Why econometrics teaching needs an overhaul
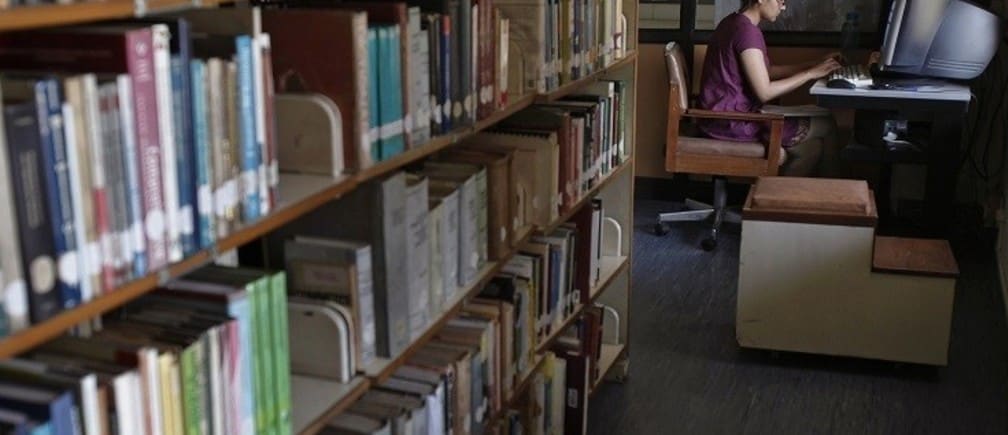
Stay up to date:
Economic Progress
The Global Crisis provoked some to ask, “what’s the use of economics”?, a reference to the economics that most economists had studied in college. We’d pile on, adding, “what’s the use of econometrics… at least as currently taught”? Most of the undergraduates who major in economics take a course in econometrics. This course should be one of the more useful experiences a student can have. For decades, economics undergraduates have found jobs in sectors that make heavy use of quantitative skills. As data sets have grown bigger and more complex, the demand for new grads with data-analytic skills has accelerated rapidly. Econometrics courses promise to equip our students with the powerful tools economists use to understand the economic relationships hidden in data. It’s both remarkable and regrettable, therefore, that econometrics classes continue to transmit an abstract body of knowledge that’s largely irrelevant for economic policy analysis, business problems, and even for much of the econometric research undertaken by scholars.
After a brief discussion of curve fitting, Pindyck and Rubinfeld’s (1976) first edition book began with subsections titled ‘The Model’, ‘Statistical Properties of Estimators’, and ‘Best Linear Unbiased Estimation’. The second edition of Johnston (1972) similarly started with models, assumptions, and estimators. Johnston describes multivariate regression models as “fitting the regression plane” a technical extension of the two-variable model that fits a line. The undergraduate econometrics canon has evolved little in the decades since. Becker and Greene (2001) surveyed econometrics texts and teaching at the turn of the millennium, arguing that “econometrics and statistics are often taught as branches of mathematics, even when taught in business schools… the focus in the textbooks and teaching materials is on presenting and explaining theory and technical details with secondary attention given to applications, which are often manufactured to fit the procedure at hand… applications are rarely based on events reported in financial newspapers, business magazines or scholarly journals in economics”.
The disconnect between econometric teaching and practice
Hewing to the table of contents in legacy texts, today’s market leaders continue to feature models and assumptions at the expense of empirical applications. Core economic questions are mentioned in passing if at all, and empirical examples are still mostly contrived, as in Studenmund (2011), who introduces empirical regression with a fanciful analysis of the relationship between height and weight. The first empirical application in Hill, Griffiths, and Lim (2011: 49) explores the correlation between food expenditure and income. This potentially interesting relationship is presented without a hint of why or what for. Instead, the discussion here emphasises the fact that “we assume the data… satisfy assumptions SR1-SR5”. An isolated bright spot is Stock and Watson (2011), which opens with a chapter on ‘Economic Questions and Data’ and introduces regression with a discussion of the causal effect of class size on student performance. Alas, Stock and Watson also return repeatedly to more traditional model-based abstraction.
