Why shifting from prediction to foresight can help us plan for future disruption
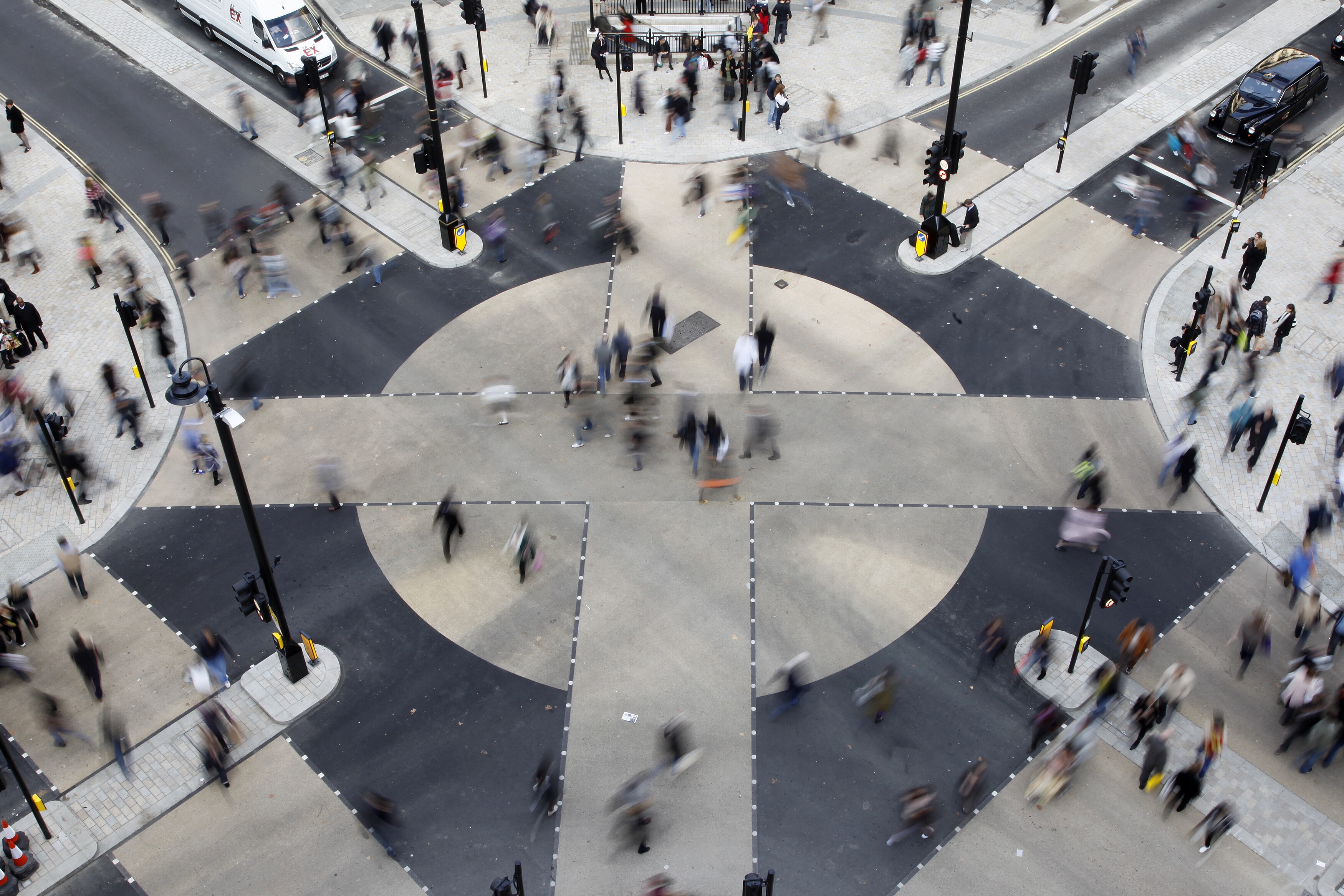
As the world becomes more complex, foresight methodologies account for a greater set of possible futures.
Image: REUTERS/Stefan Wermuth
Stay up to date:
Horizon Scan: Nita Farahany
- As the world becomes more complex, foresight methodologies account for a greater set of possible futures.
- Scenario development, a foresight methodology, is an alternative to prediction which can help map new possibilities.
- Foresight may predict possible futures but more importantly, it allows for preparation.
