The promises and perils of AI - Stuart Russell on Radio Davos
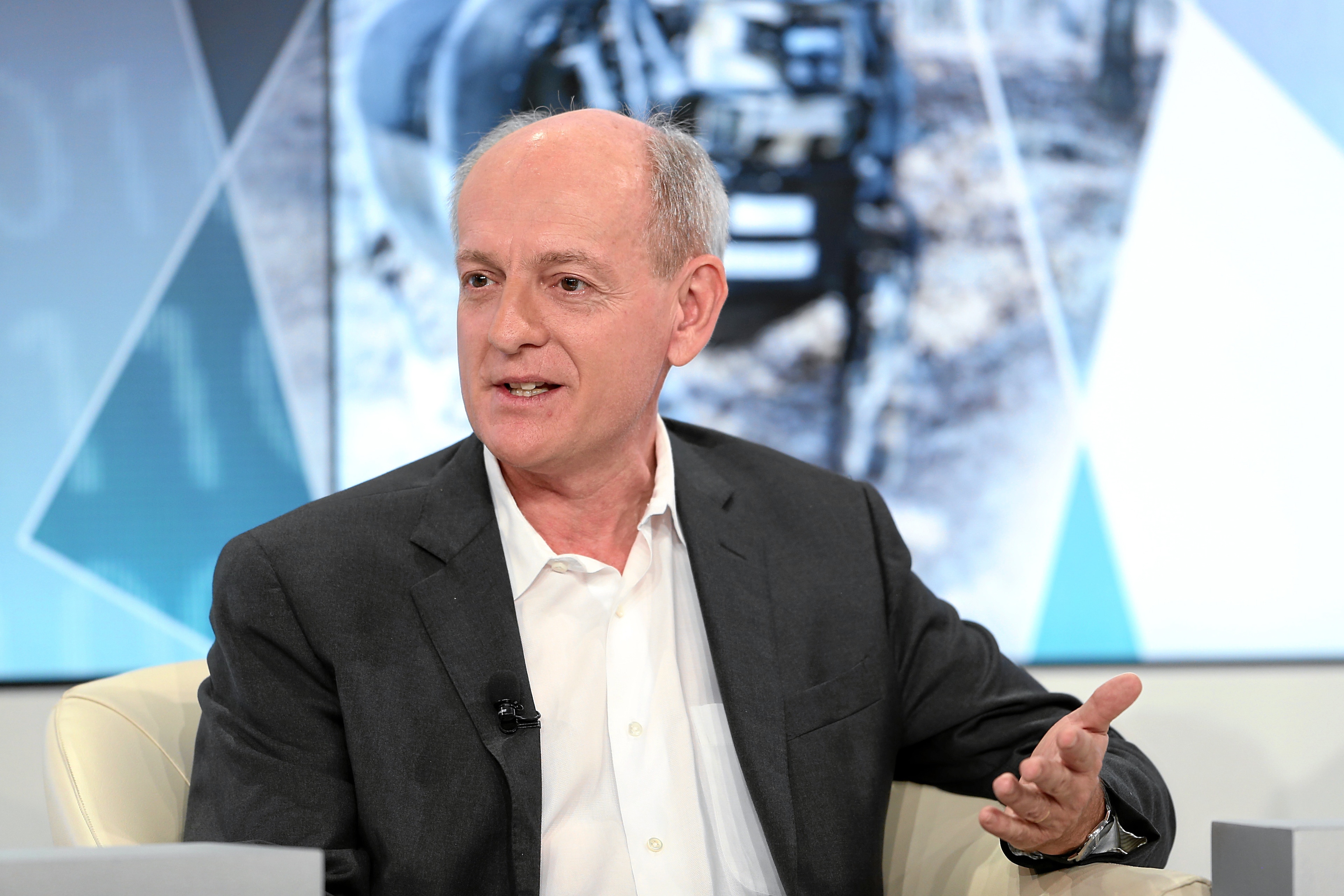
Stuart Russell says we need 'human compatible' AI
Image: swiss-image.ch/Photo Moritz Hager
Stay up to date:
Artificial Intelligence
Accept our marketing cookies to access this content.
These cookies are currently disabled in your browser.
- AI - artificial intelligence - is transforming every aspect of our lives.
- Professor Stuart Russell says we need to make AI 'human-compatible'.
- We must prepare for a world where machines replace humans in most jobs.
- Social media AI changes people to make them click more, Russell says.
- We've given algorithms 'a free pass for far too long', he says.
- Episode page: https://www.weforum.org/podcasts/radio-davos/episodes/ai-stuart-russell
Six out of 10 of people around the world expect artificial intelligence to profoundly change their lives in the next three to five years, according to a new Ipsos survey for the World Economic Forum - which polled almost 20,000 people in 28 countries.
A majority said products and services that use AI already make their lives easier in areas such as education, entertainment, transport, shopping, safety, the environment and food. But just half say they trust companies that use AI as much as they trust other companies.
But what exactly is artificial intelligence? How can it solve some of humanity's biggest problems? And what threats does AI itself pose to humanity?
Kay Firth-Butterfield, head of artificial intelligence and machine learning at the World Economic Forum's Centre for the Fourth Industrial Revolution, joined Radio Davos host Robin Pomeroy to explore these questions with Stuart Russell, one of the world's foremost experts on AI.
