A new AI managed to predict a scientific discovery before it happened
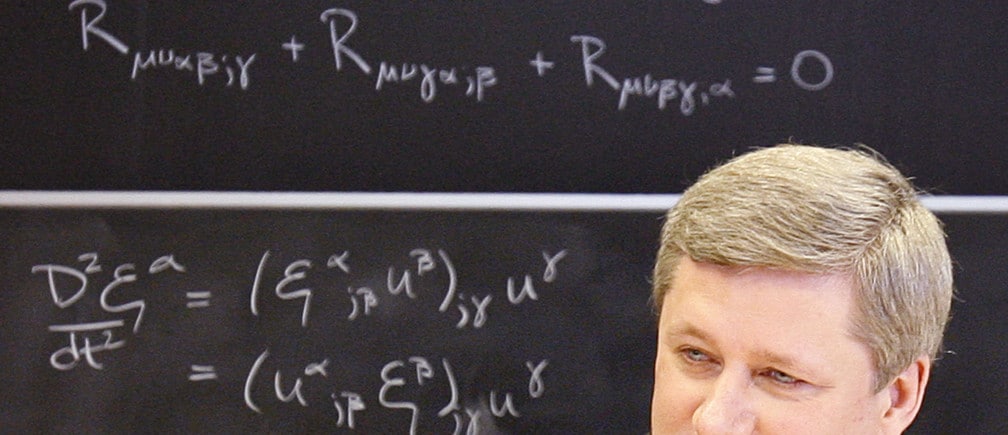
'For example, the AI managed to identify a substance known as CsAgGa2Se4as as a thermoelectric material, which scientists only discovered in 2012.' Image: REUTERS/J.P. Moczulski (CANADA) - GM1DVGYIALAA
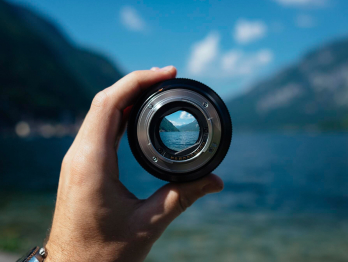
Get involved with our crowdsourced digital platform to deliver impact at scale
Stay up to date:
Artificial Intelligence
“Can machines think?”, asked the famous mathematician, code breaker and computer scientist Alan Turing almost 70 years ago. Today, some experts have no doubt that Artificial Intelligence (AI) will soon be able to develop the kind of general intelligence that humans have. But others argue that machines will never measure up. Although AI can already outperform humans on certain tasks – just like calculators – they can’t be taught human creativity.
After all, our ingenuity, which is sometimes driven by passion and intuition rather than logic and evidence, has enabled us to make spectacular discoveries – ranging from vaccines to fundamental particles. Surely an AI won’t ever be able to compete? Well, it turns out they might. A paper recently published in Nature reports that an AI has now managed to predict future scientific discoveries by simply extracting meaningful data from research publications.
Language has a deep connection with thinking, and it has shaped human societies, relationships and, ultimately, intelligence. Therefore, it is not surprising that the holy grail of AI research is the full understanding of human language in all its nuances. Natural Language Processing (NLP), which is part of a much larger umbrella called machine learning, aims to assess, extract and evaluate information from textual data.
Children learn by interacting with the surrounding world via trial and error. Learning how to ride a bicycle often involves a few bumps and falls. In other words, we make mistakes and we learn from them. This is precisely the way machine learning operates, sometimes with some extra “educational” input (supervised machine learning).
For example, an AI can learn to recognise objects in images by building up a picture of an object from many individual examples. Here, a human must show it images containing the object or not. The computer then makes a guess as to whether it does, and adjusts its statistical model according to the accuracy of the guess, as judged by the human. However we can also leave the computer program to do all the relevant learning by itself (unsupervised machine learning). Here, AI automatically starts being able to detect patterns in data. In either case, a computer program needs to find a solution by evaluating how wrong it is, and then try to adjust it to minimise such error.
Suppose we want to understand some properties related to a specific material. The obvious step is to search for information from books, web pages and any other appropriate resources. However, this is time consuming, as it may involve hours of web searching, reading articles and specialised literature. NLP can, however, help us. Via sophisticated methods and techniques, computer programs can identify concepts, mutual relationships, general topics and specific properties from large textual datasets.
