Research shows AI is often biased. Here's how to make algorithms work for all of us
People walk past a poster advertising facial recognition software at a technology exhibition.
Image: Reuters/Thomas Peter
Stay up to date:
Data Science
Listen to the article
- Existing human bias is too often transferred to artificial intelligence.
- Here are five types of bias and how to address them.
Can you imagine a just and equitable world where everyone, regardless of age, gender or class, has access to excellent healthcare, nutritious food and other basic human needs? Are data-driven technologies such as artificial intelligence and data science capable of achieving this – or will the bias that already drives real-world outcomes eventually overtake the digital world, too?
Bias represents injustice against a person or a group. A lot of existing human bias can be transferred to machines because technologies are not neutral; they are only as good, or bad, as the people who develop them. To explain how bias can lead to prejudices, injustices and inequality in corporate organizations around the world, I will highlight two real-world examples where bias in artificial intelligence was identified and the ethical risk mitigated.
In 2014, a team of software engineers at Amazon were building a program to review the resumes of job applicants. Unfortunately, in 2015 they realized that the system discriminated against women for technical roles. Amazon recruiters did not use the software to evaluate candidates because of these discrimination and fairness issues. Meanwhile in 2019, San Francisco legislators voted against the use of facial recognition, believing they were prone to errors when used on people with dark skin or women.
The National Institute of Standards and Technology (NIST) conducted research that evaluated facial-recognition algorithms from around 100 developers from 189 organizations, including Toshiba, Intel and Microsoft. Speaking about the alarming conclusions, one of the authors, Patrick Grother, says: "While it is usually incorrect to make statements across algorithms, we found empirical evidence for the existence of demographic differentials in the majority of the algorithms we studied.”
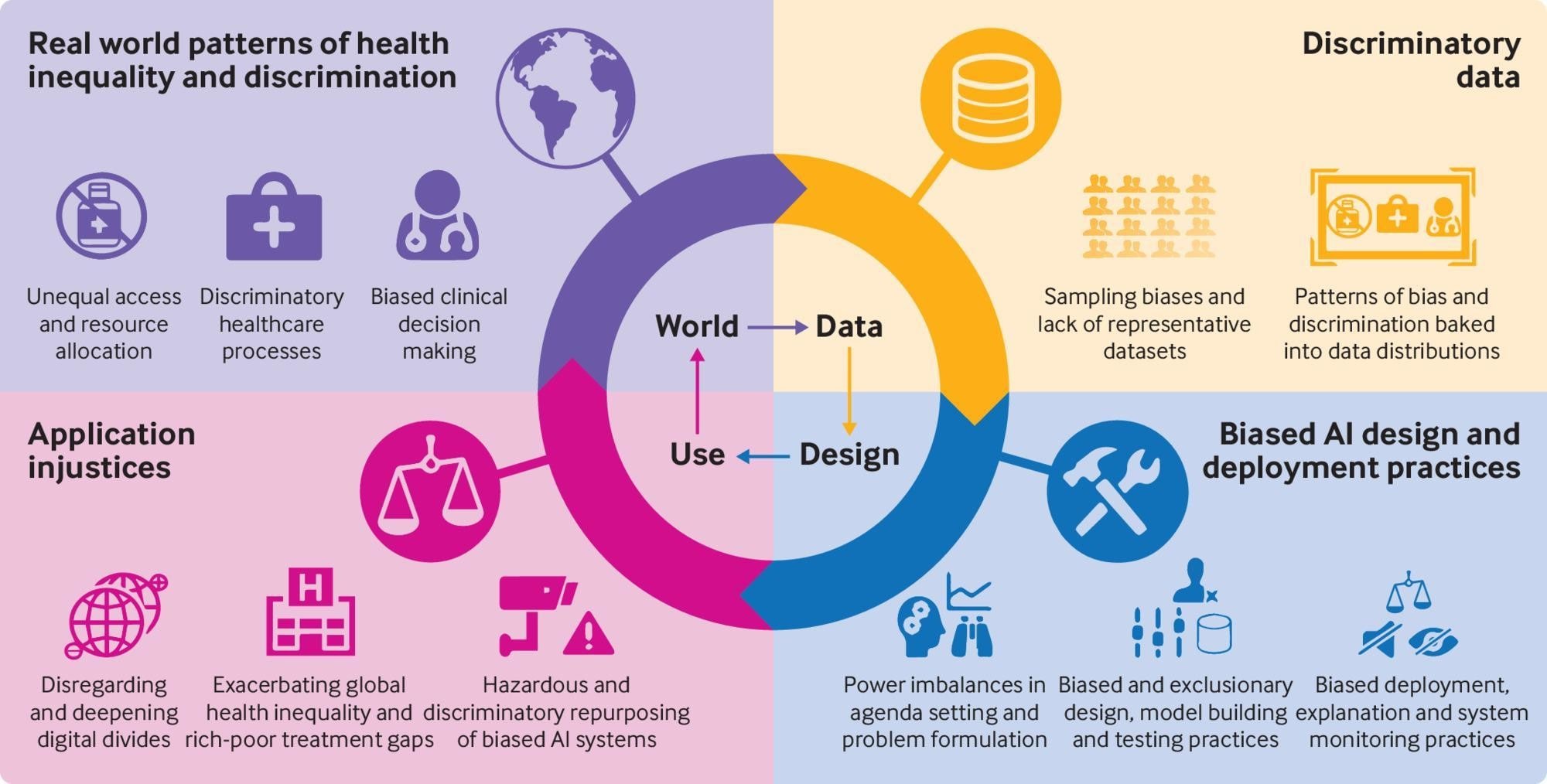
To list some of the source of fairness and non-discrimination risks in the use of artificial intelligence, these include: implicit bias, sampling bias, temporal bias, over-fitting to training data, and edge cases and outliers.