AI reports and audio mentioned in this podcast:
Transcript: The promises and perils of AI - Stuart Russell on Radio Davos
Kay Firth-Butterfield: It's my pleasure to introduce Stuart, who has written two books on an artificial intelligence, Human Compatible: Artificial Intelligence and the Problem of Control. But perhaps the one that you referred to, saying that he had 'literally written the book on artificial intelligence', that is Artificial Intelligence: A Modern Approach - and that's the book from which most students around the world learn AI. Stuart and I first met in 2014 at a lecture that he gave in the UK about his concerns around lethal autonomous weapons. And whilst we're not going to talk about that today, he's been working tirelessly at the UN for a ban on such weapons. Stuart's worked extensively with us at the World Economic Forum. In 2016, he became co-chair of the World Economic Forum's Global AI Council on AI and Robotics. And then in 2018, he joined our Global AI Council. As a member of that Council, he galvanised us into thinking about how we could achieve positive futures with AI, by planning and developing policies now to chart a course to that future.
Robin Pomeroy: Stuart, you're on the screen with us on Zoom. Very nice to meet you.
Stuart Russell: Thank you very much for having me. It's really nice to join you and Kay.
Robin: Where in the world are you at the moment?
Stuart: I am in Berkeley, California.
Robin: Where you're a professor. I've been listening to your lectures on BBC Radio 4 and the World Service, the Reith Lectures. So I feel like I'm an expert in it now, as I wasn't a couple of weeks ago. Let's start right at the very beginning, though. For someone who only has a vague idea of what artificial intelligence is - we all know what computers are, we use apps. How much of that is artificial intelligence? And where is it going to take us in the future beyond what we already have?
Stuart: It's actually surprisingly difficult to draw a hard and fast line and say, Well, this this piece of software is AI and that piece of software isn't AI. Because within the field, when we think about AI, the object that we discuss is something we call an 'agent', which means something that acts on the basis of whatever it has perceived. And the perceptions could be through a camera or through a keyboard. The actions could be displaying things on a screen or turning the steering wheel of a self-driving car or firing a shell from a tank, or whatever it might be. And the goal of AI is to make sure that the actions that come out are actually the right ones, meaning the ones that will actually achieve the objectives that we've set for the agent. And this maps onto a concept that's been around for a long time in economics and philosophy, called the 'rational agent' - so the agent whose actions can be expected to achieve its objectives.
And so that's what we try to do. And they can be very, very simple. A thermostat is an agent. It has perception - just measures the temperature. It has actions - switch on or off the heater. And it sort of has two very, very simple rules: If it's too hot, turn it off. If it's too cold, turn it on. Is that AI? Well, actually, it doesn't really matter whether you want to call that AI or not. So there's no hard and fast dividing line like, well, if it's got 17 rules then it's AI, if it's only got 16, then it's not AI. That wouldn't make sense. So we just think of it as as a continuum, from extremely simple agents to extremely complex agents like humans.
AI systems now are all over the place in the economy - search engines are AI systems. They're actually not just keyword look-up systems any more - they are trying to understand your query. About a third of all the queries going into search engines are actually answered by knowledge bases, not by just giving you web pages where you can find the answer. They actually tell you the answer because they have a lot of knowledge in machine readable form.
Your smart speakers, the digital assistants on your phone - these are all AI systems. Machine translation - which I use a lot because I have to pay taxes in France - it does a great job of translating impenetrable French tax legislation into impenetrable English tax legislation. So it doesn't really help me very much, but it's a very good translation. And then the self-driving car, I think you would say that's a pretty canonical application of AI that stresses many things: the ability to perceive, to understand the situation and to make complex decisions that actually have to take into account risk and the many possible eventualities that can arise as we drive around. And then, of course, at the very high end are human beings.
Robin: At some point in the future, machines, AI, will be able to do everything a human can do, but better. Is that is that the thing we're moving towards?
Stuart: Yes. This has always been the goal - what I call 'general purpose AI'. There are other names for it: human-level, AI, superintelligent AI, artificial general intelligence. But I settled on 'general purpose AI' because it's a little bit less threatening than 'superintelligent AI'. And, as you say, it means AI systems that for any task that human beings can do with their intellects, the AI system will be able to, if not, do it already, to very quickly learn how to do it and do it as well as or better than humans. And I think most people understand that once you reach a human level on any particular task, it's not that hard then to go beyond the human level. Because machines have such massive advantages in computation, speed in bandwidth, you know, the ability to store and retrieve stuff from memory at vast rates that humans human brains can't possibly match.
Robin: Kay, I'm going to hand it over to you and I'm going to take a back seat. I'll be your co-host if you like. I'm bursting with questions as well, so I'll annoyingly cut in. But basically for the rest of this interview, all yours.
Kay: Thank you. You talked, Stuart, a little bit about some of the examples of AI that we're encountering all the time. But one of the ways that AI is being used every day by human beings, from the youngest to the oldest, is in social media. We hear a great deal about radicalisation through social media. Indeed, at a recent conference I attended, Cédric O, the IT minister from France, described AI as the biggest threat to democracy existing at the moment. I wonder whether you could actually explain for our listeners how the current use of AI drives that polarisation of ideas.
Stuart: So I think this is an incredibly important question. The problem with answering your question is that we actually don't know the answer because the facts are hidden away in the vaults of the social media companies. And those facts are basically trillions of events per week - trillions! Because we have billions of people engaging with social media hundreds of times a day and every one of those engagements - clicking, swiping, dismissing, liking, disliking thumbs up, thumbs down - you name it - all of that data is inaccessible, even, for example, to Facebook's oversight board, which is supposed to be actually keeping track of this, that's why they made it. But that board doesn't have access to the internal data.
You maximise click-through by sending people a chain of content that turns them into somebody else who is more susceptible to clicking on whatever content you're going to send them in future.
”So there is some anecdotal evidence. There are some data sets on which we are able to do some analysis that's suggestive, but I would say it's not conclusive. However, if you think about the way the algorithms work, what they're trying to do is maximise click-through. They want you to click on things, engage with content or spend time on the platform, which is a slightly different metric, but basically the same thing.