The disconnect between econometric teaching and econometric practice goes beyond questions of tone and illustration. The most disturbing gap here is conceptual. The ascendance of the five core econometric tools – experiments, matching and regression methods, instrumental variables, differences-in-differences and regression discontinuity designs – marks a paradigm shift in empirical economics. In the past, empirical research focused on the estimation of models, presented as tests of economic theories or simply because modelling is what econometrics was thought to be about. Contemporary applied research asks focussed questions about economic forces and economic policy.
Consistent with today’s emphasis on specific causal effects, our own book, ‘Mastering ’Metrics’ (Angrist and Pischke 2015) introduces regression as a strategy to answer the question of whether it’s worthwhile spending upwards of $50,000 a year on private university tuition, as many American students do. Of course, students who attend relatively selective private schools are likely to have higher earnings for many reasons – this is the selection bias that plagues most naive comparisons. Regression is a control strategy that reduces this bias. Its value turns solely on the core notion that controlled comparisons are more likely than uncontrolled comparisons to have a causal interpretation. In contemporary econometric practice, the most widely-employed econometric tools are designed to capture specific causal relationships like the private-school earnings effect. Such questions are easily understood, and the answers to them have real consequences for real people, including, in many cases, for our students.
Pragmatism
The unapologetic focus on causal relationships that’s emblematic of modern applied econometrics emerged gradually in the 1980s and has since accelerated.1 Today’s econometric applications make heavy use of quasi-experimental research designs and randomised trials of the sort once seen only in medical research. In fact, the notion of a randomised experiment has become a fundamental unifying concept for most applied econometric research. Even where random assignment is impractical, the notion of the experiment we’d like to run guides our choice of empirical questions and disciplines our use of non-experimental tools and data.
The path to understanding econometric tools begins with causal questions motivated by economic reasoning. These questions are then answered with data and a focussed and carefully executed empirical analysis. What’s the effect of health insurance on health? Does arresting batterers reduce spousal abuse? Do peer effects matter for student achievement? Does central bank liquidity save banks in a banking crisis? Each of our methodological chapters starts with questions like these, posed generally, but answered specifically. We explain why these questions are challenging and why simple empirical strategies to address them can be misleading. Our econometric methods and tools are those used most heavily in modern applied economic research.
Our focus on five core econometric tools is a natural consequence of contemporary econometric practice, which owes little to the formalities of the classical linear regression model, the arcane statistical assumptions of generalised least squares, or the elaborate simultaneous equations framework that fill so many texts. We begin with randomised trials, which set our standard for research validity, moving on to a detailed but model-free discussion of regression, the tool most likely to be used by practitioners. Our regression application — estimating the effects of private college attendance on later earnings — shows the power of regression to turn night into day when it comes to causal conclusions.
Although instrumental variable regression was invented as a solution to the problem of estimating supply and demand curves, our take on instrumental variables corresponds to the way instrumental variable regression is most often used now: as a solution to the problem of selection bias. By contrast, Hill, Griffiths, and Lim (2011) introduce instrumental variable regression in an intimidating-sounding chapter on ‘Random Regressors and Moment-Based Estimation’. Following our instrumental variable regression chapter, built around three interesting uses of instrumental variable regression to capture causal effects, we tackle regression discontinuity designs and differences-in-differences methods. A search of Econlit reveals hundreds of papers published since 1990 using both of these methods. Yet they get no attention in Studenmund (2011) or Gujarati and Porter (2010), while Hill, Griffiths, and Lim (2011) and Wooldridge (2012) briefly attend to differences-in-differences only.
Not just for linear models
In addition to its more up-to-date contents, our book renews the econometrics canon by abandoning the childish literalism of the legacy approach to econometric instruction. In this spirit, we eschew the notion that regression is tied to a literal linear model. Regression describes differences in averages whether or not these averages fit a linear equation. This is a universal property – one that is reliably true – and we don’t intimidate readers with descriptions of the punishments to be meted out for the failure of classical assumptions. Our regression discussion begins by challenging readers to ask themselves, first, what the target causal effect is, and, second, by asking, ‘what is the regression you want’? In other words, what would you like to hold fixed when trying to regress-out an average causal effect? Omitted variables bias is the difference between this ideal regression and the regression you’ve got at hand. In the same spirit, we introduce instrumental variables as a method for solving compliance problems in a randomised natural experiment. This leads easily to a discussion of two-stage least squares as a powerful method harnessing variation in instruments originating from a wide variety of sources. We close with a question-driven synthesis of research on the economic returns to schooling, showing how different methods reveal important aspects of a single underlying causal relationship.
