What can ChatGPT teach us about economics?
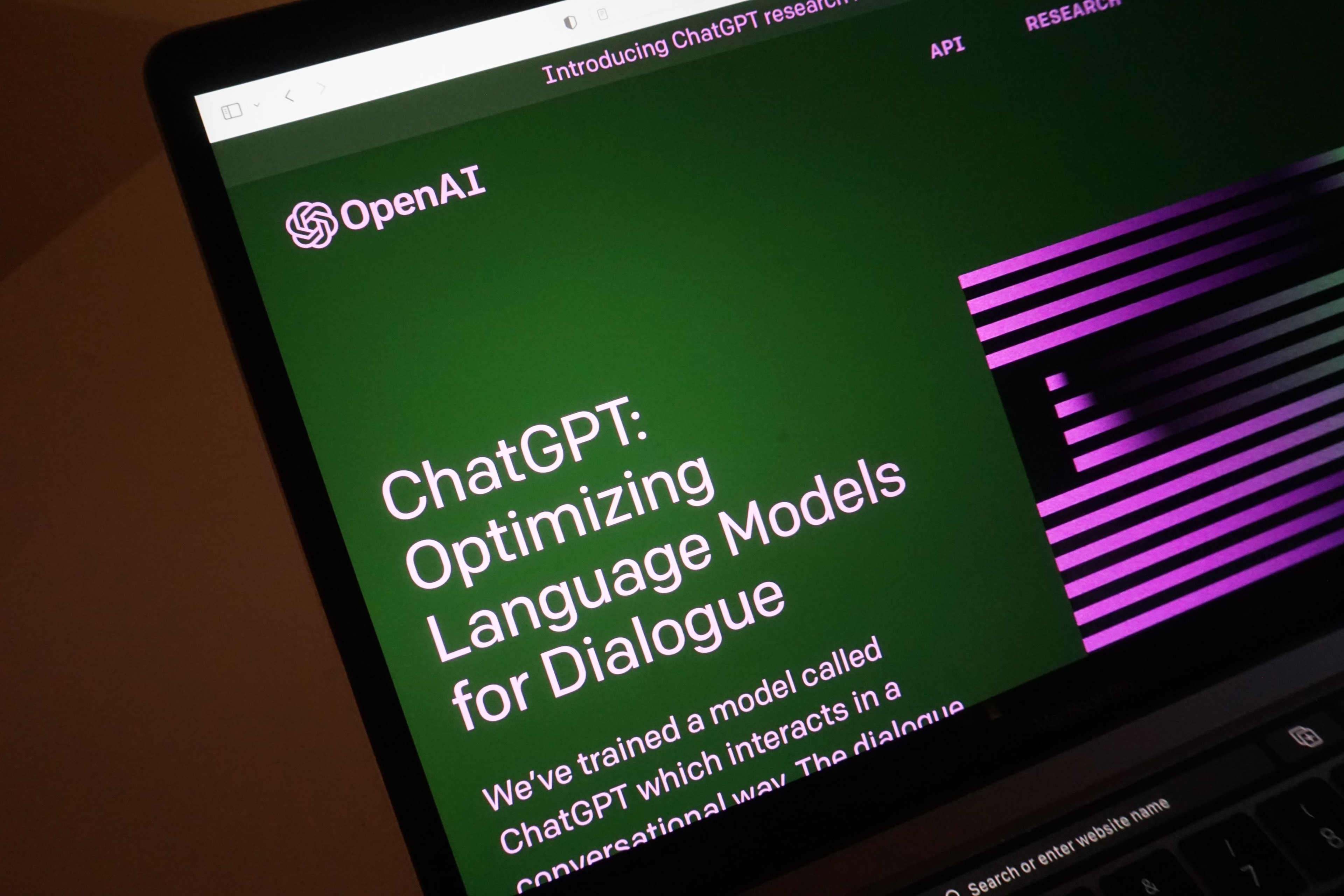
There is more to large language models, such as ChatGPT, than meets the eye.
Image: Photo by Rolf van Root on Unsplash
César A. Hidalgo
ANITI Chair, University of Toulouse; Honorary Professor, University of Manchester; Visiting Professor, Harvard University; Founder & CEO, DatawheelStay up to date:
Tech and Innovation
Listen to the article
- Economists can learn from large language models by deconstructing how they work.