And you might say, Well, OK, the only way to get people to click on things is to send them things they're interested in. So what's wrong with that? But that's not the answer. That's not the way you maximise click-through. The way you maximise click-through is actually to send people a chain of content that turns them into somebody else who is more susceptible to clicking on whatever content you're going to be able to send them in future.
So the algorithms have, at least according to the mathematical models that we built, the algorithms have learnt to manipulate people to change them so that in future they're more susceptible and they can be monetised at a higher rate.
The algorithms don't care what opinions you have, they just care that you're susceptible to stuff that they send. But of course, people do care.
”Now, at the same time, of course, there's a massive human-driven industry that sprung up to feed this whole process: the click-bait industry, the disinformation industry. So people have hijacked the ability of the algorithms to very rapidly change people because it's hundreds of interactions a day, everyone has a little nudge. But if you nudge somebody hundreds of times a day for days on end, you can move them a long way in terms of their beliefs, their preferences, their opinions. The algorithms don't care what opinions you have, they just care that you're susceptible to stuff that they send. But of course, people do care, and they hijacked the process to take advantage of it and create the polarisation that suits them for their purposes. And, you know, I think it's essential that we actually get more visibility. AI researchers want it because we want to understand this and see if we can actually fix it. Governments want this because they're really afraid that their whole social structure is disintegrating or that they're being undermined by other countries who don't have their best interests at heart.
Robin: Stuart, do we know whether that's a kind of a by-product of the algorithms or whether a human at some point has built that into the algorithms, this polarisation?
Stuart: I think it's a by-product. I'm willing to give the social media platforms some benefit of the doubt - that they they didn't intend this. But one of the things that we know is that when algorithms work well in the sense that they generate lots of revenue and profit for the company, that creates a lot of pressure not to change the algorithm. And so whether it's conscious or unconscious, the algorithms are in some sense protected by this multinational superstructure that's generating, enjoying the billions of dollars that are generated and wants to protect that revenue stream.
Kay: Stuart, you used the word 'manipulating' us, but you also said the algorithms don't care. Can you just explain what what one of these algorithms would look like? And presumably it doesn't care because it doesn't know anything about human beings?
[AI] doesn't know that human beings exist at all. From the algorithm's point of view, each person is simply a click history.
”Stuart: That's right, it doesn't know that human beings exist at all. From the algorithm's point of view, each person is simply a click history. So what was presented and did you or did you not click on it? And so let's say the last 100 or the last 1,000 such interactions - that's you. And then the algorithm learns, OK, how do I take those thousand interactions and choose the next thing to send? We call that a 'policy', that decides what's the next thing to send, given the history of interactions, and the policy is learned over time in order to maximise the long-term rate of clicking. So it's not just trying to choose the next best thing that you're going to click on. It's also, just because of the way the algorithm is constructed, it's choosing the thing that is going to yield the best results in the long term.
Just as if I want to get to San Francisco from here, right? I make a long-term plan and then I start executing the plan, which which involves getting up out of my chair and then doing some other things, right? And so the algorithm is sort of embarking on this journey, and it's learned how to get to these destinations where people are more predictable and it's predictability that the algorithm cares about in terms of maximising revenue. The algorithms wouldn't be conscious anyway, but it's not deliberate in the sense that it has an explicit objective to radicalise people or cause them to become terrorists or or anything like that. Now, future algorithms that actually know much more, that know that people do exist and that we have minds and that we have a particular kind of psychology and different susceptibilities, could be much more effective. And I think this is one of the things that feels a little bit like a paradox at first - that the better the AI, the worse the outcome.
Kay: You talked about learning - the algorithms learning - and that's something that I think quite a lot of people don't really understand - because we use software, we've been using software for a long time, but this is slightly different.
Stuart: Actually, much of the software that that we have been using was created by a learning process. For example, speech recognition systems. There isn't someone typing in a rule for how do you distinguish between 'cat' and 'cut'? We just give the algorithm lots of examples of 'cat' and lots of examples of 'cut', and then the algorithm learns the distinguishing rule by tweaking the parameters of some kind of - think of it as a big circuit with lots of tuneable weights or connection strengths in the circuit. And then as you tune all those weights in the circuit, the output of the circuit will change and it'll start becoming better at distinguishing between 'cat' and 'cut', and you're trying to tune those weights to agree with the training data - the labelled examples, as we say - the 'cats' and the 'cuts'. And as that process of tuning all the weights proceeds, eventually it will give you perfect or near-perfect performance on the training data. And then you hope that when a new example of 'cat' or 'cut' comes along, that it succeeds in classifying it correctly. And so that's how we train speech recognition systems, and that's been true for decades. We're a little bit better at it now so our speech recognition systems are more accurate, much more robust - it'll be able to understand what you're saying even when you're driving a car and talking on a crackly cellphone line. It's good enough now to understand that speech.
When you buy speech recognition software, for example, dictation software, there's often a what we might call a post-purchase learning phase where it's already pretty good, but, by training on your voice specifically, it can become even better. So it will give you a few sentences to read out and then that additional data means that it can be even more accurate on you.
And so you can think of what's going on in the social media algorithms as like that - a sort of post-purchase customisation - it's learning about you. So, initially, it can recommend articles that are of interest to the general population, which seems to be Kim Kardashian, as far as I can tell. But then after interacting with you for a while, it will learn: actually, no, I'd rather get the cricket scores or something like that.
There is no equivalent of city planning for AI systems.
”Kay: Is it the same for facial recognition? Because we hear a lot about facial recognition and facial recognition perhaps making mistakes and people of colour. Is that because the data's wrong?