As the complexities of our world grow, the inherent uncertainty of the future intensifies.
In 1982, John Naisbitt defined megatrends as large, transformative processes with global reach and dramatic impact. While analyzing trends of any size can seem to yield insights into the future, they share a common weakness: trends are little more than rearview mirrors.
Extrapolating trends is dangerous, especially when they compound flawed assumptions. With time, assumptions magnify; wrong assumptions cascade and blow up.
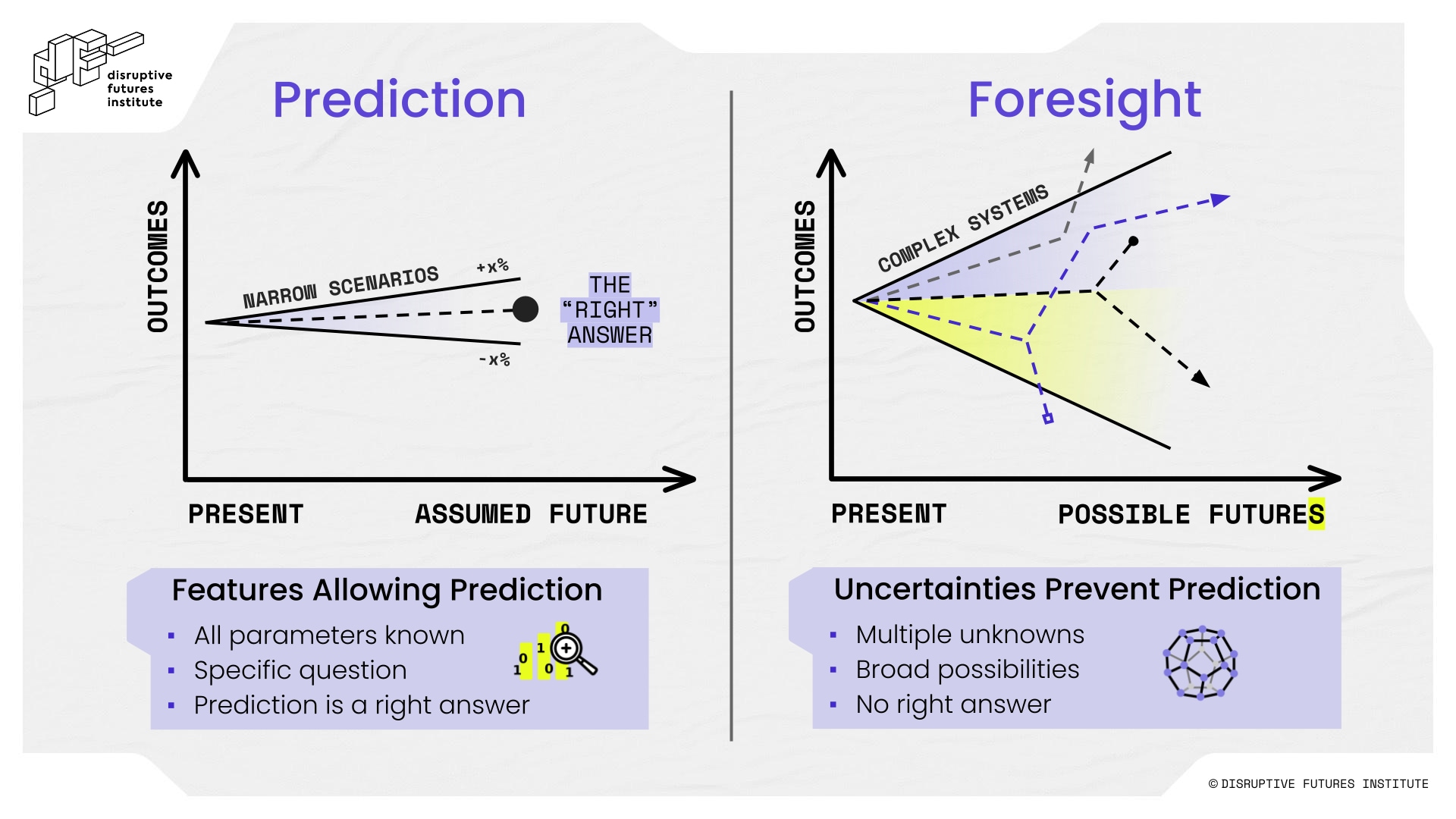
Systemic disruption marks the end of trends
In contrast to trends, disruptions are discontinuous. Systemic disruption - the cascade of constant disruptions across our increasingly complex and hyperconnected world - forces us to confront unpredictability.