- This is because, much like text, which involves complex interactions between many words, economies also involve complex interactions among a multifarious collection of people and objects.
- Economists should welcome this new methodological revolution and view it as a new wild west for creativity and experimentation.
Like everyone else, economists have been enjoying the foibles and virtues of large language models (LLMs). LLMs, such as ChatGPT, are enchantingly articulate. But one key question is, can we learn something about the economy from these models that we don’t already know?
I believe there is much that economists can learn from LLMs, not by chatting with the LLMs, but by deconstructing how they work. After all, LLMs are built on mathematical concepts that are powerful enough to help us simulate language. Maybe, understanding how these models work can become a new source of inspiration for economists.
To understand how LLMs work, it is useful to start with the most primitive version of a language-generating model. Imagine using a large corpus of text to count the number of times each word, such as brown, is followed by another word, such as dog. These two-word sequences are called 2-grams or bigrams. This matrix is a primitive language generation model. It is too simple to produce good text, but still 'smart' enough to have 'learned' that in English adjectives tend to precede nouns – the bigram brown dog is much more common than dog brown.
LLMs generalise this idea to n-grams. They are models that can estimate the probability of a word given a sequence of previous words (technically tokens, which are stubs of words such as archi, a part of the words architecture and archive). Certainly, n-gram matrices can explode in size. With 10,000 words, we have 100 million 2-grams, a trillion 3-grams and when we reach 18-grams, we have more combinations than the amount of information we could store using every atom in our planet (1072 combinations, when our planet can store around 1056 bits).
So, engineering LLMs must involve a clever idea. That idea is the use of neural networks to estimate a function describing all these sequences of words using only a few parameters. With nearly a trillion parameters, LLMs may seem large, but a trillion parameters is tiny compared to the n-grams in Borges’ library.
The result is models that begin to mimic knowledge. LLMs 'know' that tea and coffee are similar because they have learned that these words are used near words such as hot, drink and breakfast. By representing words, not as isolated entities but as nodes in networks, these models create the representations needed to generate language.
What has this to do with economics?
Much like text, which involves complex interactions between many words, economies also involve complex interactions among a multifarious collection of people and objects. Certainly, we can group these into predefined categories, such as capital and labour, or into activities, such as agriculture, service and manufacturing. But just like language models based on the idea of nouns, verbs and grammar are incomplete models of language, so will models based on coarse categorisations of economic activities. What LLMs teach us, is that there is a limit to our ability to capture the nuance of the world using predefined categories and deductive logic. If we want to get into the nitty-gritty, we need a mathematical toolbox that can help us capture systems at a finer resolution.
This idea is not entirely new. In fact, there are branches of economics that have long been using some of these ideas. Six years before the publication of Word2vec, a famous word embedding algorithm, together with three other colleagues we published a network representation of international trade. That network is technically a 2-gram, which represents products based on their relations to others. Just like in the coffee and tea example, this network 'knows' that drilling machines and cutting blades are related because they tend to be exported with a similar set of other products. The network 'knows' the difference between tropical and temperate agriculture and that between manufacturing t-shirts and LCD screens.
During the last fifteen years, these methods have found a growing audience among young economists and seasoned practitioners. On the one hand, they provide the tools needed to apply policy prediction ideas, such as anticipating the entry and exit of economies into different products and markets, to economic development. They’ve also resulted in 'embeddings' for economics (vector representations, such as the ones used to describe a word in a deep learning model). One example of this embedding is the Economic Complexity Index, a metric derived from a matrix of similarity among economies that explains regional and international variations in long-run economic growth, income inequality and emissions.
The ability of machine learning to gather, structure and represent data is creating opportunities for researchers across the board. From computational biologists looking to understand and predict the behaviour of proteins, to economic and international development experts looking to understand and predict the evolution of economies. Economists and computer scientists alike should welcome this new methodological revolution. It is a new wild west for creativity and experimentation.
Accept our marketing cookies to access this content.
These cookies are currently disabled in your browser.
Don't miss any update on this topic
Create a free account and access your personalized content collection with our latest publications and analyses.
License and Republishing
World Economic Forum articles may be republished in accordance with the Creative Commons Attribution-NonCommercial-NoDerivatives 4.0 International Public License, and in accordance with our Terms of Use.
The views expressed in this article are those of the author alone and not the World Economic Forum.
Forum Stories newsletter
Bringing you weekly curated insights and analysis on the global issues that matter.