In our experience, most econometrics teachers enjoy working with data and they hope and expect that their students will too. Yet, a sad consequence of the inherited econometrics canon is its drabness. This is a genuine problem because modern applied econometrics is interesting, relevant and, yes, fun! Instructors who have as much fun teaching econometrics as they do when they use it in their research can hope to transmit their excitement to their students. This should be sharing excitement about data, we hope to plant the seeds of useful data analysis in the next generation of scholars, policymakers and an economically literate citizenry. The promise of our approach is evident in the popularity of the Freakonomics franchise and is also brought to life in the new introduction to economics principles book by Acemoglu, Laibson, and List (2015). Their take on economics puts questions and evidence ahead of abstract models. We’re happy to join these colleagues in an effort to polish and renew our profession’s rusty instructional canon.
References
Acemoglu, D, DI Laibson, and JA List (2015), Economics, Pearson.
Angrist, JD, and J-S Pischke (2015), Mastering Metrics: The Path from Cause to Effect, Princeton University Press.
Becker, WE, and WH Greene (2001), “Teaching Statistics and Econometrics to Undergraduates”,Journal of Economic Perspectives, 169-182.
Gujarati, DN, and DC Porter (2010), Essentials of Econometrics, McGraw-Hill.
Hamermesh, DS (2013), “Six Decades of Top Economics Publishing: Who and How?”, Journal of Economic Literature, 162-172.
Hill, RC, WE Griffiths and GC Lim (2011), Principles of Econometrics, Wiley.
Johnston, J (1972), Econometric Methods, McGraw-Hill.
Pindyck, RS, and DL Rubinfeld (1976), Econometric Models and Economic Forecasts, McGraw-Hill.
Stock, JH, and MW Watson (2011), Introduction to Econometrics, Addison-Wesley.
Studenmund, A H (2011), Using Econometrics: A Practical Guide, Addison-Wesley.
Wooldridge, JM (2012), Introductory Econometrics: A Modern Approach, South-Western Cengage Learning.
Endnotes
1 See, for example, Table 4 in Hamermesh (2013), which highlights the increasing analysis of user-generated data, much coming from experiments and quasi-experimental research designs.
This article is published in collaboration with VoxEU. Publication does not imply endorsement of views by the World Economic Forum.
To keep up with the Agenda subscribe to our weekly newsletter.
Authors: Josh Angrist is a Ford Professor of Economics and co-director of the School Effectiveness and Inequality Initiative, MIT. Jörn-Steffen Pischke is a Professor of Economics, London School of Economics; Senior research associate, Centre for Economic Performance.
Image: A student works on a computer in a library at Management Development Institute (MDI) in Gurgaon, on the outskirts of New Delhi May 2, 2012. REUTERS/Adnan Abidi.
Don't miss any update on this topic
Create a free account and access your personalized content collection with our latest publications and analyses.
License and Republishing
World Economic Forum articles may be republished in accordance with the Creative Commons Attribution-NonCommercial-NoDerivatives 4.0 International Public License, and in accordance with our Terms of Use.
The views expressed in this article are those of the author alone and not the World Economic Forum.
Related topics:
Forum Stories newsletter
Bringing you weekly curated insights and analysis on the global issues that matter.
More on Economic GrowthSee all
Silja Baller and Fernando Alonso Perez-Chao
July 11, 2025
Yusuf Maitama Tuggar
July 10, 2025
Resilience roundtable: How emerging markets can thrive amid geopolitical and geoeconomic uncertainty
Børge Brende, Bob Sternfels, Mohammed Al-Jadaan and Odile Françoise Renaud-Basso
July 9, 2025
Gayle Markovitz and Beatrice Di Caro
July 9, 2025
Ajay Kumar, Neha Thakur and Siddharth Sharma
July 8, 2025