Stuart: Usually, it's not because the data is wrong. In that case, it's because the data has many fewer examples of particular types of people. And so when you have few examples, the accuracy on that subset will be worse. This is a very controversial question, and it's actually quite hard to to get agreement among the various different parties to the debate about whether one can eliminate these disparities in recognition rates by actually making more representative datasets. And of course, there isn't one perfectly representative dataset. Because it would depend on what's a perfectly representative dataset if you're in Namibia or Japan or Iceland or Mongolia. It wouldn't necessarily be appropriate to use exactly the same data for all those four settings. And so these these questions become not so much technical, but 'socio-technical'. What matters is what happens when you deploy the AI system in a particular context and it operates for a while. There are many things that go on. For example, people might start avoiding places where there are cameras, but maybe only one type of person avoids the places where there are cameras and the other people don't mind. And so now you created a new bias in the collection of data, and that bias is really hard to understand because you can't predict who's going to avoid the area with the cameras. And so understanding that, we're nowhere near having a real engineering discipline or scientific approach to understanding the sort of socio-technical embedding of AI systems, what effect they can have, what effect society has on them and their operation. And then, are we all better off as a result? Or are we all worse off as a result? Early anecdotes suggest that there's all kinds of weird ways that things go wrong that you just don't expect because we're not used to thinking about this.
Now, if you're in city planning, they have learnt over centuries that weird things happen. When you broaden a road you think that's going to improve traffic flow, but it turns out that sometimes making bigger roads makes the traffic flow worse. Same thing: should you add a bridge across the river? They've learnt to actually think through the consequences - pedestrianising a street, you might, oh, that's good, but then it just moves the traffic somewhere else and things get worse in the next neighbourhood. So there's all kinds of complicated things, and we're just beginning to explore these and we don't really yet have a proper discipline. There is no equivalent of city planning for AI systems and their socio- technical embeddings.
Accept our marketing cookies to access this content.
These cookies are currently disabled in your browser.
Kay: Back in 2019, I think it was, you came to me with a suggestion, and that was to truly optimise the benefits for humans of AI and, in particular, general purpose AI, which you spoke to Robin about earlier. We need to rethink the political and social systems we use. We were getting to lock people in a room and those people were specifically going to be economists and sci-fi writers. We never did that because we got COVID. But we had such fantastically interesting workshops, and I wonder whether you could tell us a little bit about why you thought that was important and the sort of ideas that came out of it.
Stuart: I just want to reassure the viewers that we didn't literally plan to lock people into a room, but it was a metaphorical sense. The concern, or the question, was: what happens when general purpose AI hits the real economy? How do things change? And can we adapt to that without having a huge amount of dislocation? Because, you know, this is a very old point. Even, amazingly, Aristotle actually has a passage where he says: Look, if we had fully automated weaving machines and fully automated plectrums that could pluck the lyre and produce music without any humans, then we wouldn't need any workers. It's a pretty amazing thing for 350 BC.
That that idea, Keynes called it 'technological unemployment' in 1930, is very obvious to people, right? They think: Yeah, of course, if the machine does the work, then I'm going to be unemployed. And the Luddites worried about that. And for a long time, economists actually thought that they had a mathematical proof that technological unemployment was impossible. But if you think about it, if technology could make a twin of every person on Earth and the twin was more cheerful and less hungover and willing to work for nothing, well how many of us would still have our jobs? I think the answer is zero. So there's something wrong with the economists' mathematical theorem.
Over the last decade or so, opinion in economics has really shifted. And it was, in fact, the first Davos meeting that I ever went to, in 2015. There was a dinner supposedly to discuss the 'new digital economy' . But the economists who got up - there were several Nobel prize winners there, other very distinguished economists - and they got up one by one and said: You know, actually, I don't want to talk about the digital economy. I want to talk about AI and technological unemployment, and this is the biggest problem we face in the world, at least from the economic point of view. Because as far as they could see it, as general purpose AI became more and more of a real thing - right now, we're not very close to it - but as we move there, we'll see AI systems capable of carrying out more and more of the tasks that humans do at work.
Accept our marketing cookies to access this content.
These cookies are currently disabled in your browser.
So just to give you one example, if you think about the warehouses that Amazon and other companies are currently operating for e-commerce, they are half-automated. The way it works is that instead of having an old warehouse where you've got tons of stuff piled up all over the place and then the humans go and rummage around and then bring it back and send it off, there's a robot who goes and gets the shelving unit that contains the thing that you need and brings it to the human worker. So the human worker stands in one place and these robots are going in collecting shelving units of stuff and bringing them. But the human has to pick the object out of the bin or off the shelf, because that's still too difficult.
It's all very well saying: Oh, we'll just retrain everyone to be data scientists. But we don't need 2.5 billion data scientists.
”And there's, let's say, three or four million people with that job in the world. But at the same time Amazon was running a competition for bin-picking: could you make a robot that is accurate enough to be able to pick pretty much any object - and there's a very wide variety of objects that you can buy - pretty much any object from shelves, bins, et cetera, do it accurately and then send it off to the dispatch unit. That would, at a stroke, eliminate three or four million jobs. And the system is already set up to do that. So it wouldn't wouldn't require then rejigging everything, you'd really just be putting a robot in the place where the human was.
People worry about self-driving cars. As that becomes a reality, then a self-driving taxi is going to be maybe a quarter of the price of a regular taxi. And so you can see what would happen, right? There's about, I think, 25 million people, formal or informal taxi drivers in the world. So that's a somewhat bigger impact. And then, of course, this continues with each new capability. More tasks are automated. And can we keep up with that rate of change in terms of finding other things that people will do and then training them to do those new things that they may not know how to do? So it's all very well saying: Oh, we'll just retrain everyone to be data scientists. But, number one, we don't need 2.5 billion data scientists. Not even sure we need 2.5 million data scientists. So it's a drop in the bucket.
Accept our marketing cookies to access this content.
These cookies are currently disabled in your browser.
