3 ways generative AI is reshaping the patient experience
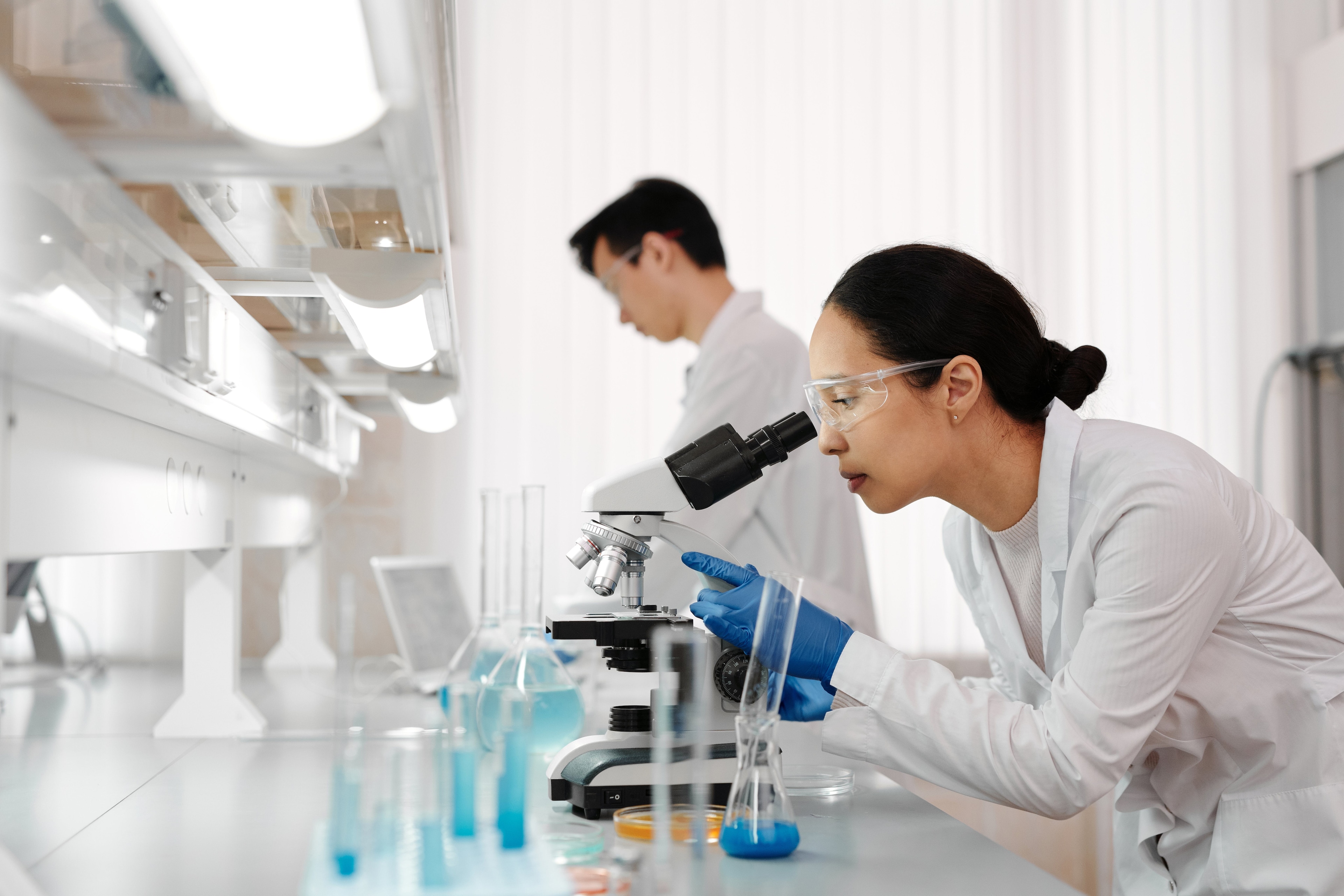
Mistrust is preventing the use of AI in healthcare.
Image: Pexels/Edward Jenner
Shyam Bishen
Head, Centre for Health and Healthcare; Member of the Executive Committee, World Economic ForumStay up to date:
Health and Healthcare
- Generative artificial intelligence (AI) holds significant promise to help healthcare consumers across their health journeys – from providing reliable health information and ensuring appropriate care levels to designing interventions for patient treatment.
- The biggest barriers to adopting generative AI in healthcare are mistrust among doctors and the public, holes in baseline data and scalability in low-resource environments.
- Fostering the adoption of generative AI in healthcare depends on keeping models relevant with the right knowledge, human empathy and data and monitoring bias while addressing cost barriers.
Since at least the 1970s, artificial intelligence (AI) has been hailed as the solution to some of healthcare’s most intractable problems. So far, the reality has fallen short of the hype.
Generative AI, specifically large language models such as those underpinning ChatGPT, offers a powerful new modality to improve global health and healthcare. The World Economic Forum and ZS interviewed leaders spanning healthcare, technology, academia and beyond to assess generative AI’s transformational potential in healthcare, the most significant adoption barriers and actions stakeholders must take to overcome them.
The findings are the subject of the Forum and ZS joint white paper Patient-First Health With Generative AI: Reshaping the Care Experience (January 2024), which sheds light on the relevant timing of generative AI solutions, their potential and what needs to happen to see generative AI in healthcare deployed successfully.
