas from the approximate location of 15 billion calls and texts. I use the origins, time and length of travel of incoming travelers from the mobile phone data and monthly malaria incidence data for the origins to compute the expected number of imported malaria cases. Focusing on the low malaria area of the country, I use a panel data strategy in which I estimate the impact of imported incidence on malaria incidence controlling for location fixed effects and time dummies.
I find that for each expected imported case of malaria entering, there are 1.6 cases reported at the closest health facility. If infected travelers only represented a relocation of a case without generating any externality in the form of additional infections, then for each expected imported case there should be just one case reported in the area. Since I use health facility area fixed effects, I identify the effect from changes in expected imported cases within a health facility area, controlling for malaria seasonality, and I take advantage of variation from the combination of number of travelers and where travelers enter from. In the study area, based on the size of the effect, on average travelers account for 30% of all malaria cases.
Who Should the Government Target?
Given these results, should the government stop all travel or target all travelers with an intervention? Travel has important social and economic benefits; therefore, preventing all travel will have high costs that outweigh the benefit, as discussed in Adda 2016. Additionally, in the study area in Senegal, there were almost 7 million travelers in 2013. If targeting each traveler cost only $0.50, it would result in a cost of $6,000 per case averted, 40 times higher than a normative cost-effectiveness benchmark.
Therefore, if a policy were to target travelers, only a subset of travelers should be targeted. I categorize travelers by the district they are coming from and the month in which they enter the low malaria area. I assign a cost to each district-month based on the number of travelers that would need to be targeted. The benefit for that district-month is how many cases of malaria would be treated (for cases entering) or averted (for cases generated by the imported cases). I consider four strategies for choosing which district-months to target.
Figure 1 shows the ordering of district-months based on the four strategies. These strategies are to choose randomly, choose randomly within the malaria season, choose by ordering district-months from highest to lowest incidence in the previous year, or choose by ordering district-months based on the least number of travelers targeted per case treated/averted as calculated from my analysis. The figure is zoomed in on the cost curve up to 100,000 travelers. It shows if 50,000 travelers are targeted, the benefit using the cost-effective policy averts around 30% more cases as compared to the next best policy of using the information on incidence from the previous year and almost 8 times more cases are averted as compared to randomly targeting during the malarial season.
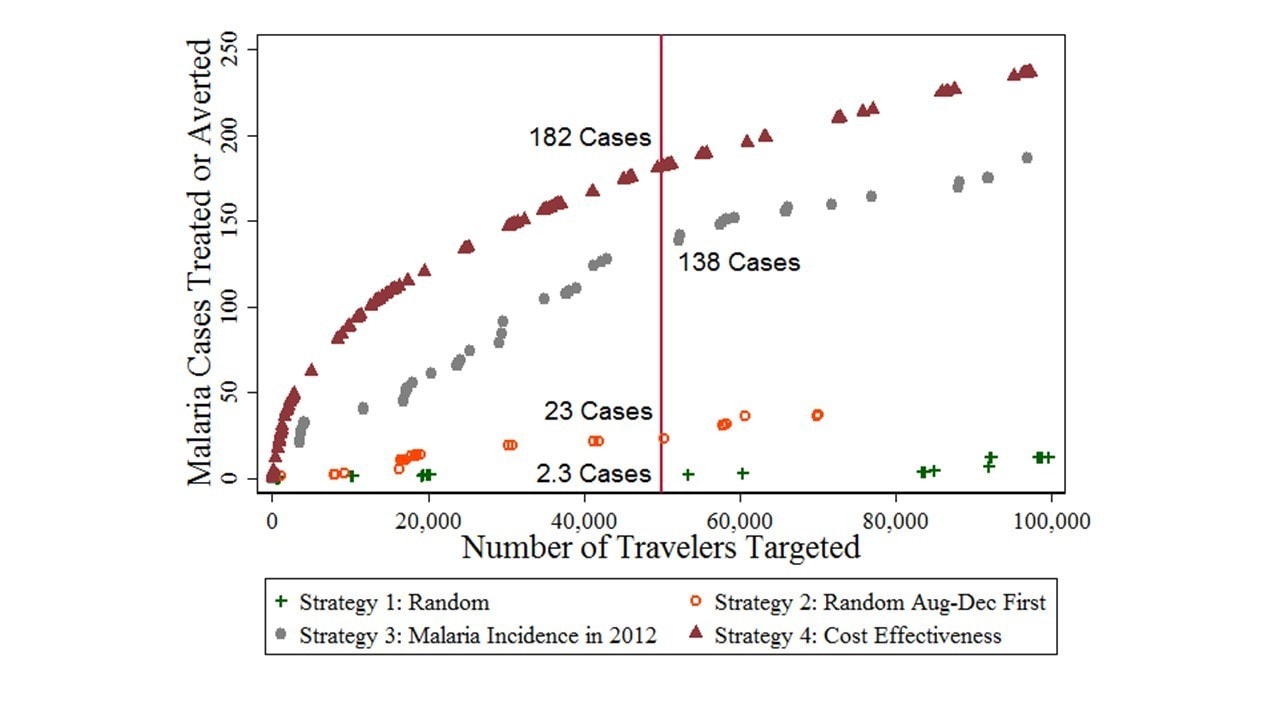
Implications
There are a few policies that could be targeted in this district-month manner. One policy could utilize the location based on the mobile tower from which a person makes or receives calls and texts. When a person changes location from a tower in a low malaria district to one in a high malaria district, an automated SMS could be sent highlighting the higher malaria risk and tips for avoiding mosquitoes. When a person travels from a high to a low malaria tower, an SMS could provide the location of the closest health facility and an incentive to visit it. A policy that would not require coordination with the mobile phone provider would be to send health workers to meet buses from certain locations upon arrival at the bus terminal to encourage travelers to get tested and to provide them with information.
The work here focuses on malaria, but it is possible to implement these types of models for other infectious diseases such as influenza, Ebola, or Zika. As cell phone usage becomes more prevalent throughout the developing world and cell phone providers begin to understand how the data they collect can be used by policy makers to implement better policies, measuring short term movement becomes much easier. What will then become necessary is the collection of high frequency data on all infectious diseases. This data will make it possible to study these types of models that help policymakers better target interventions, and in turn will make it easier to evaluate the interventions if case data are already being collected. This could help countries more effectively fight existing infectious diseases and prevent epidemics of new diseases.
Don't miss any update on this topic
Create a free account and access your personalized content collection with our latest publications and analyses.
License and Republishing
World Economic Forum articles may be republished in accordance with the Creative Commons Attribution-NonCommercial-NoDerivatives 4.0 International Public License, and in accordance with our Terms of Use.
The views expressed in this article are those of the author alone and not the World Economic Forum.
Related topics:
Forum Stories newsletter
Bringing you weekly curated insights and analysis on the global issues that matter.