Here’s how we teach machines to be fair
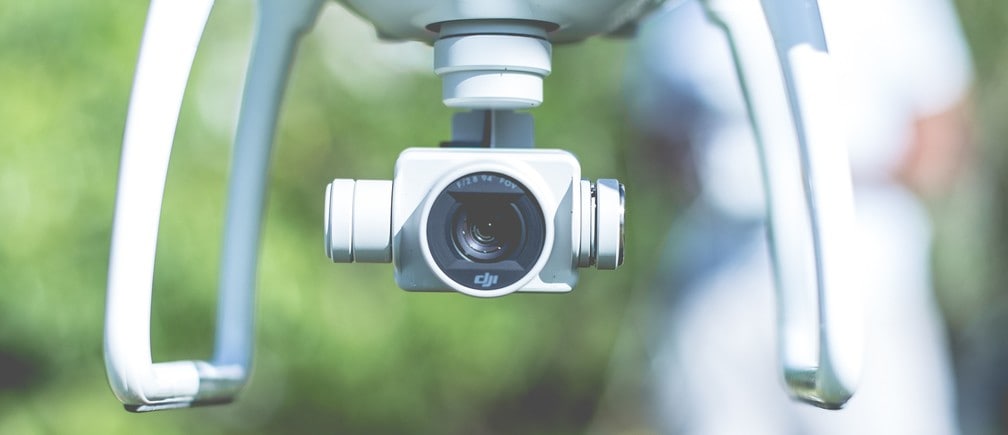
Evidence has shown that AI can lead to systematic bias. As its creators, we have a responsibility to put controls in place
Image: William Stitt/Unsplash
Stay up to date:
Emerging Technologies
The opportunities that artificial intelligence (AI) can unlock for our world – from discovering cures to diseases that kill millions each year to significantly cutting carbon emissions – are expanding every day. This includes a subset of AI called machine learning, which leverages the ability of machines to learn from vast quantities of data and use those lessons to make predictions. Machine learning (ML) is already enabling pathways to financial inclusion, citizen engagement, more affordable healthcare and many more vital systems and services. ML systems might highlight a post in your Facebook newsfeed based on your online activity, or select applicants in a hiring process. ML is one of the most powerful tools humanity has created – and it is more important than ever that we learn how to harness its power for good.
Public attention often focuses either on the existential threats artificial superintelligence poses to humanity (“the robots are coming to kill us”), or the opposite, salvation narrative (“AI will solve all our problems”). But there is a more immediate and less visible risk when machines make decisions: the potential reinforcement of systemic bias and discrimination. ML technologies are already making life-altering decisions for humans on a daily basis. As Jim Dwyer wrote in the New York Times: “Algorithms can decide where kids go to school, how often garbage is picked up, which police precincts get the most officers, where building code inspections should be targeted, and even what metrics are used to rate a teacher.”
As we empower machines to make critical decisions about who can access vital opportunities, we need to prevent discriminatory outcomes. After all, machine learning is only a tool. The responsibility falls on people use it wisely – especially the people leading the way in its advancement, from corporate leaders down to system engineers. In other words, we need to design and use ML applications in a way that not only improves business efficiency but also promotes and protects human rights. Using technology to automate decisions isn’t a new practice. But the nature of ML technology – its ubiquitousness, complexity, exclusiveness and opaqueness – can amplify longstanding problems related to unequal access to opportunities. Not only can discriminatory outcomes in machine learning undermine human rights, they can also lead to the erosion of public trust in the companies using the technology. We must address these risks by evaluating the ways discrimination can enter ML systems, and then getting these systems to “learn” not to discriminate.
Most of the stories we’ve heard about discrimination in machine learning come out of the United States and Europe. Events like a Google photo mechanism that mistakenly labelled an image of two black friends as gorillas, and predictive policing tools that have been shown to amplify racial bias, have received extensive and important media coverage. In many parts of the world, particularly in middle- and low-income countries, using ML to make decisions without taking adequate precautions to prevent discrimination is likely to have far-reaching, long-lasting and potentially irreversible consequences. Take, for instance, any one of the following examples:
• In Indonesia, economic development has unfolded unequally across geographical (and, subsequently, ethnic) lines. While access to higher education is relatively uniform across the country, the top 10 universities are all on the island of Java, and a large majority of the students who attend those universities are from Java. As firms hiring in white-collar sectors train ML systems to screen applicants based on factors like educational attainment status, they may systematically exclude those from poorer islands such as Papua.
• There are now ways for insurance companies to predict an individual’s future health risks. Mexico is among the countries where, for most, quality healthcare is available only through private insurance. At least two private multinational insurance companies operating in Mexico are now using ML to maximize their efficiency and profitability, with potential implications for the human right to fair access to adequate healthcare. Imagine a scenario in which insurance companies use ML to mine data such as shopping history to recognize patterns associated with high-risk customers, and charge them more: the poorest and sickest would be least able to afford access to health services.
• While few details are publicly available, reports suggest that China is creating a model to score its citizens by analyzing a wide range of data, from banking, tax, professional and performance records to smartphones, e-commerce and social media information. The Washington Post described this as an attempt “to use the data to enforce a moral authority as designed by the Communist party”. What will it mean, in future, if governments act on scores computed using data that is incomplete or historically biased, using models not built for fairness?
These scenarios tell us that, while machine learning can hugely benefit this world, there are also important risks to consider. We need to look closely at the ways discrimination can creep into ML systems, and what companies can do to prevent this.
If, as Klaus Schwab argues in his book, The Fourth Industrial Revolution, we want to work together to “shape a future that works for all by putting people first, empowering them and constantly reminding ourselves that all of these new technologies are first and foremost tools made by people for people”, we need to design and use machine learning to prevent and not deepen discrimination.
How to Prevent Discriminatory Outcomes in Machine Learning. Download the full report here.
Don't miss any update on this topic
Create a free account and access your personalized content collection with our latest publications and analyses.
License and Republishing
World Economic Forum articles may be republished in accordance with the Creative Commons Attribution-NonCommercial-NoDerivatives 4.0 International Public License, and in accordance with our Terms of Use.
The views expressed in this article are those of the author alone and not the World Economic Forum.
Forum Stories newsletter
Bringing you weekly curated insights and analysis on the global issues that matter.
More on Emerging TechnologiesSee all
Ayed Abdulhadi Al-Ruwaili
July 8, 2025
Linda Lacina and Kate Whiting
July 8, 2025
Benedict Singleton and Marta Ferreira de Sá
July 8, 2025
Kelly Ommundsen
July 4, 2025
Michael Donatti and Laura Fisher
July 3, 2025
Benjamin Hertz-Shargel
July 1, 2025