Generative AI for patient care – why now
While generative AI is already being used to discover new drug candidates, revolutionize clinical trials and more, its biggest near-term opportunity may be helping to reimagine patient experience.
The COVID-19 pandemic exacerbated existing healthcare ills, including skyrocketing doctor burnout and increasing financial strain on global health systems. The severe shortage of healthcare workers – the World Health Organization estimates the shortfall at 15 million today, roughly 95% of which is in low- and middle-income countries – poses the greatest risk to global health as uneven access to treatment widens health disparities and adds to the growing burden of care from treating patients with chronic conditions.
Meanwhile, the consumerization of health continues to catalyze healthcare transformation as patients increasingly seek a more active role in managing their health. But this phenomenon poses risks as patients with low health literacy can fall prey to inaccurate or misleading health information online, and increasing use of digital health tools to track and manage health overwhelms doctors’ ability to use new data streams.
Generative AI – with its ability to mimic human speech, process unstructured data (which makes up 80% of all medical information) and solve a wide variety of problems with minimal training – can tackle these challenges by communicating directly with healthcare consumers, thereby shoring up the troubling gap in healthcare workers.
How generative AI will revolutionize patient care
Health education assistance
In a survey conducted before ChatGPT became widespread, 94% of US healthcare consumers said they go online for health information. But studies consistently have found that few health websites meet established quality thresholds and that most US healthcare consumers have limited health literacy skills. Large language models trained on high-quality health data in all languages can offer health information that’s correct and accessible, and therefore actionable, for users regardless of their location, education level or cultural context.
Patient triage co-pilots
Given the healthcare worker shortage, health systems need help shifting resources away from non-urgent patients to those who require timely, high-touch care. Early “virtual care assistants” have failed to scale due to a lack of open-source models for the more than 7,000 languages spoken globally and predictive AI’s limited grasp of nuances across cultural contexts and health system archetypes. With specific and culturally diverse training, large language models can help healthcare providers safely stratify patients entering the healthcare system and route their care accordingly.
Disease management
In their first year of treatment, up to 60% of chronically ill patients miss doses, take the wrong dosage or abandon treatment altogether, costing health systems hundreds of billions of dollars per year. While traditional algorithms can predict when a patient is likely to drop off treatment, they struggle to suggest (let alone execute) effective interventions to keep patients on track. Pairing classical AI with generative AI can create a powerful tool to manage chronic illness by keeping patients on therapy.
The risk of medical or ethical malpractice stemming from the responsible use of generative AI pales compared with the benefit of ignoring this modality and its boundless potential for patient impact.
”The need for strong multilateral collaboration
As with classical AI, mistrust is the most formidable barrier to adopting generative AI in healthcare. For reasons that are frequently unclear, large language models occasionally generate incorrect or misleading answers. Meanwhile, insufficient or biased training data can produce dangerously biased outputs, and it’s inherently risky to feed private health data into an AI model.
At the same time, large language models are currently very expensive to train, fine-tune and run, potentially putting them out of reach for many health systems in low- and middle-income countries. If we’re not careful, generative AI risks widening existing health disparities as those in high-income countries get the spoils.
These barriers aren’t insurmountable. There are four steps stakeholders can take to ensure everyone benefits from advances in generative AI.
- Build trust through empathy and domain expertise. We must prioritize fine-tuning models on healthcare-specific data and having doctors test and rate responses to improve outputs and instil models with empathy.
- Mitigate against bias. It’s impossible to correct for bias inherent in models’ training data fully, so mitigating output bias requires connecting the data ecosystem and then measuring response bias on an ongoing basis – especially when used in underserved populations.
- Keep humans in the loop. We must first educate patients and providers on generative AI’s limitations, especially its potential for hallucination (when the large language model perceives something that doesn’t exist). Additionally, healthcare providers must implement clinical processes that maintain human oversight, especially in high-risk discussions and with patients with severe disease.
- Plan to scale across contexts. Given resource constraints, developing more cost-efficient means to run large language models will be critical. Stakeholders must also work to create flexible deployment models that recognize varying needs across regions, cultures and contexts.
Accept our marketing cookies to access this content.
These cookies are currently disabled in your browser.
A person’s health is inherently precious. Any stakeholder developing, deploying or vouching for a tool that could put even one patient’s health at risk should be cautious. However, the risk of medical or ethical malpractice stemming from the responsible use of generative AI pales compared with the benefit of ignoring this modality and its boundless potential for patient impact.
Generative AI could be the most profound technological breakthrough in our lifetimes. However, society must make a concerted effort to put appropriate protections in place to ensure its safe and responsible use. We have the tools to do so, but now we need to summon the collective will.
Don't miss any update on this topic
Create a free account and access your personalized content collection with our latest publications and analyses.
License and Republishing
World Economic Forum articles may be republished in accordance with the Creative Commons Attribution-NonCommercial-NoDerivatives 4.0 International Public License, and in accordance with our Terms of Use.
The views expressed in this article are those of the author alone and not the World Economic Forum.
Forum Stories newsletter
Bringing you weekly curated insights and analysis on the global issues that matter.
More on Health and Healthcare SystemsSee all
Sofiat Makanjuola-Akinola and Dr Zainab Shinkafi-Bagudu
May 19, 2025
Shyam Bishen and Lorna Friedman
May 19, 2025
Shyam Bishen
May 19, 2025