With systemic disruption, the inability to understand the implications of initial impacts is why changes become apparent only in hindsight. As there may be no measurable data to substantiate our understanding, imagination outshines analysis.
Metaruptions drive systemic disruption
To contrast Naisbitt’s megatrends, the Disruptive Futures Institute coined the term “metaruptions”. A metaruption is a multidimensional family of systemic disruptions.
Metaruptions cause widespread, self-perpetuating effects that extend beyond their initial disruptions. As early changes spill over, impacts combine, propagate, and modify other elements within the system. Imagining the interplay of metaruptions is a creative endeavour, not a number-crunching exercise.
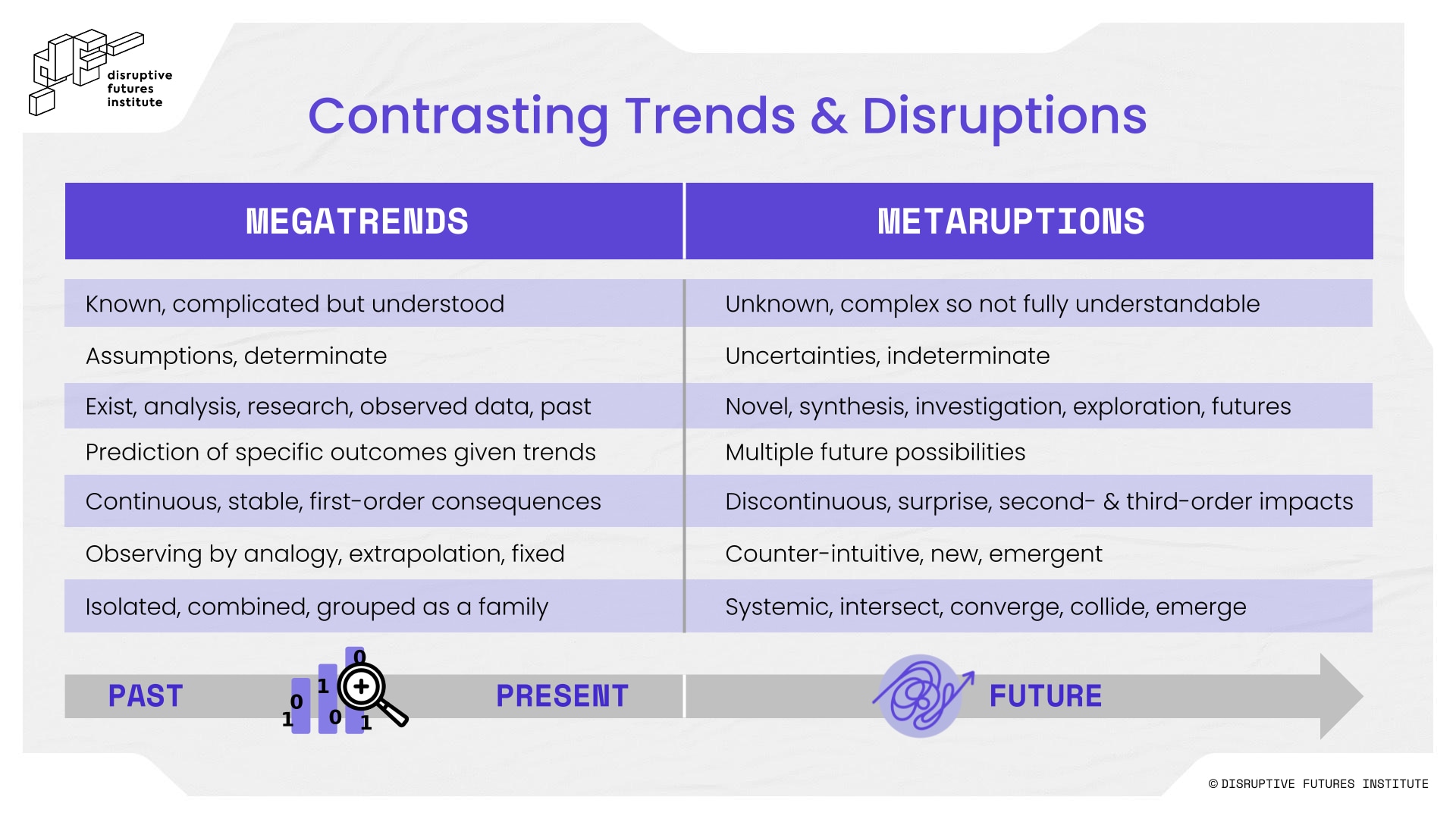
Does good judgment enable prediction?