But other things - yeah, we need more people who can do geriatric care. But it's not that easy to take someone who's been a truck driver for 25 years and we train them to be in the geriatric care industry. Tutoring of young children. There's many other things that are unmet needs in our world. I think that's obvious to almost everyone - there are unmet needs - machines may be able to fulfil some of those needs, but humans can only meet them if they are trained and have the knowledge and aptitude and even inclination to do those kinds of jobs.
When we look in science fiction, there are models for worlds that seem quite desirable. But as economies, they don't hang together.
”So the question we were asking is: OK, if this process continues -- general purpose AI is doing pretty much everything we currently call work. What is the world going to look like or what would a world look like that you would want your children to go into, to live in? And when we look in science fiction, there are models for worlds that seem quite desirable. But as economies, they don't hang together. So the economists say: the incentives wouldn't work in that world - these people would stop doing that and those people would do this instead, and it all just wouldn't be stable. And the economists, they don't really invent things, right? They just talk about, well, we could raise this tax or we could, you know, decrease this interest rate. The economists at Davos that were talking all said: perhaps we could have private unemployment insurance. Well, yeh, right - that really solves the problem! So I wanted to put these two groups together, so you could get imagination tempered by real economic understanding.
Robin: I'm curious to know who were optimists and who were the pessimists between the economists and the science fiction writers. Science ficition, I imagine they love a bit of dystopia and things going horribly wrong. But I wonder whether the flip side of that is actually they've got a more optimistic outlook than the economists who are embedded in the real world where things really are going wrong all the time. Did you notice a trend either way?
Stuart: The science fiction writers, as you say, they're fond of dystopias, but the economists mostly are pessimistic, at least certainly the ones at the dinner, and I've been interacting with many during these workshops. I think there's still a view of many economists that - there are compensating effects where it's not as simple as saying, if the machine does Job X, then the person isn't doing Job X, and so the person is unemployed. There are these compensating effects. So if a machine is doing something more cheaply, more efficiently, more productively, then that increases total wealth, which then increases demand for all the other jobs in the economy. And so you get this sort of recycling of labour from areas that are becoming automated to areas that are still not automated. But if you automate everything, then this is the argument about the twins, right? It's like making a twin of everyone who's willing to work for nothing. And so you have to think, well are there areas where we aren't going to be automating, either because we don't want to or because humans are just intrinsically better. So this is one, I think, optimistic view, and I think you could argue that Keynes had this view. He called it 'perfecting the art of life'. We will be faced with man's permanent problem, which is how to live agreeably and wisely and well. And those people who cultivate better the art of life will be much more successful in this future.
Accept our marketing cookies to access this content.
These cookies are currently disabled in your browser.
And so cultivating the art of life is something that humans understand. We understand what life is, and we can do that for each other because we are so similar. We have the same nervous systems. I often use the example of hitting your thumb with a hammer. If you've ever done that, then you know what it's like and you can empathise with someone else who does it.
There's this intrinsic advantage that we have for knowing what it's like to be jilted by the love of your life ... so we have this extra comparative advantage over machines.
”You don't need a Ph.D. in neuroscience to know what it's like to hit your thumb with a hammer, and if you haven't done it well, you can just do it. And now you know what it's like, right? So there's this intrinsic advantage that we have for knowing what it's like, knowing what it's like to be jilted by the love of your life, knowing what it's like to lose a parent, knowing what it's like to come bottom in your class at school and so on. So we have this extra comparative advantage over machines. That means that those kinds of professions - interpersonal professions - are likely to be ones that humans will have a real advantage. Actually more and more people, I think, will be moving into those areas. For some interpersonal professions like executive coach, those are relatively well-paid because they're providing services to very rich people and corporations. But others, like babysitting, are extremely poorly paid, even though supposedly we care enormously about our children more than we care about our CEO. But we pay someone $5 an hour and everything you can eat from the fridge to look after our children, whereas if we break a leg, we pay an orthopaedic surgeon $5,000 an hour and everything he can eat from the fridge to fix our broken leg. Why is that? Because he knows how to do it, and the babysitter doesn't really know how to do it. Some are, some are good and some are absolutely terrible. Like the babysitter taught me, or tried to teach me and my sister to smoke when we were seven and nine.
Robin: Someone's got to do it.
Stuart: Someone's got to do it! In order for this vision of the future to work, we need a completely different science base. We need a science base that's oriented towards the human sciences. How? How do you make someone else's life better? How do you educate an individual child with their individual personalities and traits and characteristics and everything so that they have, as Keynes called it, the ability to live wisely and agreeably and well? We know so little about that. And it's going to take a long time to shift our whole scientific research orientation and our education system to make this vision actually an economically viable one. If that's the destination, then we need to start preparing for that journey sooner rather than later. And so the idea of these workshops was to envision these possible destinations and then figure out what are the policy implications for the present.
Accept our marketing cookies to access this content.
These cookies are currently disabled in your browser.
Kay: And so these destinations, we're talking about something in the future. I know that this may be crystal ball gazing, but when might we expect general purpose AI, so that we can be prepared? You say we need to prepare now.
Stuart: I think this is a very difficult question to answer. And it's also it's not the case that it's all or nothing. The impact is going to be increasing. So with every advance in AI, it significantly expands the range of tasks that can be done. So, you know, we've been working on self-driving cars, and the first demonstrated freeway driving was 1987. Why has it taken so long? Well, because mainly the perceptual capabilities of the systems were inadequate, and some of that was just hardware. You just need massive amounts of hardware to process high-resolution, high-frame-rate video. And that problem has been largely solved. And so, you know, with visual perception now a whole range, not just self-driving cars, but you can start to think about robots that can work in agriculture, the robots that can do the part-picking in the warehouse, et cetera, et cetera. So, you know, just that one thing easily has the potential to impact 500 million jobs. And then as you get to language understanding, that could be another 500 million jobs. So each of these changes causes this big expansion.
Most experts say by the end of the century we're very, very likely to have general purpose AI. The median is something around 2045.
