How AI could dramatically improve your healthcare
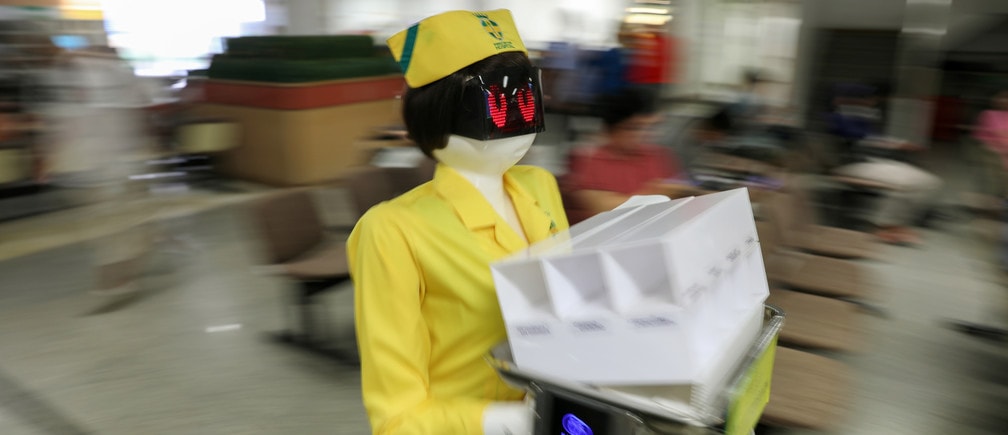
Faster, more accurate diagnoses. Image: REUTERS/Athit Perawongmetha
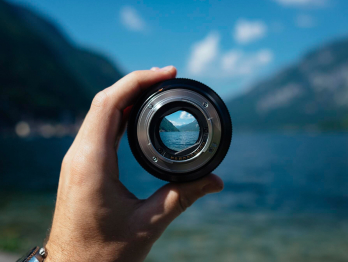
Get involved with our crowdsourced digital platform to deliver impact at scale
Stay up to date:
Fourth Industrial Revolution
When Google DeepMind’s AlphaGo shockingly defeated legendary Go player Lee Sedol in 2016, the terms artificial intelligence (AI), machine learning and deep learning were propelled into the technological mainstream.
BBC Newsnight: AlphaGo and the future of Artificial Intelligence.
AI is generally defined as the capacity for a computer or machine to exhibit or simulate intelligent behaviour such as Tesla’s self-driving car and Apple’s digital assistant Siri. It is a thriving field and the focus of much research and investment. Machine learning is the ability of an AI system to extract information from raw data and learn to make predictions from new data.
Deep learning combines artificial intelligence with machine learning. It is concerned with algorithms inspired by the structure and function of the brain called artificial neural networks. Deep learning has received much attention lately both in the consumer world and throughout the medical community.
Interest in deep learning surged with the success of AlexNet, a neural network designed by Alex Krizhevsky that won the 2012 ImageNet Large Scale Visual Recognition Challenge, an annual image classification competition.
Another relatively recent advancement is the use of graphical processing units (GPUs) to power deep learning algorithms. GPUs excel at computations (multiplications and additions) needed for deep learning applications, thereby lowering application processing time.
In our lab at the University of Saskatchewan we are doing interesting deep learning research related to healthcare applications — and as a professor of electrical and computer engineering, I lead the research team. When it comes to health care, using AI or machine learning to make diagnoses is new, and there has been exciting and promising progress.
Extracting blood vessels in the eye
Detecting abnormal retinal blood vessels is useful for diagnosing diabetes and heart disease. In order to provide reliable and meaningful medical interpretations, the retinal vessel must be extracted from a retinal image for reliable and meaningful interpretations. Although manual segmentation is possible, it is a complex, time-consuming and tedious task which requires advanced professional skills.
My research team has developed a system that can segment retinal blood vessels simply by reading a raw retinal image. It is a computer-aided diagnosis system that reduces the work required by eye-care specialists and ophthalmologists, and processes images 10 times faster, while retaining high accuracy.
Detecting lung cancer
Computer tomography (CT) is widely used for lung cancer diagnosis. However, because visual representations of benign (non-cancerous) and malignant (cancerous) lesions in CT scans are similar, a CT scan cannot always provide a reliable diagnosis. This is true even for a thoracic radiologist with many years of experience. The rapid growth of CT scan analysishas generated a pressing need for advanced computational tools to assist radiologists with the screening progress.
To improve radiologists’ diagnostic performance, we have proposed a deep learning solution. Based on our research findings, our solution outperforms experienced radiologists. Moreover, using a deep learning-based solution improves diagnostic performance overall and radiologists with less experience benefit from the system the most.
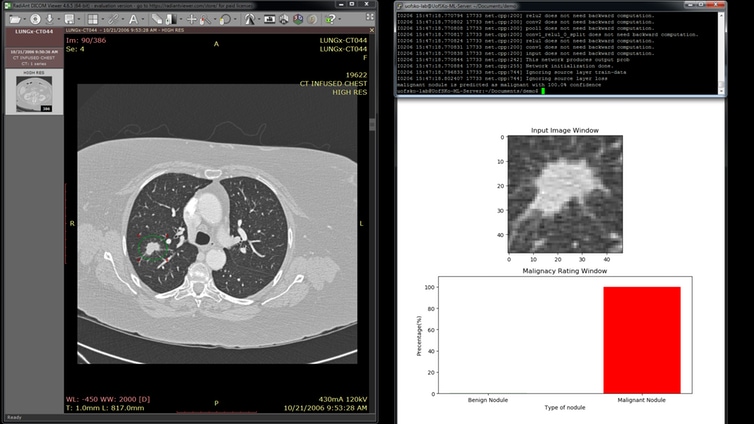
Limitations and challenges
Although great promise has been shown with deep learning algorithms in a variety of tasks across radiology and medicine, these systems are far from perfect. Obtaining high-quality annotated datasets will remain a challenge for deep learning training. Most computer vision research is based on natural images, but for healthcare applications, we need large annotated medical image datasets.
Another challenge from a clinical standpoint will be the time to test how well deep learning techniques perform in contrast to human radiologists.
There needs to be more collaboration between physicians and machine learning scientists. The high degree of complexity of human physiology will also be a challenge for machine learning techniques.
Another challenge is the requirements to validate a deep learning system for clinical implementation, which would likely require multi-institutional collaboration and large datasets. Finally, an efficient hardware platform is required to ensure fast processing of deep learning systems.
In the complex world of healthcare, AI tools can support human practitioners to provide faster service and more accurate diagnoses, and analyze data to identify trends or genetic information that may predispose someone to a particular disease. When saving minutes can mean saving lives, AI and machine learning may be transformative for healthcare workers and patients.
Don't miss any update on this topic
Create a free account and access your personalized content collection with our latest publications and analyses.
License and Republishing
World Economic Forum articles may be republished in accordance with the Creative Commons Attribution-NonCommercial-NoDerivatives 4.0 International Public License, and in accordance with our Terms of Use.
The views expressed in this article are those of the author alone and not the World Economic Forum.
The Agenda Weekly
A weekly update of the most important issues driving the global agenda
You can unsubscribe at any time using the link in our emails. For more details, review our privacy policy.
More on Fourth Industrial RevolutionSee all
Thomas Beckley and Ross Genovese
April 25, 2024
Agustina Callegari and Daniel Dobrygowski
April 24, 2024
Christian Klein
April 24, 2024
Sebastian Buckup
April 19, 2024
Claude Dyer and Vidhi Bhatia
April 18, 2024