In 2015, Philip Tetlock and Dan Gardner found that amateur forecasters were often more accurate than experts. Their book, Superforecasting, was based on data from the Good Judgment Project (GJP).
GJP’s research found that certain people (“superforecasters”) are extremely skilled at assigning probabilities to possible outcomes, because they take different perspectives, balance conflicting arguments, and update their beliefs.
But prediction is inherently speculative. So to understand why superforecasters are so skilled, we must reframe the degrees of uncertainty - and the nature of predictability itself.
Reframing predictability
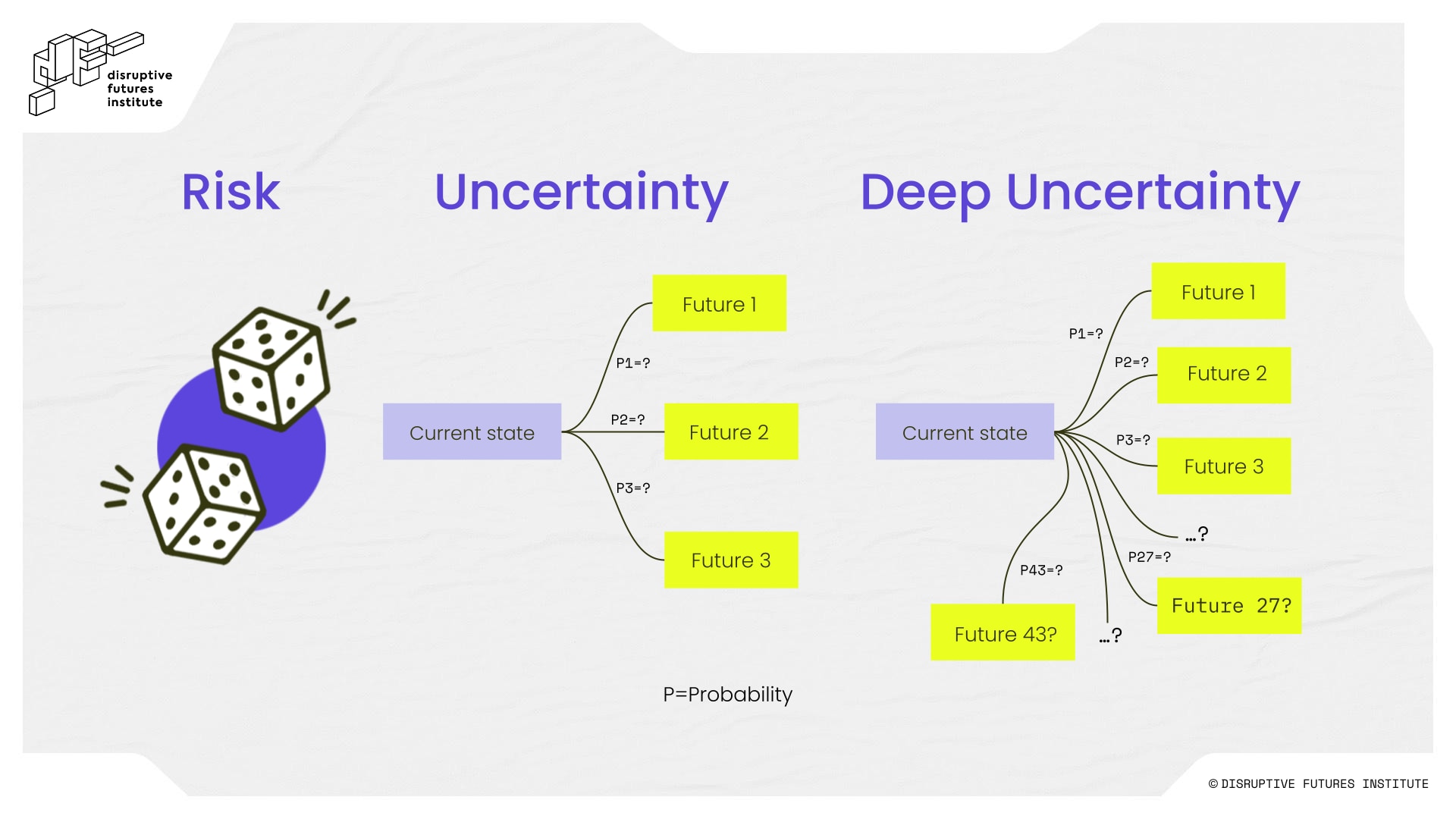
Evaluating predictability requires an understanding of the degrees of certainty:
- Risk - most predictable: All parameters, outcomes, and likelihoods are knowable.