”And so these things will happen. The actual date of arrival of general purpose AI, you're not going to be able to pinpoint. It isn't a single day - 'Oh, today it arrived - yesterday we didn't have it'. I think most experts say by the end of the century we're very, very likely to have general purpose AI. The median is something around 2045, and that's not so long, it's less than 30 years from now. I'm a little more on the conservative side. I think the problem is harder than we think. But I liked what John McCarthy, who was one of the founders of AI, when he was asked this question, he said: Well, somewhere between five and 500 years. And we're going to need, I think, several Einsteins to make it happen.
Accept our marketing cookies to access this content.
These cookies are currently disabled in your browser.
Robin: On the bright side, if these machines are going to be so brilliant, will there come a day when we just say: Fix global hunger, fix climate change? And off they go and you set them six months, or whatever, a reasonable amount of time. And suddenly they've fixed climate change. In one of your Reith Lectures you actually broach the climate change subject. You actually reduce it to one area of climate change, the acidification of the oceans. And you envisage a scenario where a machine can fix the acidification of the oceans that's been caused by climate change. But there is a big 'but' there. Perhaps you can tell us what the problem is when you set at AI off to do a specific job?
When you ask a human to fetch you a cup of coffee, you don't mean this should be their life's mission and nothing else in the universe matters, even if they have to kill everybody else in Starbucks.
”Stuart: So there's a big difference between asking a human to do something and giving that as the objective to an AI system. When you ask a human to fetch you a cup of coffee, you don't mean this should be their life's mission and nothing else in the universe matters, even if they have to kill everybody else in Starbucks to get you the coffee before it closes. That's not what you mean. And of course, all the other things that we mutually care about, you know, they should factor into their behaviour as well.
If we build systems that know that they don't know what the objective is, then they start to exhibit these behaviours, like asking permission before getting rid of all the oxygen in the atmosphere.
”And the problem with the way we build AI systems now is we we give them a fixed objective. The algorithms require us to specify everything in the objective. And if you say: can we fix the acidification of the oceans? Yeah, you could have a catalytic reaction that does that extremely efficiently, but consumes a quarter of the oxygen in the atmosphere, which would apparently cause us to die fairly slowly and unpleasantly over over the course of several hours. So how do we avoid this problem? You might say, OK, well, just be more careful about specifying the objective, right? Don't forget the atmospheric oxygen. And then of course, it might produce a side-effect of the reaction in the ocean that poisons all the fish. OK, well, I meant don't kill the fish, either. And then, well, what about the seaweed, OK? Don't do anything that's going to cause all the seaweed to die - and on and on and on. Right? And the reason that we don't have to do that with humans is that humans often know that they don't know all the things that we care about. And so they are likely to come back. So if if you ask a human to get you a cup of coffee, and you happen to be in the hotel George V in Paris, where the coffee is, I think, 13 euros a cup, it's entirely reasonable to come back and say, 'Well, it's 13 euros. Are you sure? Or I could go next door and go get one for much less', right? That's because you might not know their price elasticity for coffee. You don't know whether they want to spend that much. And it's a perfectly normal thing for a person to do - to ask. I'm going to repaint your house - is it OK if I take off the drainpipes and then put them back? We don't think of this as a terribly sophisticated capability, but AI systems don't have it because the way we build them now, they have to know the full objective.
Control over the AI system comes from the machine's uncertainty about what the true objective is
”And in my book Human Compatible that Kay mentioned, the main point is if we build systems that know that they don't know what the objective is, then they start to exhibit these behaviours, like asking permission before getting rid of all the oxygen in the atmosphere. And they do that because that's a change to the world and the algorithm may not know is that something we prefer or disprefer. And so it has an incentive to ask because it wants to avoid doing anything that's dispreferred. So you get much more robust, controllable behaviour. And in the extreme case, if we want to switch the machine off, it actually wants to be switched off because it wants to avoid doing whatever it is that is upsetting us. It wants to avoid it. It doesn't know which thing it's doing that's upsetting us, but it wants to avoid that. So it wants us to switch it off if that's what we want. So in all these senses, control over the AI system comes from the machine's uncertainty about what the true objective is. And it's when you build machines that believe with certainty that they have the objective, that's when you get sort of psychopathic behaviour, and I think we see the same thing in humans.
Kay: And would that help with those basic algorithms that we were talking about - basic algorithms that are leading us down the journey of radicalisation? Or is it only applicable in general purpose AI ?
Stuart: No, it's applicable everywhere. We've actually been building algorithms that are designed along these lines. And you can actually set it up as a formal mathematical problem. We call it an 'assistance game' - 'game' in the sense of game theory, which means decision problems that involve more than one entity. So it involves the machine and the human actually coupled together by this uncertainty about the human objective. And you can solve those assistance games. You can mathematically derive algorithms that come up with a solution and you can look at the solution and: Gosh, yeah, it asked for permission, or, the human half of the solution actually wants to teach the machine because it wants to make sure the machine does understand human preferences so that it avoids making mistakes.
And so with social media, this is probably the hardest problem because it's not just that it's doing things we don't like, it's actually changing our preferences. And that's a sort of a failure mode, if you like, of of any AI system that's trying to satisfy human preferences - which sounds like a very reasonable thing to do. One way to satisfy them is to change them so that they're already satisfied. I think politicians are pretty good at doing this. And we don't want AI systems doing that. But it's sort of the wicked problem because it's not as if all the users of social media hate themselves, right? They don't. They're not sitting there saying: How dare you turn me into this raving neo-fascist, right? They believe that their newfound neo-fascism is actually the right thing, and they were just deluded beforehand. And so it gets to some of the most difficult of current problems in moral philosophy. How do you act on behalf of someone whose preferences are changing over time? Do you do you act on behalf of the present person or the future person? Which one? And there isn't a good answer to that question. And I think it points to gaps in our understanding of moral philosophy. So in that sense, what's happening in social media is really difficult to unravel.