In the new study, an AI learned to retrieve information from scientific literature via unsupervised learning. This has remarkable implications. So far, most of the existing automated NLP-based methods are supervised, requiring input from humans. Despite being an improvement compared to a purely manual approach, this is still a labour intensive job.
However, in the new study, the researchers created a system that could accurately identify and extract information independently. It used sophisticated techniques based on statistical and geometrical properties of data to identify chemical names, concepts and structures. This was based on about 1.5m abstracts of scientific papers on material science.
A machine learning program then classified words in the data based on specific features such as “elements”, “energetics” and “binders”. For example, “heat” was classified as part of “energetics”, and “gas” as “elements”. This helped connect certain compounds with types of magnetism and similarity with other materials among other things, providing an insight on how the words were connected with no human intervention required.
Scientific discoveries
This method could capture complex relationships and identify different layers of information, which would be virtually impossible to carry out by humans. It provided insights well in advance compared to what scientists can predict at the moment. In fact, the AI could recommend materials for functional applications several years before their actual discovery. There were five such predictions, all based on papers published before the year 2009. For example, the AI managed to identify a substance known as CsAgGa2Se4as as a thermoelectric material, which scientists only discovered in 2012. So if the AI had been around in 2009, it could have speeded up the discovery.
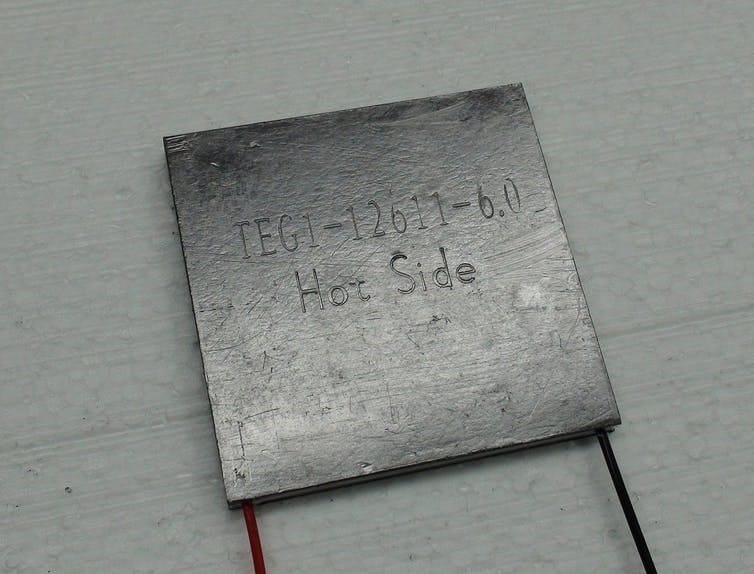
It made the prediction by connecting the compound with words such as “chalcogenide” (material containing “chalcogen elements” such as sulfur or selenium), “optoelectronic” (electronic devices that source, detect and control light) and “photovoltaic applications”. Many thermoelectric materials share such properties, and the AI was quick to show that.
This suggests that latent knowledge regarding future discoveries is to a large extent embedded in past publications. AI systems are becoming more and more independent. And there is nothing to fear. They can help us enormously to navigate through the huge amount of data and information, which is being continuously created by human activities. Despite concerns related to privacy and security, AI is changing our societies. I believe it will lead us to make better decisions, improve our daily lives and ultimately make us smarter.
Don't miss any update on this topic
Create a free account and access your personalized content collection with our latest publications and analyses.
License and Republishing
World Economic Forum articles may be republished in accordance with the Creative Commons Attribution-NonCommercial-NoDerivatives 4.0 International Public License, and in accordance with our Terms of Use.
The views expressed in this article are those of the author alone and not the World Economic Forum.
Related topics:
The Agenda Weekly
A weekly update of the most important issues driving the global agenda
You can unsubscribe at any time using the link in our emails. For more details, review our privacy policy.
More on Emerging TechnologiesSee all
Ana Kreacic and Michael Zeltkevic
May 7, 2024
Robin Pomeroy and Linda Lacina
April 29, 2024
Maroun Kairouz
April 29, 2024
Tanya Milberg
April 28, 2024