- Uncertainty - some predictability: The nature of future events are known, but their probabilities are incalculable. Here, superforecasters thrive.
- Deep uncertainty - least predictable: Stakeholders cannot agree on the nature of potential future states, let alone their probabilities. Possible outcomes are numerous and unknowable; as events occur, their interplay with other dynamic scenarios may be unimaginable.
Deep uncertainty thwarts predictability
When parameters are known, we (and the superforecasters among us) can venture into predictions. However, in deep uncertainty, countless unknown variables hinder predictability.
The most challenging aspect of prognostication is that our complex world is deeply uncertain, and becoming increasingly so. Answering narrow, probability-based questions, such as GJP’s “Will North Korea launch a new multistage missile in the next year?”, is different from answering questions about the possible future states of deeply uncertain environments.
The table below compares the features that drive predictability and unpredictability:
Predictions beyond incentives and data
Predictions about the future should be scrutinized - nobody knows how the future will unfold. Anyone being paid to make predictions has a vested interest in the outcomes. Furthermore, consultants, economists, bankers, analysts, forecasters, and algorithms who claim to have data-driven predictive capabilities somehow extrapolate the past.
Despite the hype, artificial intelligence (AI) may not be much better than humans in nonlinear, dynamic, and complex systems. AI’s predictive strength lies in relatively stable situations that can be understood through pattern recognition at scale, given well-defined outcomes. In deeply uncertain environments, causality can only be assessed retrospectively, relationships are unpredictable, and moving parts interdependent.
While data is abundant, it failed to shield the world from the highest levels of inflation in half a century. The mighty US Treasury admitted its complete lack of understanding of what it labelled as “unanticipated” shocks to the economy.
Scenario development: The alternative to prediction
Traditional planning approaches assume a predictable world. Seeking to predict an answer is reasonable when addressing clearly defined questions in stable environments. However, when variables and their interactions are unknown, relying solely on predictions can be detrimental, especially when decisions hinge on their accuracy. The future is unmapped; you can’t rely on modelling uncertainties to deliver certainty.