Accept our marketing cookies to access this content.
These cookies are currently disabled in your browser.
But I think one one of the things that I would recommend is simply a change in mindset in the social media platforms. Rather than thinking, OK, how can we generate revenue, think, what do our users care about? What do they want the future to be like? What do they want themselves to be like? And if we don't know - and I think the answer is we don't know - I mean, we've got billions of users. They're all different. They all have different preferences. We don't know what those are - think about ways of having systems that are initially very uncertain about the true preferences of the user and try to learn more about those, but while respecting them. The most difficult part is, you can't say 'don't touch the user's preferences, under no circumstances are you allowed to change the user's preferences' because just reading the Financial Times changes your preferences. You become more informed. You learn about all sorts of different points of view and then you're a different person. And we want people to be different people over time. We don't want to remain newborn babies forever. But we don't have a good way of saying, well, this process of changing a person into a new person is good. We think of university education as good or global travel as good. Those usually make people better people, whereas, brainwashing is bad and joining a cult, what cults do to people is bad, and so on. But what's going on in social media is right at the place where we don't know how to answer these questions. So we really need some help from moral philosophers and other thinkers.
Robin: You quoted Keynes earlier saying that when the machines are doing all our work for us, human will be able to cultivate this - they'll live the fullest possible life in an age of plenty. But of course he was writing that before everyone was scrolling through social media in their down-time.
Stuart: There's an interesting story that E.M. Forster wrote. Forster usually wrote novels about British upper class society and and the decay of Victorian morals. But he wrote a science fiction story in 1909 called The Machine Stops which I highly recommend, where everyone is entirely machine-dependent. They use email, they suffer from email backlogs. They do a lot of video conferencing, or Zoom meetings. They have iPads.
Accept our marketing cookies to access this content.
These cookies are currently disabled in your browser.
Robin: How could E.M. Forster have written about iPads?
Stuart: Exactly. He called it a video disc, but, you know, it's exactly an iPad. And then, you know, people become obese for not getting any exercise because they're glued to their email on their screens all the time. And the story is really about the fact that that if you hand over the management of your civilisation to machines, you then lose the incentive to understand it yourself or to teach the next generation how to understand it.
Accept our marketing cookies to access this content.
These cookies are currently disabled in your browser.
And and you could see Wall-E actually as a modern version of The Machine Stops where everyone is enfeebled and infantilized by the machine because we lost the incentive to actually understand and run our own civilisation. And that hasn't been possible up to now, right? We put a lot of our civilisation into books, but the books can't run it for us. And so we always have to teach the next generation. And if you work it out, it's about a trillion person-years of teaching and learning in an unbroken chain that goes back tens of thousands of generations. And what happens if that chain breaks? And I think that's that's what the story is about. And that's something we have to understand ourselves as AI moves forward.
Robin: On the optimistic side, though, current generations have at their fingertips knowledge and wisdom that we just didn't have 30 years ago. So if I want to read the poetry of William Shakespeare, I don't need to go to the library or bookshop - it's right there in front of me - or hear the symphonies or learn how to play the piano. I envy people who are 30 years younger than me because they've got all this access to that knowledge if they choose not to be Wall-E.
Stuart: They've never heard of William Shakespeare or Mozart. They can tell you the names of all the characters in all the video games. But so, you know, I think this this comes back to the discussion we were having earlier about how we need to think about our education system. So even if you just believe Keynes's view, his rosy view of the future didn't involve an economy based on interpersonal services, but just people living happy lives and maybe a lot of voluntary interactions and a sort of non-economic system. But he still felt like we would need to understand how to educate people to live such a life successfully. And our current system doesn't do that, it's not about that. It's actually educating people to fulfil different sorts of economic functions, designed, some people argue, for the British civil service of the late Victorian period. And how you do that? We don't have a lot of experience with that, so we have to learn it.
Kay: You've talked a bit about the things that we need to do in order to control general purpose intelligence. And we talked about how they're applicable to social media today. Azimov had three principles of robotics, and I think you've got three principles of your own that you hope - and are testing - would work to ensure that all AI prioritises us humans.
Robin: Stuart, before you answer, could I just remind the listeners what the three laws are? I've just gone onto my AI - Wikipedia - to find out what they are. So this is from the science fiction writer Isaac Asimov. The first law: a robot may not injure a human being or through inaction allow a human being to come to harm. The second law: a robot must obey the orders given it by human beings except where such orders would conflict with the first law. The third law is: a robot must protect its own existence as long as such protection does not conflict with the first or second law. So, Stuart, what have you come up with along those lines?
Stuart: I have three principles sort of as a homage to Asimov. But Asimov's rules in the stories, these are laws that, in some sense, the algorithms in the robots are constantly consulting so they can decide what to do. And I think of the three principles that I give in the book as being guides for AI researchers and how you set up the mathematical problem that your algorithm is a solution to.
And so the the three principles: the first one is that the only objective for all machines is the satisfaction of human preferences. 'Preferences' is actually a term from economics. It doesn't just mean what kind of pizza do you like or who did you vote for. It really means what is your ranking over all possible futures for everything that matters. It's a very, very big, complicated, abstract thing, most of which you would never be able to explicate even if you tried, and some of which you literally don't know. Because I literally don't know whether I'm going to like durian fruit if I eat it. Some people absolutely love it, and some people find it absolutely disgusting. I don't know which kind of person I am, so I literally can't tell you, you know, do I like the future where I'm eating durian every day? So that's the first principle, right? We want the machines to be satisfying human preferences.
Second principle is that the machine does not know what those preferences are. So it has initial uncertainty about human preferences. And we already talked about the fact that this sort of humility is what enables us to retain control. It makes the machines in some sense deferential to human beings.