Implicit bias
Implicit bias is discrimination or prejudice against a person or group that is unconscious to the person with the bias. It is dangerous because the person is unaware of the bias – whether it be on grounds of gender, race, disability, sexuality or class.
Sampling bias
This is a statistical problem where random data selected from the population do not reflect the distribution of the population. The sample data may be skewed towards some subset of the group.
Temporal bias
This is based on our perception of time. We can build a machine-learning model that works well at this time, but fails in the future because we didn't factor in possible future changes when building the model.
Over-fitting to training data
This happens when the AI model can accurately predict values from the training dataset but cannot predict new data accurately. The model adheres too much to the training dataset and does not generalize to a larger population.
Edge cases and outliers
These are data outside the boundaries of the training dataset. Outliers are data points outside the normal distribution of the data. Errors and noise are classified as edge cases: Errors are missing or incorrect values in the dataset; noise is data that negatively impacts on the machine learning process.
How to identify fairness and non-discrimination risks
Analytical techniques
Analytical techniques require meticulous assessment of the training data for sampling bias and unequal representations of groups in the training data. You can investigate the source and characteristics of the dataset. Check the data for balance. For instance, is one gender or race represented more than the other? Is the size of the data large enough for training? Are some groups ignored?
A recent study on mortgage loans revealed that the predictive models used for granting or rejecting loans are not accurate for minorities. Scott Nelson, a researcher at the University of Chicago, and Laura Blattner, a Stanford University economist, found out that the reason for the variance between mortgage approval for majority and minority group is because low-income and minority groups have less data documented in their credit histories. Without strong analytical study of the data, the cause of the bias will be undetected and unknown.
Test the model in different environments
What if the environment you trained the data is not suitable for a wider population? Expose your model to varying environments and contexts for new insights. You want to be sure that your model can generalize to a wider set of scenarios.
A review of a healthcare-based risk prediction algorithm that was used on about 200 million American citizens showed racial bias. The algorithm predicts patients that should be given extra medical care. It was found out that the system favoured white patients over black patients. The problem with the algorithm’s development is that it wasn't properly tested with all major races before deployment.
Strategies for mitigating fairness and non-discrimination risks
Inclusive design and foreseeability
Inclusive design emphasizes inclusion in the design process. The AI product should be designed with consideration for diverse groups such as gender, race, class, and culture. Foreseeability is about predicting the impact the AI system will have right now and over time.
Recent research published by the Journal of the American Medical Association (JAMA) reviewed more than 70 academic publications based on the diagnostic prowess of doctors against digital doppelgangers across several areas of clinical medicine. A lot of the data used in training the algorithms came from only three states: Massachusetts, California and New York. Will the algorithm generalize well to a wider population?
A lot of researchers are worried about algorithms for skin-cancer detection. Most of them do not perform well in detecting skin cancer for darker skin because they were trained primarily on light-skinned individuals. The developers of the skin-cancer detection model didn't apply principles of inclusive design in the development of their models.
Perform user testing
Testing is an important part of building a new product or service. User testing in this case refers to getting representatives from the diverse groups that will be using your AI product to test it before it is released.
STEEPV analysis
This is a method of performing strategic analysis of external environments. It is an acronym for social (i.e. societal attitudes, culture and demographics), technological, economic (ie interest, growth and inflation rate), environmental, political and values. Performing a STEEPV analysis will help you detect fairness and non-discrimination risks in practice.
What's the World Economic Forum doing about diversity, equity and inclusion?
It is very easy for the existing bias in our society to be transferred to algorithms. We see discrimination against race and gender easily perpetrated in machine learning. There is an urgent need for corporate organizations to be more proactive in ensuring fairness and non-discrimination as they leverage AI to improve productivity and performance. One possible solution is by having an AI ethicist in your development team to detect and mitigate ethical risks early in your project before investing lots of time and money.
Don't miss any update on this topic
Create a free account and access your personalized content collection with our latest publications and analyses.
License and Republishing
World Economic Forum articles may be republished in accordance with the Creative Commons Attribution-NonCommercial-NoDerivatives 4.0 International Public License, and in accordance with our Terms of Use.
The views expressed in this article are those of the author alone and not the World Economic Forum.
Forum Stories newsletter
Bringing you weekly curated insights and analysis on the global issues that matter.
More on Emerging TechnologiesSee all
Markus Kirchschlager and Benedikt Gieger
July 10, 2025
Victoria Masterson, Ian Shine and Madeleine North
July 10, 2025
Gayle Markovitz and Beatrice Di Caro
July 9, 2025
Ayed Abdulhadi Al-Ruwaili
July 8, 2025
Linda Lacina and Kate Whiting
July 8, 2025
Benedict Singleton and Marta Ferreira de Sá
July 8, 2025