Assumptions are unavoidable, so futures mindsets do not preclude their use. However, with foresight, we are not looking for a singular outcome. Foresight is the capacity to explore the possible futures systemically, as well as drivers of change, to inform short-term decision-making. We ask “why?, why not?, what if?” For these questions focused on the futures, there is no data.
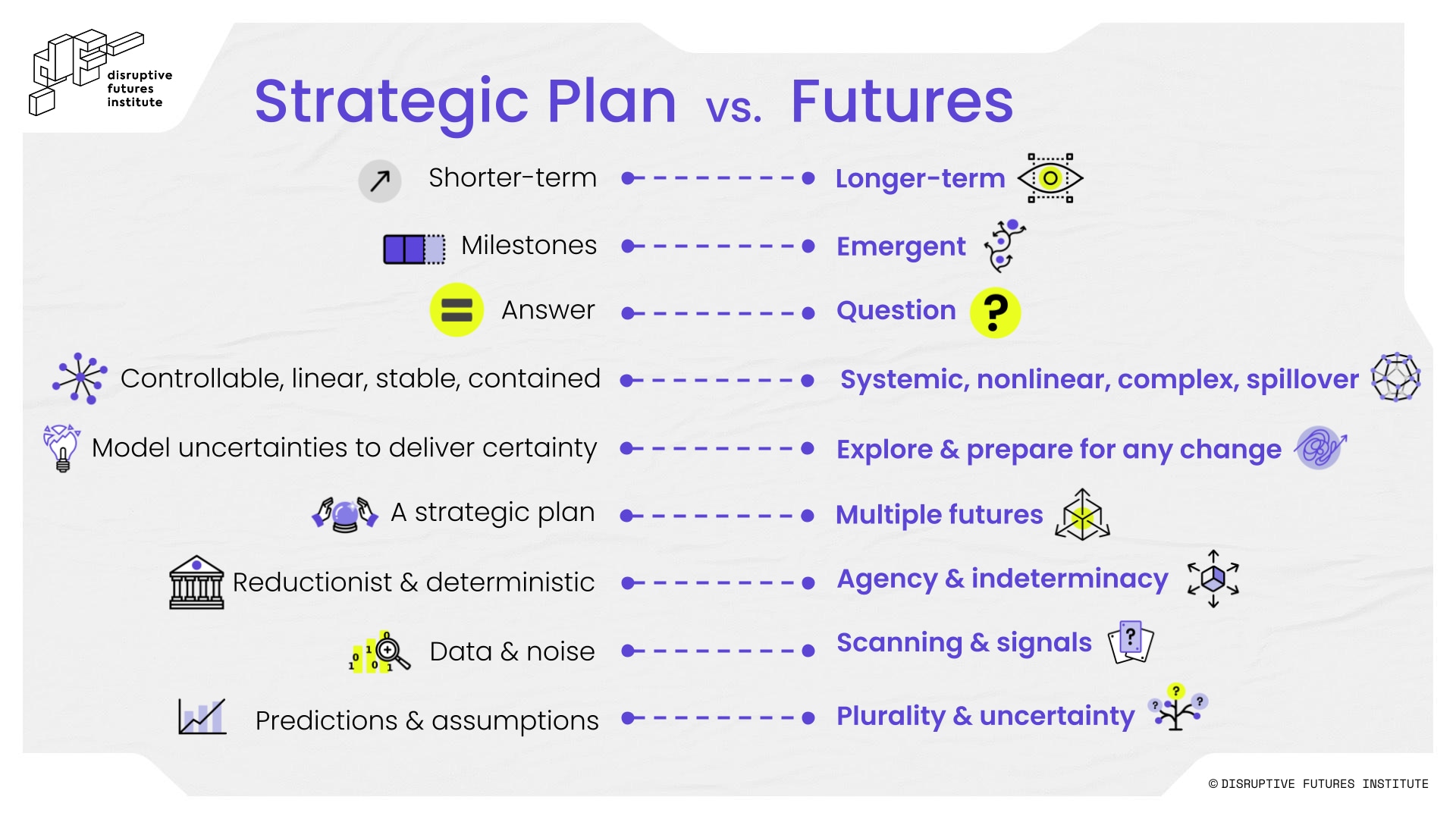
In foresight, insights from historical analysis and trends are helpful for sense-making, but only to provide a snapshot of the existing world as a base. Our imagination builds out different scenarios, outcomes, and possible futures.
Scenario planning was originally developed in the 1950s and 1970s, and it builds on linear strategic planning. The fundamental departures are that the futures are different from the past, longer timeframes matter, and next-order impacts need to be captured. Good scenarios help solve problems differently because they can illuminate new possibilities and ignite hope.
Thinking about long-term futures should never be to the detriment of the present. Only the present exists, so all our decisions towards long-term futures need to be translated to the now.
Foresight is preparation, not prediction
The purpose of scenario development is preparation, not prediction. This readying benefits any eventualities, beyond the handful of future scenarios imagined.
As we evaluate the opportunities and risks from our scenarios, we scrutinize their potential consequences. We can then build resilience to sustain even the most serious outcomes.
Foresight does not hold a crystal ball. It prepares you for the swerves. The future of prediction is imagination.
Accept our marketing cookies to access this content.
These cookies are currently disabled in your browser.
Don't miss any update on this topic
Create a free account and access your personalized content collection with our latest publications and analyses.
License and Republishing
World Economic Forum articles may be republished in accordance with the Creative Commons Attribution-NonCommercial-NoDerivatives 4.0 International Public License, and in accordance with our Terms of Use.
The views expressed in this article are those of the author alone and not the World Economic Forum.
Related topics:
Forum Stories newsletter
Bringing you weekly curated insights and analysis on the global issues that matter.
More on Global RisksSee all
William Dixon
July 16, 2025
Jesus Serrano
July 14, 2025
Naoko Tochibayashi and Mizuho Ota
June 27, 2025
Muhammad Hassan Dajana and James Balzer
June 27, 2025
Ajit Singh and Nicole Cowell
June 24, 2025
Marco Túlio Moraes
June 18, 2025