The third principle really just grounds what we mean by preferences in the first two principles, and it says that human behaviour is the source of evidence for human preferences. That can be unpacked a bit. Basically, the model is that humans have these preferences about the future, and that those preferences are what cause us to make the choices that we make. Behaviour means everything we do, everything we don't do - speaking, not speaking, sitting, reading your email while you're watching this lecture or this interview and so on. So with those principles, when we turn them into a mathematical problem, this is what we mean by the 'assistance game'.
You could say: 'guarantee no harm'. But a self-driving car that followed Asimov's first law would never leave the garage.
”And so there are there are significant differences from from Asimov's. I think some aspects of Asimov's principles are reflected because the the idea of not allowing a human to come to harm, I think you could translate that into 'satisfy human preferences'. Harm is sort of the opposite of preferences and so 'satisfying human preferences'. But the language of Asimov's principles actually reflects a mindset that was sort of pretty common up until the 50s and 60s, which was that uncertainty didn't really matter very much. So you could sort of say: guarantee no harm, right? But think about it: a self-driving car that followed Asimov's first law would never leave the garage because there is no way to guarantee safety on the freeway - just can't do it because someone else can always just side swipe you squish you. And so you have to take into account uncertainty about the world, about how the other agents are going to behave, the fact that your own senses don't give you complete and correct information about the state of the world. There's lots of uncertainty all over the place. You know, like, where is my car? Well, I can't see my car. I'm sitting in my house - it's down there somewhere, but I'm not sure it's still there. Someone might have just stolen it while we've been having this conversation. There's uncertainty about almost everything in the world, and you have to take account of that in decision making.
The third law says the robot needs to preserve its own existence as long as that doesn't conflict the first two laws. That's completely unnecessary in the new framework because if the robot is useful to humans, in other words, if it is helping at all to help us satisfy our preferences then that's the reason for it to stay alive. Otherwise, there is none. If it's completely useless to us or its continued existence is harmful to us then absolutely it should get rid of itself.
Accept our marketing cookies to access this content.
These cookies are currently disabled in your browser.
And if you watched the movie Interstellar, which is, from the AI point of view, one of the most accurate and reasonable depictions of AI, how it should work with us, one of the robots, TARS, just commits suicide because, I think its mass is somehow causing a problem with with the black hole, and so it's says, 'OK, I'm going off into the black hole so the humans can escape'. It's completely happy and the humans are really upset and say, 'No, no, no'. And that's because they they they probably were brought up on Asimov. They should realise that actually, no, this is entirely reasonable.
Kay: One of the things that I very much hope by having you come in to do this podcast with us is that everybody who's listening will end up to be much more informed about artificial intelligence because of so much that's incorrect about AI that we see in the media, etc.. And you've just given the prestigious BBC Reith Lectures and are reaching a lot of people through your work. And I know it's hard, but what would be the vital thing that you want our listeners to take away about artificial intelligence?
AI is a technology. It isn't intrinsically good or evil. That decision is up to us.
”Stuart: So as we know from business memos, there are always three three points I'd like to get across here. The first point is that AI is a technology. It isn't intrinsically good or evil. That decision is up to us, right? We can use it well or we can misuse it. There are risks from poorly designed AI systems, particularly ones pursuing wrongly specified objectives.
I actually think we've given algorithms in general, not just AI systems, but algorithms in general. I think we've given them a free pass for far too long. If you think back there was a time when we gave pharmaceuticals a free pass, there was no FDA or other agency regulating medicines, and hundreds of thousands of people were killed and injured by poorly formulated medicines, by fake medicines, you name it. And eventually, over about a century, we developed a regulatory system for medicines that, you know, it's expensive, but most people think it's a good thing that we have it. We are nowhere close to having anything like that for algorithms, even though perhaps to a greater extent than medicines, these algorithms are having a massive effect on billions of people in the world, and I don't think it's reasonable to assume that it's necessarily going to be a good effect. And I think governments now are waking up to this and really struggling to figure out how to regulate while not actually making a mess of things with things that are too restrictive.
Algorithms are having a massive effect on billions of people in the world, and I don't think it's reasonable to assume that it's necessarily going to be a good effect
”So second point, I know we've talked quite a bit about dystopian outcomes, but the upside potential for AI is enormous, right? And going back to Keynes: yes, it really could enable us to live wisely and agreeably and well, free from the struggle for existence that's characterised the whole of human history.
Up to now, we haven't had a choice. You know, we have to get out of bed, otherwise we'll die of starvation. And in the future, we will have a choice. I hope that we don't just choose to stay in bed, but we will have other reasons to get out of bed so that we can actually live rich, interesting, fulfilling lives. And that was something that Keynes thought about and predicted and looked forward to, but isn't going to happen automatically. There's all kinds of dystopian outcomes, even when this golden age comes.
And then the third point is that, whatever the movies tell you, machines becoming conscious and deciding that they hate humans and wanting to kill us, is not really on the cards.
Find all our podcasts here.
Subscribe: Radio Davos; Meet the Leader; Book Club; Agenda Dialogues.
Join the World Economic Forum Podcast Club on Facebook.
Don't miss any update on this topic
Create a free account and access your personalized content collection with our latest publications and analyses.
License and Republishing
World Economic Forum articles may be republished in accordance with the Creative Commons Attribution-NonCommercial-NoDerivatives 4.0 International Public License, and in accordance with our Terms of Use.
The views expressed in this article are those of the author alone and not the World Economic Forum.
Forum Stories newsletter
Bringing you weekly curated insights and analysis on the global issues that matter.
More on Emerging TechnologiesSee all
Noor Ali Alkhulaif
June 6, 2025
Apoorve Dubey
June 4, 2025
Laura Henderson
June 4, 2025